Building data lifecycles for IoT projects with edge analytics
The O'Reilly Podcast: Han Yang on the importance of investment, innovation, and improvisation.
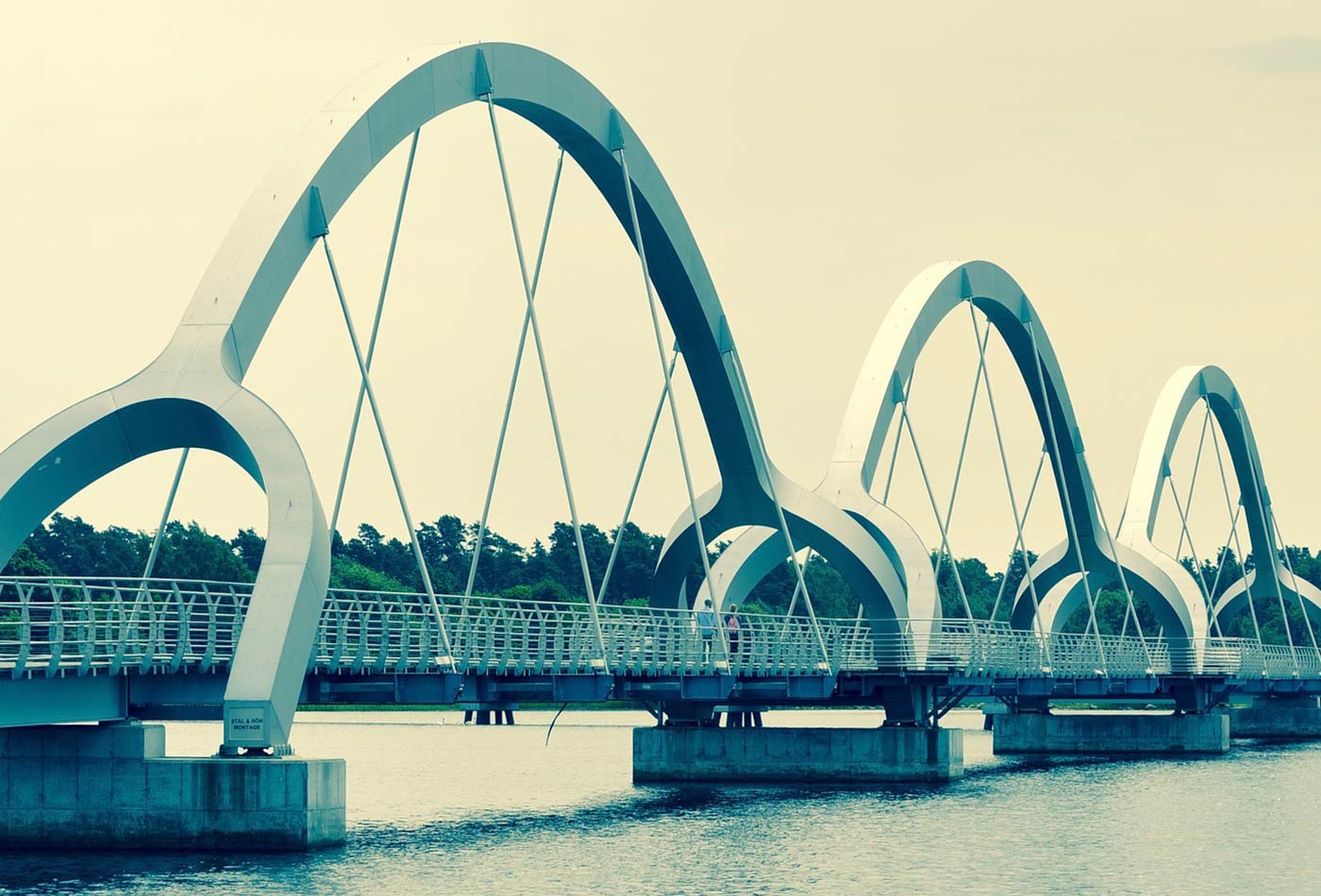
In this episode of the O’Reilly podcast, I speak with Han Yang, senior product manager at Cisco, working on analytics solutions. We discuss the impact of data analytics across industries, building data lifecycles for Internet of Things (IoT) applications, and advice for establishing successful analytics and machine learning projects.
Here are some highlights from our conversation:
Considerations for managing IoT projects
When it comes to building end-to-end analytics platforms for IoT-driven digital transformation, we want to make sure customers are building a data lifecycle from the birth of that data: from the edge to all the way to the data center, getting real-time insights on streaming data, and historical and trend analytics, and raising the abstraction of the information while dealing with the temperature of the data—moving to an active archival tier as it become cold. Another key consideration is, over time, you’re going to be dealing with ever-changing business challenges and making sure that, rather than hard-wiring your algorithms to the low-level data, you’re able to raise that abstraction.
And as with any type of analytics, there’s always a notion of investment. A lot of our customers are anxious to figure out: “What data will we really find?”, “Is it really valuable?”, and “How do we actually make money out of this investment, which seems to have promise, but may not always realize the ROI in a short period of time?”. … As the customer invests, they’re able to grow and evolve.
How the analytics market has evolved
Historically, analytics meant ‘enterprise data warehouse.’ It has changed; over the last 10-plus years, a lot of customers have been doing big data analytics with a scale-out type of architecture—in most cases, using Hadoop and NoSQL databases. Most Hadoop applications are built using MapReduce now, increasingly moving to Apache Spark. NoSQL databases are slowly becoming mainstream, even replacing traditional transaction systems. That’s the new paradigm, where you’re able to handle large amounts of data cost effectively and take advantage of innovations in the open source ecosystem. The next wave will be deeper analytics with technologies like machine learning.
Setting up your analytics project for success
Start small, with a talented team with executive sponsorship. Start small so the team can have very approachable goals and be able to quickly get the low-hanging fruit. Get some early wins. Once that happens, make sure the sponsoring executive provides the freedom for the team explore a little bit, because, at the end of the day, this is a journey of discovery. Nobody knows exactly what the value of the data will be. Let the team have at least some period of time to ponder and to explore what’s in the data—to look for that hidden value, hidden gem. If it were obvious, then everybody would have already discovered it. Giving the team that latitude of freedom is critical.
Finally, given the choice of better algorithms or analytics techniques versus more data, it seems like more data is winning out. Getting more qualified data is the key to successful analytics projects because so many of the newer techniques, such as machine learning, rely on that volume of data to train the models, and to succeed and anticipate what’s going to happen next.
This post is a collaboration between O’Reilly and Cisco. See our statement of editorial independence.