Hilary Mason on the wisdom missing in the AI conversation
The O'Reilly Radar Podcast: Thinking critically about AI, modeling language, and overcoming hurdles.
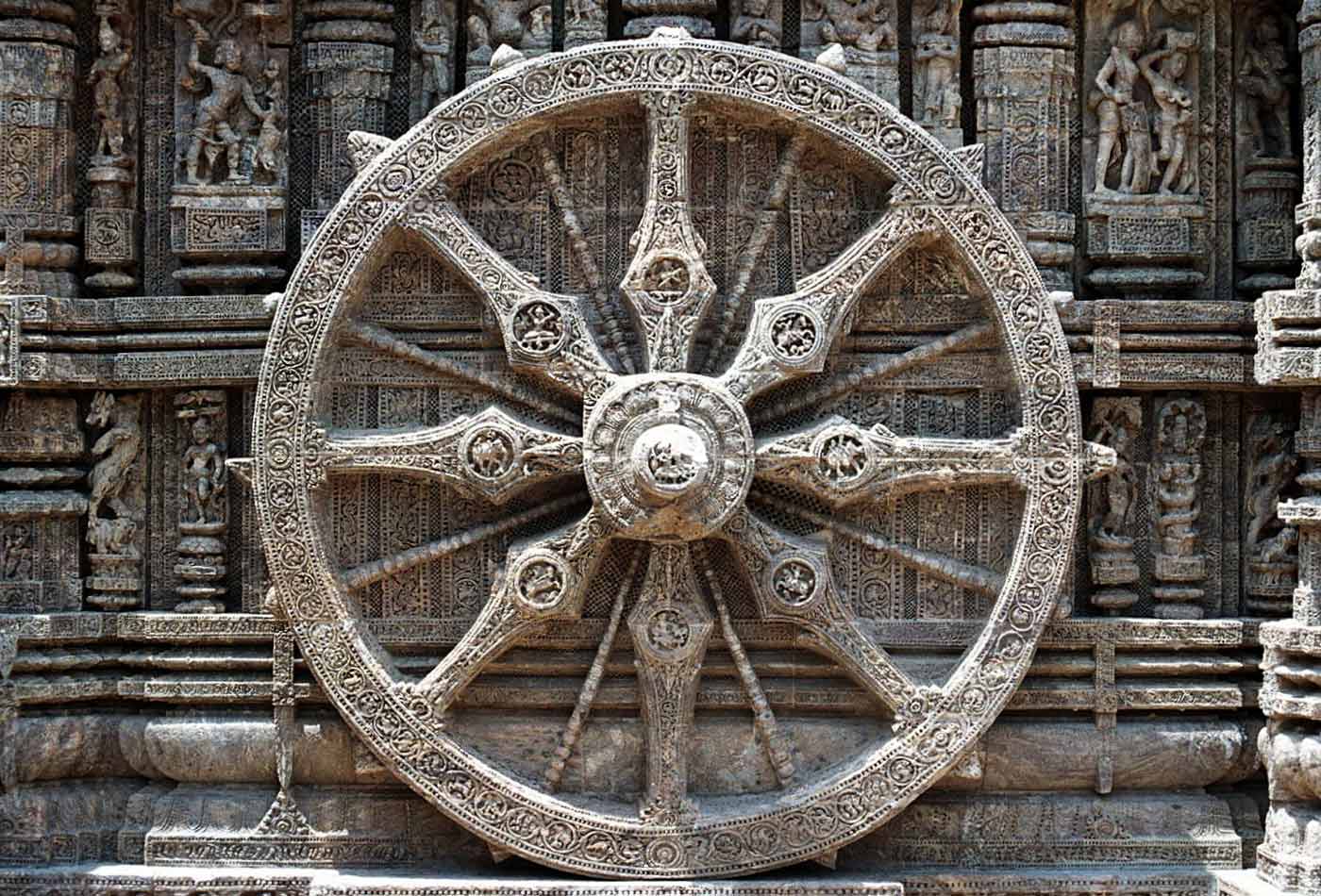
This week, I sit down with Hilary Mason, who is a data scientist in residence at Accel Partners and founder and CEO of Fast Forward Labs. We chat about current research projects at Fast Forward Labs, adoption hurdles companies face with emerging technologies, and the AI technology ecosystem—what’s most intriguing for the short term and what will have the biggest long-term impact.
Here are some highlights:
Missing wisdom
There are a few things missing [from the AI conversation]. I think we tend to focus on the hype and eventual potential without thinking critically about how we get there and what can go wrong along the way. We have a very optimistic conversation, which is something I appreciate. I’m an optimist, and I’m very excited about all of this stuff, but we don’t really have a lot of critical work being done in things like how do we debug these systems, what are the consequences when they go wrong, how do we maintain them over time, and operationalize and monitor their quality and success, and what do we do when these systems infiltrate pieces of our lives where automation may have highly negative consequences. By that, I mean things like medicine or criminal justice. I think there’s a big conversation that is happening, but the wisdom still is missing. We haven’t gotten there yet.
Making the impossible possible
I’m particularly intrigued at the moment by being able to model language. That’s something where I think we can’t yet imagine the ultimate applications of these things, but it starts to make things that previously would have seemed impossible possible, things like automated novel writing, poetry, things that we would like to argue are purely human creative enterprises. It starts to make them seem like something we may one day be able to automate, which I’m personally very excited about.
The impact question is a really good one, and I think it is not one technology that will have that impact. It’s the same reason we’re starting to see all these different AI products pop up. It’s the ensemble of all of the techniques that are falling under this umbrella together that is going to have that kind of impact and enable applications like the Google Photos app, which is my favorite AI product, or self-driving cars or things like Amazon’s Alexa, but actually smarter. That’s a collection of different techniques.
Making sentences and languages computable
We’ve done a project in automated summarization that I’m very excited about—that is applying neural networks to text, where you can put in a single article and it will extract; this is extractive summarization. It extracts sentences from that article that, combined together, contain the same information in the article as a whole.
We also have another formulation of the problem, which is multi-document summarization, where we apply this to Amazon product reviews. You can put in 5,000 reviews, and it will tell you these reviews tend to cluster in these 10 ways, and for each cluster, here’s the summary of that cluster review. It gives you the capability to read or understand thousands of documents very quickly. … I think we’re going to see a ton of really interesting things built on the techniques that underlie that. It’s not just summarization, but it’s making sentences and languages computable.
Adoption hurdles
I think the biggest adoption hurdle [for emerging technologies]—there are two that I’ll say. The one is that sometimes these technologies get used because they’re cool, not because they’re useful. If you build something that’s not useful, people don’t want to use it. That can be a struggle.
The second thing is that people are generally resistant to change. When you’re in an organization and you’re trying to advocate for the use of a new technology to make the organization more efficient, you will likely run into friction. In those situations, it’s a matter of time and making the people who are most resistant look good.