Conquering data preparation at enterprise scale
The O’Reilly Podcast: Nikolaus Bates-Haus on tools and techniques for addressing data variety and augmentation at scale.
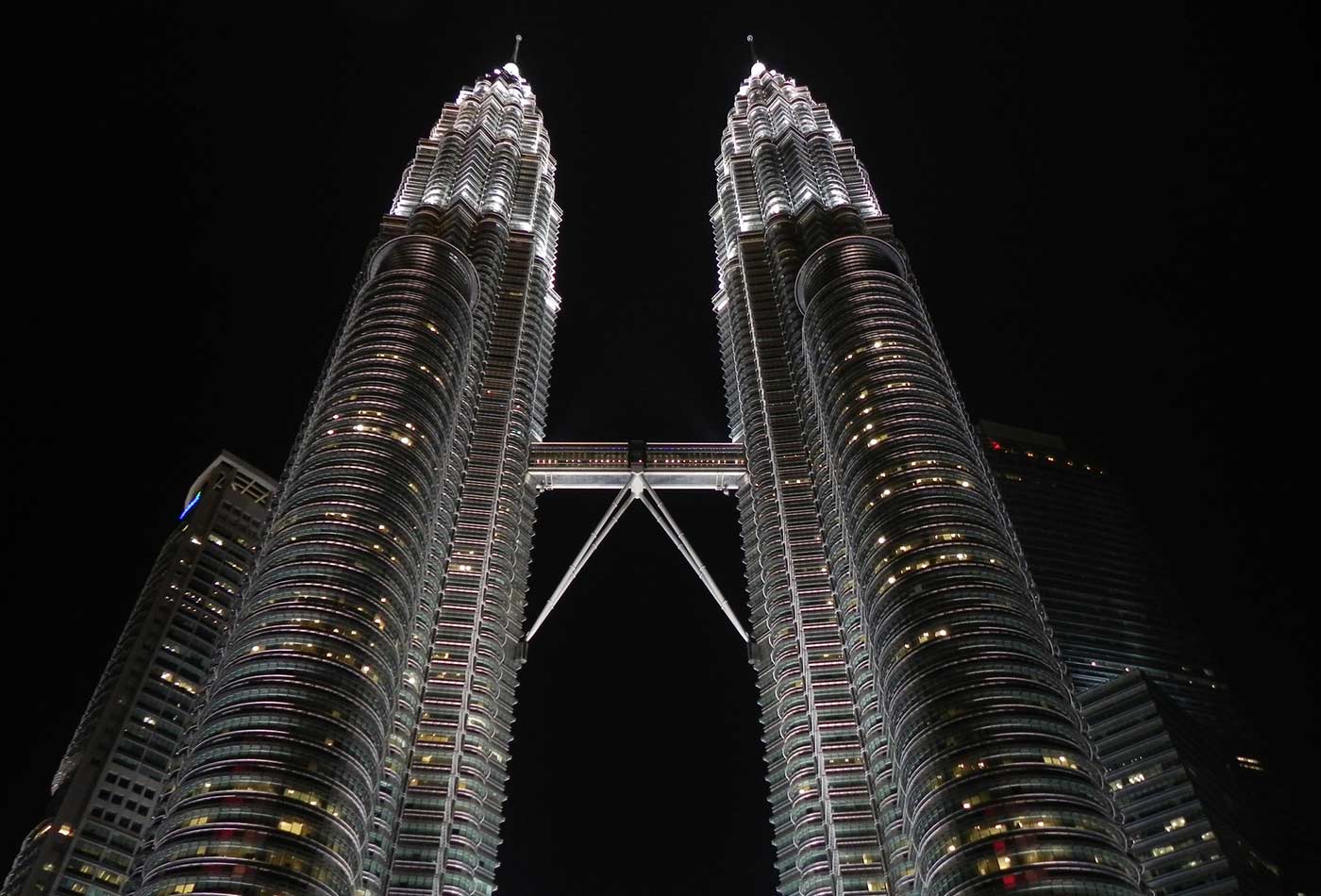
In this episode of the O’Reilly Podcast, O’Reilly’s Ben Lorica sat down with Nikolaus Bates-Haus, technical lead at Tamr. Lorica and Bates-Haus discuss principal dimensions of data preparation, challenges and solutions for data processing at enterprise scale, the value of the data catalog, and how Tamr solutions integrate Apache Spark.
Here are a few highlights from their conversation:
Augmentation, volume, and variety at scale
Domain experts are absolutely critical. Without domain expertise brought to bear on machine learning, the machine learning itself is prone to going off in crazy directions—doing crazy things that make no sense to anyone. The business is only going to trust results where people whom they know and trust have their hands on the data, their hands on the system, have given the system guidance—where those same people have looked at the results that the system has produced and have validated them. That’s an absolutely critical part to any automated system, be it a machine learning system or otherwise.
The social aspect of data integration
At enterprise scale, you run into a lot of challenges around data semantics, data representation, data governance. There’s no one person who understands all of the data, much less where it is and how you might access it.
Data preparation at enterprise scale really means working closely with many groups and helping them to work together in order to achieve a common goal. A big part of that is making sure they do understand the common goal.
A lot of what we had found with these broad data unification, data integration projects is that the effort is as much social and business as it is technological. … It’s not just about data engineering and giving people all of the different functions and functionality that they need in order to manage the data in particular ways. It’s about helping people talk to each other, identify problems, and bring their colleagues into the conversation around those challenges, so they can collaborate and decide on what the right course of action is.
Challenges of operating at enterprise scale
There are a couple of big challenges that are really magnified when you get to large scale. One is data variety. If you’re working with a couple of sources, finding a common representation and a common set of data semantics to bridge those few sources, that is a tractable problem, and you can have a person working on that problem.
When you have hundreds of sources or thousands of sources, finding a common set of data semantics that bridge all of those sources is just not a human-tractable problem. That’s where Tamr’s machine-human collaboration really shines, by having a person describe to the system how to reconcile differences between a few different sources, and have the machine go and apply that broadly across all these different sources.
Another challenge is the velocity of change. … When you get to scale, having a person or a team of people try to keep up with the ever-changing representation and semantics of the data just doesn’t work anymore. You need a system that can adopt automatically to those changes.
This post and podcast is part of a collaboration between O’Reilly and Tamr. See our statement of editorial independence.