Jupyter Insights: Tim Gasper, director of product and marketing at Bitfusion
Getting started with data science, Jupyter as shareable hub, and JupyterLab adoption.
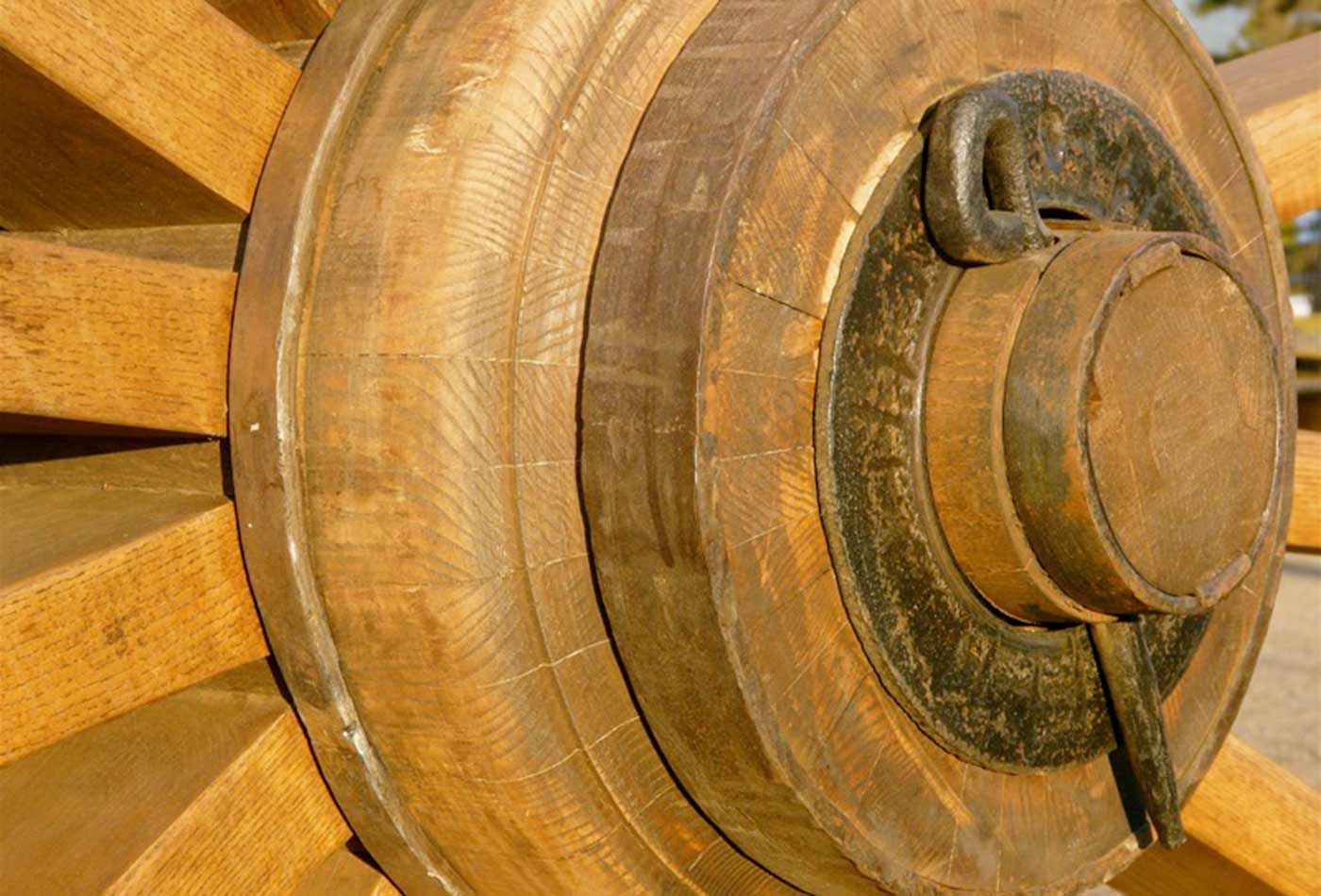
Tim Gasper is director of product and marketing at Bitfusion, a deep learning automation software company enabling easier, faster development of AI applications, and co-founder of Ponos, an IoT-enabled hydroponics farming technology company.
Below, Gasper shares his thoughts on the current and future state of Jupyter. He will also be speaking at JupyterCon, August 22-25, 2017, in New York City.
1. How has Jupyter changed the way you work?
We incorporated Jupyter into our Bitfusion Flex product. It has changed the way our users work by making it much easier to get started with data science, faster to prototype code, and simpler to share very readable code assets with one another.
2. How does Jupyter change the way your team works? How does it alter the dynamics of collaboration?
Jupyter provides a common user interface and sharable hub for code assets among both our internal team at Bitfusion as well as the teams of our customers that use the Bitfusion Flex software bundle.
3. How do you expect Jupyter to be extended in the coming year?
We expect Jupyter to get even stronger support for deep learning and AI workloads, and we expect JupyterLab—the new user interface for Jupyter—to greatly grow in adoption (if they’ll release it out of preview soon!).
4. What will you be talking about at JupyterCon?
We will be discussing how to do deep learning using Jupyter Notebooks, and how Bitfusion has implemented some modifications behind the scenes to make it easier to work with both GPUs and CPUs in a very flexible manner to train deep learning algorithms.
5. What sessions are you looking forward to seeing at JupyterCon?
Building a notebook platform for 100,000 users seems very interesting because of the massive scale that Jupyter implementation had to reach—and what best practices and architecture enabled it.
Jupyter notebooks and production data science workflows also seems very interesting because we’d like to see how Jupyter can become a bigger part of not just initial code development and collaboration around code, but also the production flow of implementing code for end use case applications.