AI adoption at the atomic level of jobs and work
O'Reilly Radar Podcast: David Beyer on AI adoption challenges, the complexities of getting an AI ROI, and the dangers of hype.
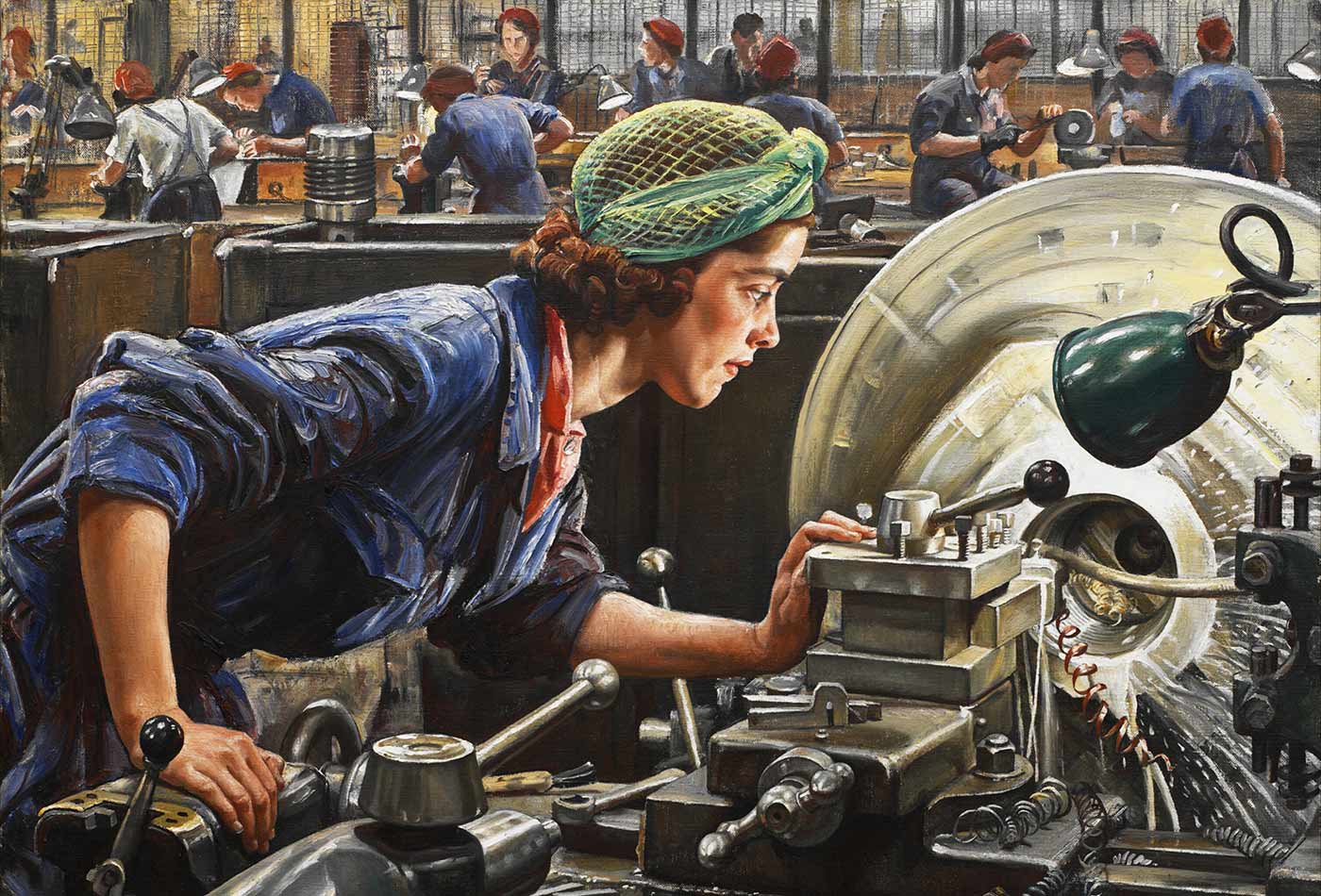
This week, I sit down with David Beyer, an investor with Amplify Partners. We talk about machine learning and artificial intelligence, the challenges he’s seeing in AI adoption, and what he thinks is missing from the AI conversation.
Here are a few highlights:
Complexities of AI adoption
AI adoption is actually a multifaceted question. It’s something that touches on policy at the government level. It touches on labor markets and questions around equity and fairness. It touches on broad commercial questions around industries and how they evolve over time. There’s many, many ways to address this. I think a good way to think about AI adoption at the broader, more abstract level of sectors or categories is to actually zoom down a bit and look at what it is actually replacing.
The way to do that is to think at the atomic level of jobs and work. What is work? People have been talking about questions of productivity and efficiency for quite some time, but a good way to think of it from the lens of the computer or machine learning is to divide work into four categories. It’s a two-by-two matrix of cognitive and manual, cognitive versus manual work, and routine versus non-routine work. The 90s internet and computer revolution, for the most part, tackled the routine work—Spreadsheets and word processing, things that could be specified by an explicit set of instructions.
The more interesting stuff that’s happening now, and that should be happening over the next decade, is how does software start to impact non-routine, both cognitive and manual, work? Cognitive work is tricky. It can be divided into two categories: things that are analytical (so, math and science and the like) and things that are more interpersonal and social—sales, being a good example.
Then with non-routine work, the first instinct is to think about whether the job seems simple to us as people—so, cleaning a room for us, at first blush, seems like something pretty much anyone who’s able could do; it’s actually incredibly difficult. There’s this bizarre, unexpected result that the hard problems are easier to automate, things like logic. The easier problems are incredibly hard to automate—things that require visuospatial orientation, navigating complex and potentially changing terrain. Things that we have basically been programmed over millennia in our brains to accomplish are actually very difficult to do from the perspective of coding a set of instructions into a computer.
AI ROI
The question I have in my mind is: in the 90s and 2000s, was simply applying computers to business and communication its own revolution? Does machine learning and AI constitute a new category or is machine learning the final complement to extract the productivity out of that initial Silicon revolution, so to speak? There’s this economic historian Paul David, out of Oxford, who wrote an interesting thing looking at American factories and how they adapted to electrification because, previously, a lot of them were steam powered. The initial adoption was really with a lack of imagination: they used motors where steam used to be and hadn’t really redesigned anything. They didn’t really get much of any productivity.
It was only when that crop of old managers was replaced with new managers that people fully redesigned the factory to what we now recognize as the modern factory. The question is the technology itself: from our perspective as investors, it’s insufficient. You need business process and workplace rethinking. An area of research, as it relates to this model of AI adoption, is how reconstructible is it—is there an index to describe how particular industries or particular workflows or businesses can be remodeled to use machine learning with more leverage?
I think that speaks to how those managers in those instances are going to look at ROI. If the payback period for a particular investment is uncertain or really long, we’re less likely to adopt it, which is why you’re seeing a lot of pickup of robots in factories. You can specify and drive the ROI; the payback period for that is coming down because it’s incredibly clear, well-defined. Another industry is, for example, using machine learning in a legal setting for a law firm. There are parts of it—for example, technology assisted review—where the ROI’s pretty clear. You can measure it in time saved. Other technologies that help assist in prediction or judgment for, say, higher-level thinking, the return on that is pretty unclear. A lot of the interesting technologies coming out these days—from, in particular, deep learning—enable things that operate at a higher level than we’re used to. At the same time, though, they’re building products around that that do relatively high-level things that are hard to quantify. The productivity gains from that are not necessarily clear.
The dangers of AI hype
One thing I’d say, rather than missing from the AI conversation, is something that there’s too much of: I think hype is one of them. Too many businesses now are pitching AI almost as though it’s batteries included. That’s dangerous because it’s going to potentially lead to over-investment in things that overpromise. Then, when they under-deliver, it has a deflationary effect on people’s attitudes toward the space. It almost belittles the problem itself. Not everything requires the latest whiz-bang technology. In fact, the dirty secret of machine learning—and, in a way, venture capital—is so many problems could be solved by just applying simple regression analysis. Yet, very few people, very few industries do the bare minimum.