Cancer detection, one slice at a time
New technology is allowing researchers to use digitization to help detect cancer.
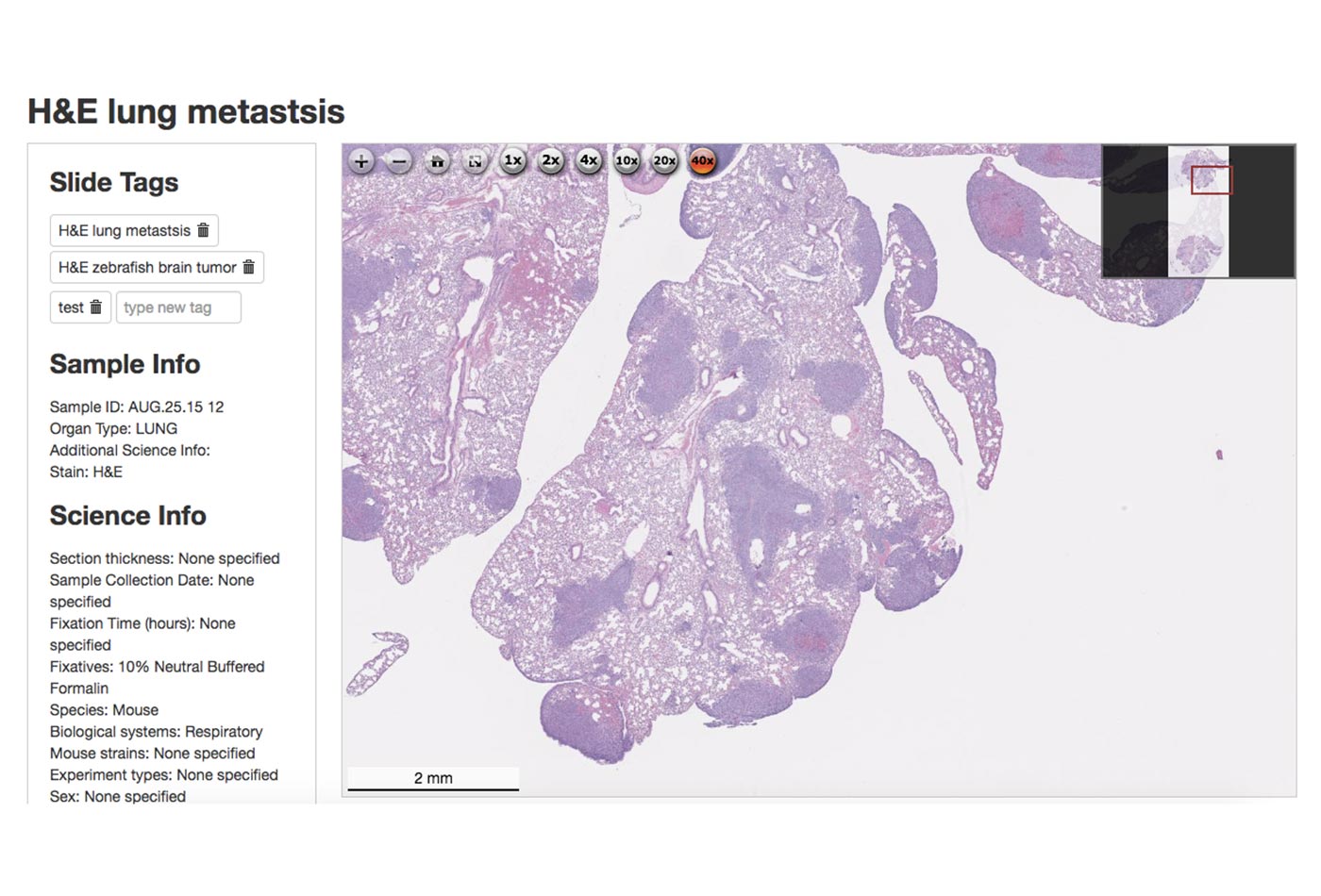
Ke Cheng’s story begins in a way that’s achingly familiar. She recalled late nights and long hours as a grad student, repeating a tedious task in the name of research. Like many of us, Ke dreamed of starting a company that would make that job easier and faster. She ended up building the world’s largest online, preclinical pathology database, which catalyzes and could ultimately lead to the automated detection of cancer. But let’s take a step back—how did Ke go from an overworked grad student to building a fountain of digital data?
For her, the tedious grad-school task was cutting tissue and making slides for histopathology (the analysis of tissue to identify or determine changes due to a disease). The process starts by preserving a tissue sample and embedding it within a block of paraffin wax. A microtome is used to section the tissue into slices thin enough for microscopy (typically, about five microns thick). Each slice is placed on a microscope slide, where it can be stained to color different structures. The most common stain, H&E, is a mixture of an acidic dye (hematoxylin) and a basic one (eosin), which will stain cytoplasm pink and nuclei purple. This alone can be used to detect disease, just by allowing researchers to observe the organization (or disorganization) of the tissue structure. But there are hundreds of “special stains” to detect tissue elements (e.g., muscle fibers, glycoproteins, and mucins), microorganisms (e.g., fungi and bacilli), or specific ions like ferric iron (Fe3+). Immunohistochemistry (IHC) can also be performed on tissue slides. IHC uses antibody-based detection to confirm cancer subtypes. While standard H&E staining is automated, using special stains or IHC can take significant time to optimize. Most researchers prep the tissue themselves and then send it off for sectioning and staining. At the time of Ke’s graduate work, it took two or more weeks to get the slides back.
The key step that transformed Ke’s histology-service company into a pathology data powerhouse was simple: digitization. When Ke founded HistoWiz four years ago, there were already instruments available that would automate histopathology (though they were uncommon to see in everyday research labs). But automating slide histology wasn’t the final vision; it was just the first step. Ke was inspired by the open source genomic research model, in which searchable databases exist that everyone can access and contribute to. She saw firsthand that a trove of histopathology data existed but was unable to be easily shared because it was siloed in slide boxes (and tucked away in dusty cabinets or drawers). Even in the literature, only representative images (tiny sections of the entire slide) were used; and of course, publishable results are a small fraction of the all data collected.
Ke started HistoWz on her own and drew an initial customer base by promising a three-day turnaround (compared to the two-week industry standard at the time). She spent her days going door to door in her old research buildings, asking if people would be interested in doing a free trial. Then she spent her nights processing the samples using core facility equipment. Four years later, HistoWiz supports a number of major research institutions (including Harvard, MD Anderson, and HHMI), as well as big pharma (Pfizer, Regeneron, and Novo Nordisk).
The difference between HistoWiz and the other histology competitors was that Ke was the first to digitize the slides. After placing an order, the customer receives a high-res, microscope-quality image of the entire slide that can be viewed (with up to 40x magnification) on a laptop, smartphone, or tablet (Figure 1-1). If desired, the customer can receive the raw image or have the slide analyzed by one of HistoWiz’s in-house pathologists. While providing faster, better histology as a service, HistoWiz had an open pipeline to data. Each customer receives a discount if they choose to contribute their data, so each slide HistoWiz processed could be incorporated into a database.
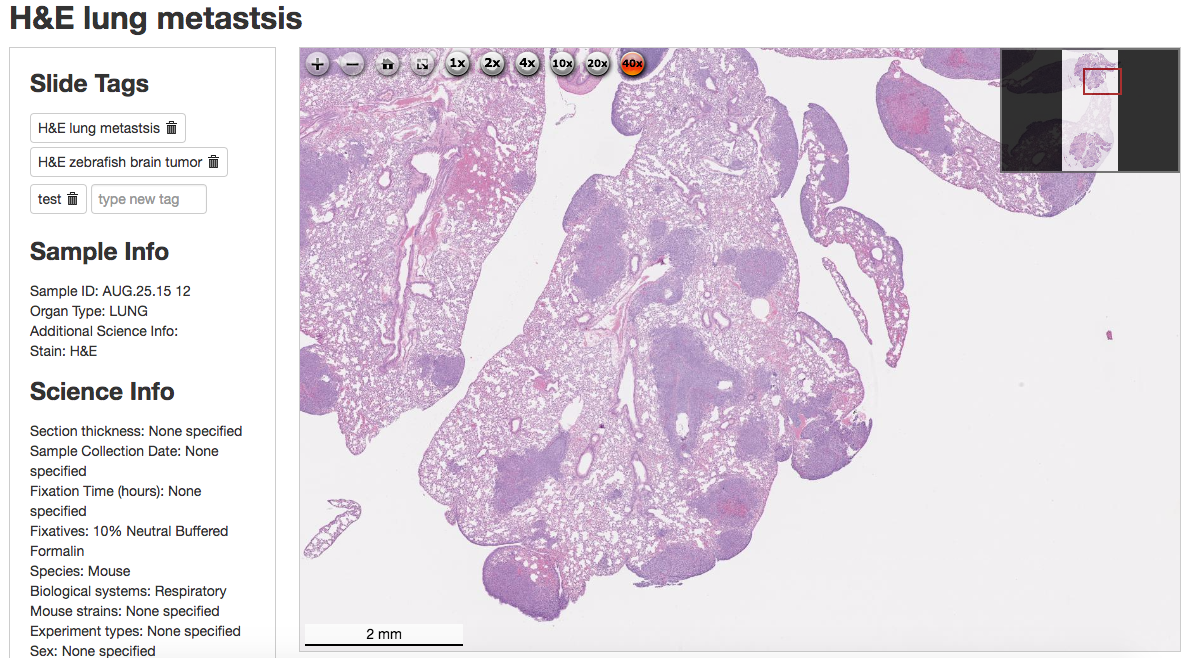
HistoWiz’s PathologyMap now has over 30,000 slides and is growing at a rate of over 200 percent each year. While most of the data is from mouse tissue, PathologyMap has data from human and other preclinical/experimental models (zebrafish, rats, rabbits, etc.) as well. Ke’s mission is to “fight cancer cooperatively.” No more slide boxes or static representative images—this database allows researchers to do tissue-driven data mining by using whole slides in a massive dataset. It’s important to Ke that this data is not restricted to just pathologists or academic researchers. For her, this opens a door to allow anyone to step inside the histology field and access the latest discoveries in cancer research.
The possibilities of what can be done with this database are pretty fascinating. This is a new era of “digital pathology.” The FDA recently approved digital pathology for primary diagnosis, but acquiring enough data is still a very limiting factor. Ke’s database provides the raw data needed to accomplish that goal.
Secondly, Ke sees a potential for researchers to use the HistoWiz database as a tissue bank. She’s collecting extra unstained slides from her contributors, with a vision that someday researchers could request an unstained slide to analyze with their experimental tools instead of having to develop a mouse model themselves. While clinical tissue banks exist, there are few analogous resources for the preclinical research community.
And last but not least, the moonshot. Ke’s team is in the process of developing a machine learning algorithm to automate the diagnosis and prognosis of cancer. The goal is, from histology alone, to be able to predict whether a patient will respond to a certain type of therapy (or be a “nonresponder”). This approach would be cheaper and faster than current methods (genetic sequencing, for example). And this future may be closer than we think! A team at Stanford working on genetics and biomedical informatics recently published an algorithm that can predict non-small cell lung cancer prognosis just from H&E slides (Snyder and Rubin, 2016).
HistoWiz has a number of pathologists on staff to contribute “tagging” (identifying tumor types, tumor margins, etc.) for the database. An online annotation tool is under development so that the data and the analysis can be crowdsourced. Different users will have different levels to help distinguish tags from the in-house pathologists, versus the slide owner, versus the general public. But all in all, the more data, the better to feed the machine learning algorithm. Ke doesn’t expect machine learning to take the place of pathologists for diagnosis any time soon but can see the value of starting with prognosis of preclinical models.
While the potential to automate cancer detection would get anyone up in the morning, Ke finds inspiration from the end goal and the artistic beauty of the data itself. The company posts on Twitter “images of the day,” sometimes with holiday themes (Figure 1-2). If you’d like to get to know Ke or learn more about HistoWiz, sign up to join one of the monthly dinners.
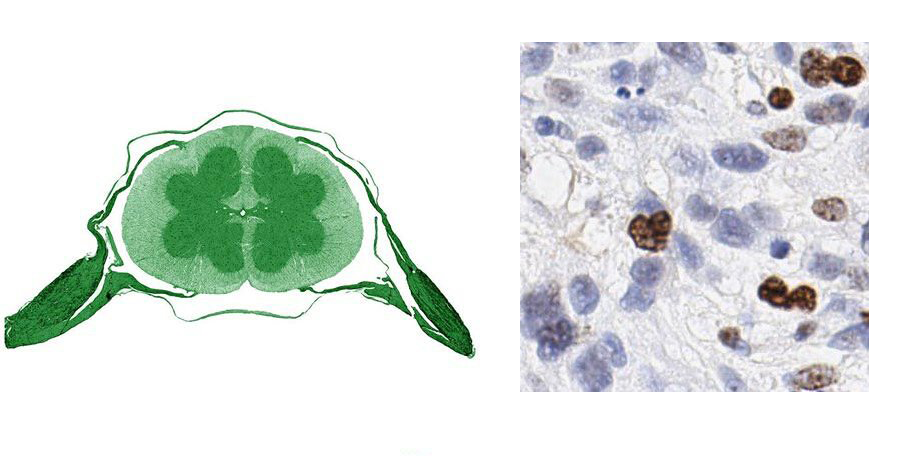