Ben Lorica on the emergence of intelligent, real-time data applications
The O'Reilly Radar Podcast: Emerging themes in the data space.
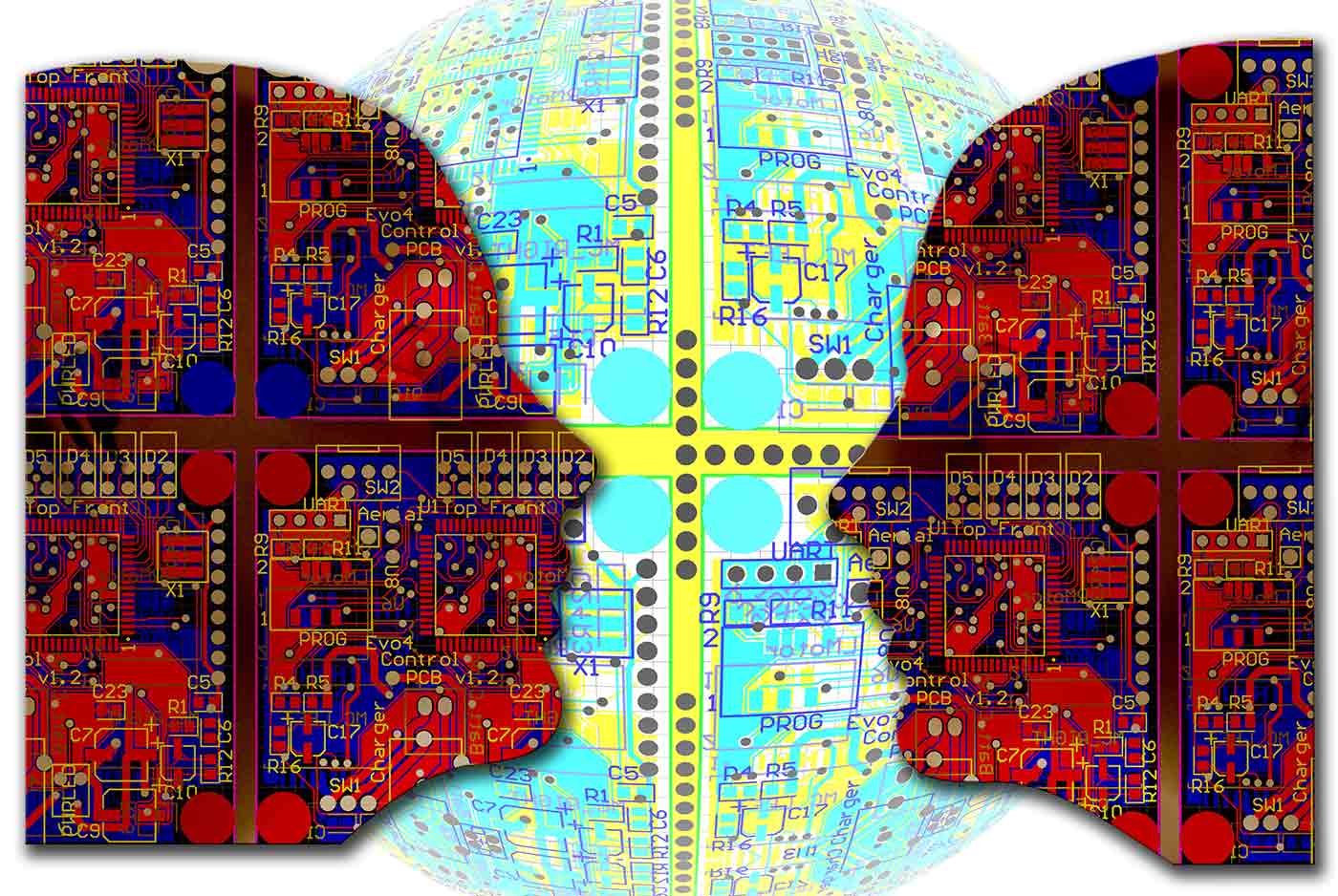
This week, O’Reilly’s Mac Slocum chats with Ben Lorica, O’Reilly’s chief data scientist and host of the O’Reilly Data Show Podcast. Lorica talks about emerging themes in the data space, from machine learning to deep learning to artificial intelligence, and how those technologies relate to one another and how they’re fueling real-time data applications. Lorica also talks about how the concept of a data center is evolving, the importance of open source big data components, and the rise in interest of big data ethics.
Here are a few highlights:
Human-in-the-loop recommendations
Stitch Fix is a company that I like to talk about. They use machine learning recommendations. This is a company that basically recommends clothing and fashion apparel to women. They use machine learning to generate a series of recommendations but then, human fashion experts actually take those recommendations and filter them further. In many ways it’s the true example of augmentation. That humans are always in the loop of the decision making process.
Deep learning inspiration
The developments in deep learning have inspired ideas from other parts of machine learning as well. … People have realized it’s really a sequence of steps in the pipeline, and in each step, you get better and better representation of your data culminating in some kind of predictive task. I think people have realized that if they can automate some of these machine learning pipelines in the way that deep learning does, then they can provide alternative approaches. In fact, one of things that has happened a lot recently is that people will use deep learning particularly for the feature engineering, the feature representation step in the machine learning task and then apply another algorithm in the end to do an actual prediction.
There’s a group out of UC Berkeley, AmpLab, that produced Apache Spark. They recently built a machine learning pipeline on top of Spark. Some of the examples that they ship with are pipelines that you normally associate with deep learning, such as images and speech index. They built a series of primitives that you can understand—you can understand how each of these primitive components works—and then you just piece them together in the pipeline. Then they optimize the pipeline for you, so in many ways, they mimic what a deep learning architecture does, but maybe they provide more transparency because you know exactly what’s happening in each step of this pipeline.
Learning to build a cake
Deep learning people—and Yann LeCun in particular—have this distinction where, if you think of machine learning as playing a role in AI there are really three parts to it. Unsupervised learning, supervised learning and re-enforcement learning. He talks about unsupervised learning as being the cake, supervised learning being the icing on the cake, and reinforcement learning as being the cherry on the cake. He says the problem is that we don’t know how to build the cake. There’s a lot of unresolved problems in unsupervised learning.
Structuring the unstructured
At the end of the day, I think AI, and machine learning in general, will require the ability to basically do feature extraction intelligence. In technical terms, if you think of machine learning as discovering some kind of functional mapping one space to another—here’s an image, map it into a category—then what you are really talking about is a function that requires variables, so these variables are features. One of the areas I’m excited about is people who are about to take unstructured information like text or images and turn it into structured information. Because once you go from unstructured information to structured information, the structured information can be used as features in machine learning algorithms.
There’s a company that came out of Stanford’s Deep Dive project called Lattice.io. It’s doing interesting things in this area, where they are taking text and imaging and extracting structured information from these unstructured data sources. Basically, human-level accuracy, but, obviously, since they are doing it using computers, they can scale as machines scale. I think this will unlock a lot of data sources that normally people would not use for predictive purposes.
The rise of the mini data center
The other interesting thing I’ve noticed over the past few months is the notion of a data center. What is a data center? Well, a data center is a huge warehouse near a hydroelectric plant, right? It’s the usual notion. But as some of our environments generate more data—think of a self-driving car, or a smart building, or an airplane—once they are generating lots and lots of data, you could consider them mini data centers in many ways, right? Some of these platforms need to look ahead into the future, where they have to be simpler. Simple enough so you can stick a mini data center inside a car. Slim down your big data architecture enough so they can stick it somewhere so you don’t have to rely too much on network communication to do all of your data crunching. I think that’s an interesting concept; there are companies that are already deciding their architectures around this notion that there will be a proliferation of these data centers, so to speak.