Integrating data with AI
Tamr’s Eliot Knudsen on algorithms that work alongside human experts.
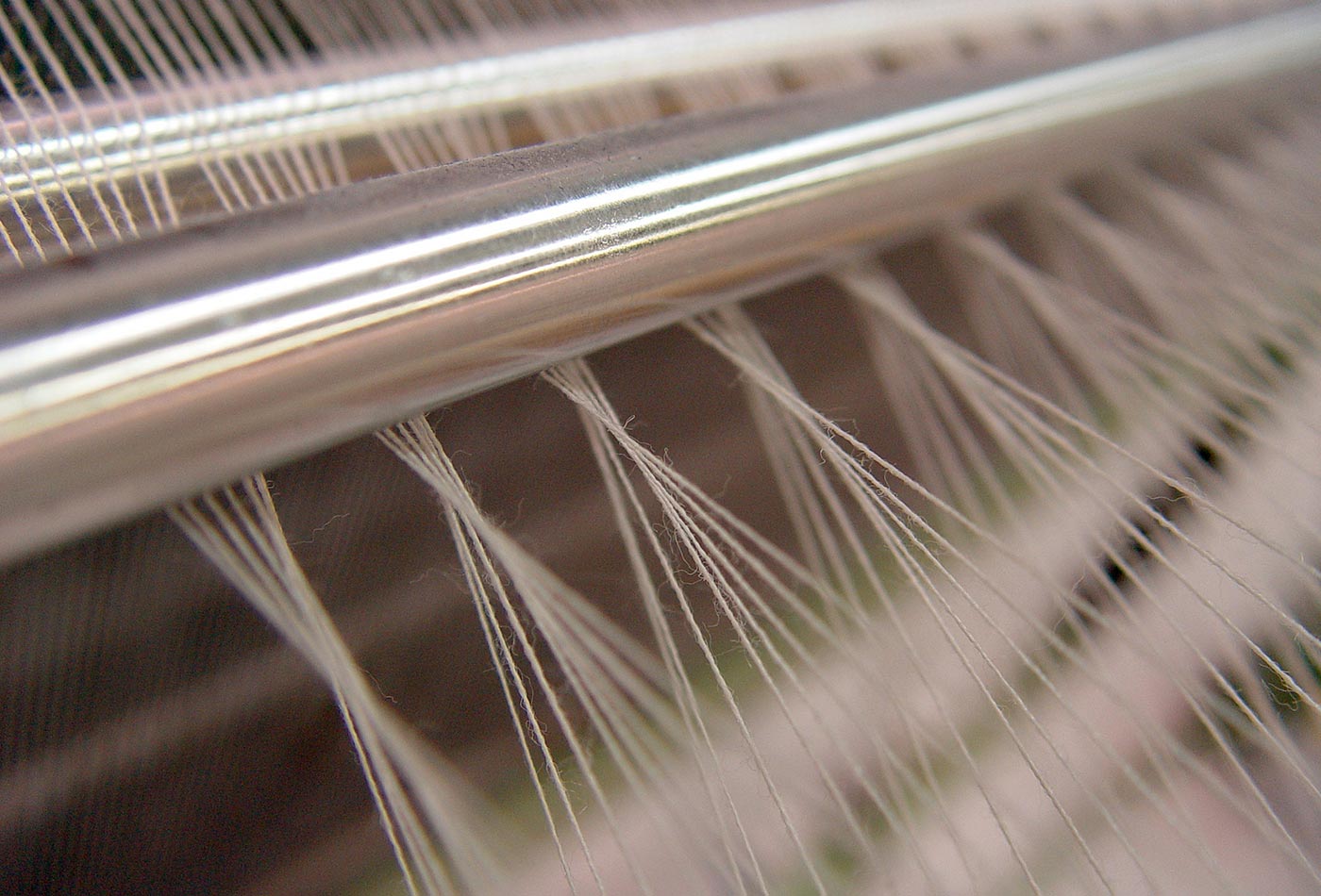
As companies have embraced the idea of data-driven management, many have discovered that their hard-won stores of valuable data are badly siloed: separate troves of data live in different parts of the company on separate systems. These data sets may relate to each other in essence, but they’re often difficult to integrate because they differ slightly in schemas and data definitions.
In this podcast episode, I speak with Eliot Knudsen, data science lead at Tamr, a company that uses AI to integrate data across silos. Data integration is often a painstaking, highly manual process of matching fields and resolving entities, but new tools can work alongside human experts to discover patterns in data and make recommendations for automatically merging it.
The participation of humans in the process is essential. Knudsen points to Andrew Ng’s assessment that “if a typical person can do a mental task with less than two seconds of thought, we can probably automate it using AI either now or in the near future.” AI-driven products that make more complex judgments need to acknowledge their limitations and invite humans into the loop.
Knudsen cites a Gmail feature as an example: the service launched in 2004 with a sophisticated spam filter that was able to work with a high degree of accuracy by training its model across all of Gmail’s user accounts. A few years later, Gmail introduced another AI-driven feature that’s considerably more complex: a flag that indicates whether you’re likely to think a message is important. Knudsen emphasizes the fact that this feature is only a flag, not a filter like the spam detector.
“On the surface, the idea of flagging email as being spam versus not spam, and important versus not important looks very similar,” he says. “But if you think about what it takes for someone to figure out whether or not an email is actually important and actually deserving of their attention, that’s usually something that—at least for me—takes longer than two seconds. I need to actually go through, I need to think about whether this is an email that I should be responding to at all. It’s unlikely that artificial intelligence is in any near term going to be able to say that an email is important versus not important with a high degree of accuracy.”
So, says Knudsen, Google’s response to that challenge shows the “important flag” as a recommendation that, unlike a spam filter and more similar to a notification, fits better with the user experience.
In the same way, the process of data integration needs to draw on the expertise of human users—whether technical or business managers—who often have deep insight into the ways that their data sets are structured and used. By using AI-driven tools, managers can get the best possible leverage out of the time that humans need to put into the integration process—what Knudsen calls “the machine-driven, human-guided approach.”
This post is a collaboration between O’Reilly and Tamr. See our statement of editorial independence.