Ditch the data dump
How in-page analytics and design thinking produces a rich, functional product.
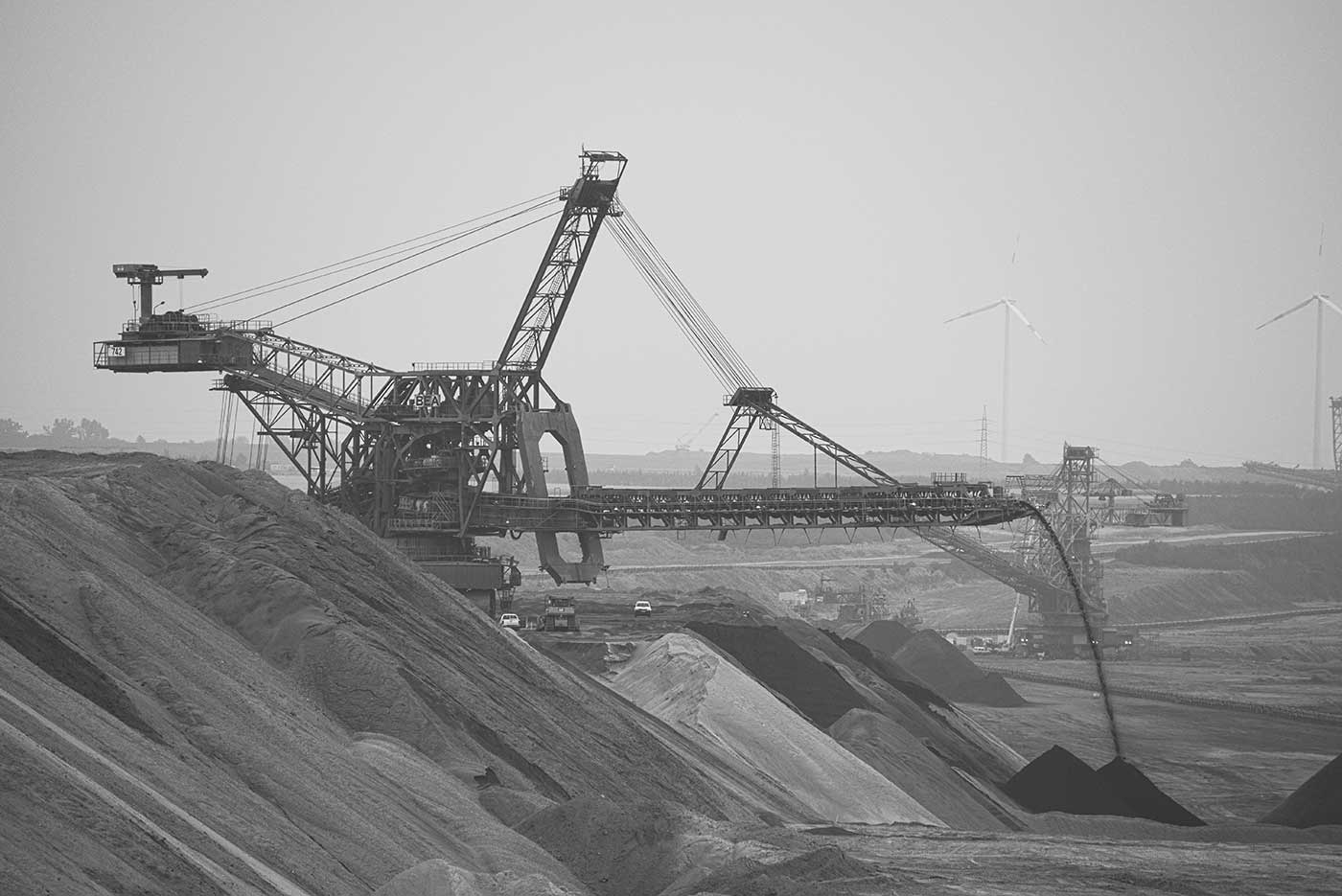
The democratization of data is a shift in data access—it’s intended to liberate the masses so that anyone can make data-driven decisions. But all too often, democratization became synonymous with a data dump, requiring time and data literacy to provide meaningful insight. While the dashboard era (providing analytics in a separate portal or analytics program) assisted in DIY insight, this technique still outsources the process to the customer instead of building analytics into the tools.
New habits in user consumption (specifically, cloud-based and responsive mobile- or tablet-friendly products) have increased users’ expectations. Immediate answers are a must, as is eliminating the need to devote time and focus for a spreadsheet download and data crunch. Users expect intelligent design and intuitive user interfaces to distill complex information into insights.
Good UI/UX leads to action; bad UI/UX leads to distraction
In response to user demands, analytics inside applications are changing. Static reporting and separate reporting portals are no longer meeting users’ needs. Analytics must be natively embedded (often termed “in-page” or “infused”) within the application. In-page analytics let you build an Amazon Dash button for your software, putting the response right next to the analytics that inform your response. Your user sees a particular item is low in stock and, like magic, the reorder button is right there. Otherwise, the user must navigate from the reporting interface to the ordering interface, costing precious time and resources. The only way to achieve “actionable insights” is to tie analytics to native application functionality. Good UI/UX leads to action; bad UI/UX leads to distraction.
The benefits of in-page analytics also affect the bottom line: new sales, increased customer retention, increased win rates with lower costs per lead, and higher costs for advanced functionality. Companies can charge, on average, $526 more per user for a software edition that includes advanced analytics, compared to basic reporting.
Product design must be “analytics-first”
Understandably, core application functionality is at the forefront of the design and development process. Agile development and minimum viable products can shaft analytics to backburner status, yet modern data consumption expectations force us to invert that paradigm. Design thinking is vital to providing the right analytic answers to your users since product value is inherently tied to solving customer problems.
If you are building a new product from scratch that includes analytics, what’s the risk of designing analytics early, based on the needs and behaviors of your users? You would still have had product design workshops, and you would have white-boarded all the possible analytics you can think of. You surely would have picked some/most of the right metrics. But would you have organized the metrics based on varying users (e.g., front-line employees versus operations versus the CMO?) Would you have planned a structure that provides the right flexibility for exploration?
If you are retro-fitting an existing product, we’d argue that providing wrong, inflexible metrics is worse than an iterative alternative. Pick your top three (or even just the top) user persona and build flexible data exploration for the rest. You can release your product or MVP quickly, gather feedback, and assign remaining personas to upcoming releases on the product roadmap.
With a design mindset, focus not only on the answers needed but the consumption model that best fits the user’s needs. Does this user have time to do root cause analysis on a particular issue? If so, they’re likely going to use a laptop and could benefit from ad-hoc functionality. Are they asked to provide quick answers to urgent questions? In that case, provide clear graphics for “at-a-glance” decision-making in a mobile- or tablet-friendly interface.
Design thinking not only guides the right data model, but informs the right UX (laptop versus mobile versus tablet) and the right UI (what actions should the user take in response to this data). Building in-page analytics allows you to achieve this trifecta, where more outdated analytics models (like separate reporting tabs or static reports downloads) fall short.
Find an embedded analytics tool to meet modern product needs
The next step is to identify the best analytics tool to use for embedding. The new free book Embedding Analytics in Modern Applications reviews how to select the right analytics vendor to overcome modern challenges like multitenancy, scalability, and performance, while providing best-in-class analytics for your users.
Download “Embedding Analytics in Modern Applications” to learn more about embedded analytics methods and selecting the right tool to meet the challenges of modern applications.
This post is a collaboration between O’Reilly and TIBCO Jaspersoft. See our statement of editorial independence.