Leading cross-functional conversations about data-driven products
A product manager's guide to employing data as a feature.
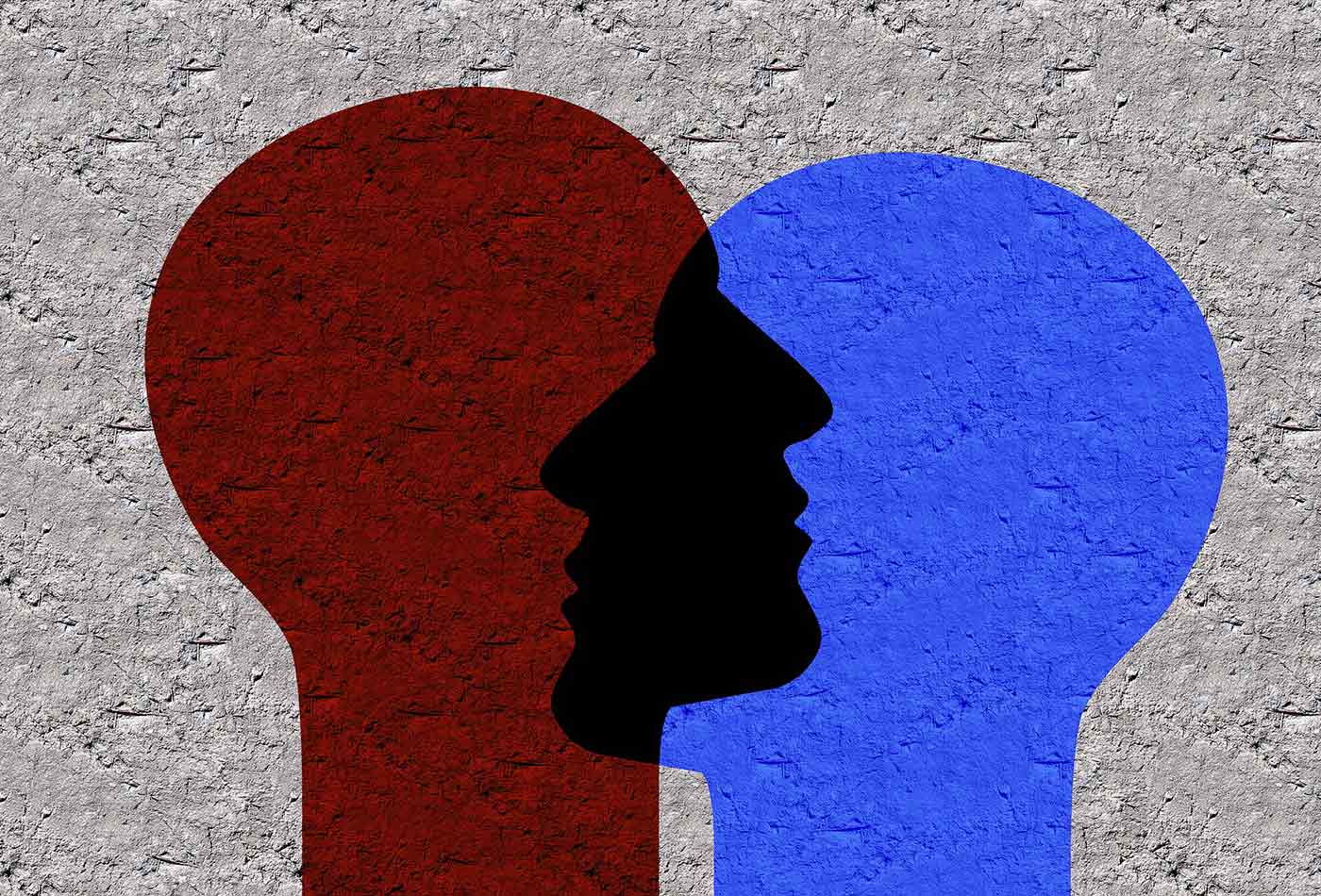
As data becomes more accessible to consumers and enterprises, the demand for actionable data-driven products is growing exponentially across industries. For product managers, treating data not as a resource with intrinsic value but as a feature that needs to be scoped and prioritized according to user need, is a critical way to meet this demand within a specific business context. But creating data-driven products is easier said than done. Precisely because the idea of “data-driven products” sounds so compelling, it is easy for their success to seem like a foregone conclusion if they are sufficiently visually compelling or technically advanced. As a result, far too many “data-driven products” wind up functioning more like expensive technical demos, as opposed to useful products.
For product managers, the key to successfully launching data as a feature is to lead cross-functional conversations that are firmly grounded in an understanding of the user’s goals and needs. What follows is a practical guide to leading cross-functional conversations about data-driven products, starting with the user and avoiding the traps of jargon and justification that sink many well-intentioned data-driven product initiatives.
Start with the user
Why should companies create data-driven products in the first place? To showcase bleeding-edge analysis technology? To unleash insights that were previously locked away in thousands of rows of raw numbers? To create a new revenue stream from the “exhaust” from other products?
The answer can be any of the above—if and only if these products are addressing a real and well-understood set of needs for a real and well-understood set of users.
Far too often, conversations about data-driven products do not start with an understanding of the people for whom the product will be built, but with the wish to show off a particular data set or tool for analysis. As a product manager, it is your job to dig deeper into why this data set or tool is worth showing off, and to look for opportunities to redirect that excitement not toward data or technology for its own sake, but toward how data or technology can be used to meet the needs of your users.
For example, an engineer or data scientist on your product team might be itching to build a product that incorporates a new tool for data storage or analysis that they heard about at a conference. Rather than moving forward under the assumption that this tool will provide value to users—or dismissing it under the assumption that it will not provide value to users—work with the people on your team to understand what makes this tool so exciting. What can it do better than tools you’ve used in the past? What are some examples you’ve seen of how it can be used to address the needs of a specific set of users? And, critically, how might you use it to better address the needs of your users?
Similarly, if individuals within your organization see an opportunity to monetize a particular data set, work with them to understand why and how this data set might be valuable to your users. What is the underlying goal that this data will help users meet? What data, if any, are they currently using to meet that goal? Are competitors offering features rooted in similar data sets?
Remember, your goal is not to discourage people from building data-driven products around the specific data sets and data tools that interest them, but to harness that excitement toward building data-driven products that start with your user in mind.
Avoiding the traps of jargon and justification
Once you’ve done the work of orienting your initial idea for a data-driven product around the needs of your user, you can begin thinking through the downstream design and execution details of how this feature might look and feel. As you begin to scope out your data-driven product, remember to keep the conversation as focused as possible on the needs of your users and how your data-driven product can meet those needs. Doing so will help you avoid the two most common traps that befall teams working on data-driven products: jargon and justification.
The trap of jargon can be difficult to avoid when working on data-driven products. Data science often incorporates both engineering jargon and mathematical jargon, as well as its own ever-changing landscape of jargon. Using this jargon can feel like a way to demonstrate your expertise and build camaraderie with your technical counterparts. But jargon has an intrinsic pull against the needs and interests of your user. It fragments your product team into those who “get it” and those who don’t, and it often ascribes unwarranted importance to the most arcane, complex, or newfangled solutions. You should always do your best to make sure your product team is speaking the language of your user—and in most cases, that language is not jargon.
The trap of justification can prove a bit trickier to diagnose, and to treat. Generally speaking, teams fall into this trap when they justify what they already want to build with user input, rather than letting that input guide the product from the outset. This is a common trap for all product teams, but it becomes particularly insidious when working on data-driven products—for the very reason that “data-driven” products are so easy to justify.
One surefire sign that your team may have fallen into both of these traps at the same time is that the feedback you are receiving from users starts to sound a lot like a technical spec, rather than a statement of needs and goals. Unless you are dealing with a very technical and specialized user base, technical language in user interviews and feedback usually suggests that users are being led to justify technical decisions already made by the product team—especially when those decisions involve jargon.
Summary: Simple, but not easy
The key to leading cross-functional conversations about data-driven products is not to advocate for the most technically clever solution, or the solution that uses the most data. Nor is it to “talk the talk” of data and analytics, and deploy a cutting-edge technical vocabulary. Instead, it is to keep the conversation accessible across all functions and firmly grounded in the goals and needs of your users. This may sound simple, but it is definitely not easy. To summarize, here are a few things to keep in mind:
- If there is excitement about a particular data set or analysis technology, dig deeper into the “why” and discover how that data set or technology could help your users meet their goals.
- Avoid falling into the jargon trap, which will fragment your cross-functional team and further remove you from the reality of your users.
- Let user feedback and insights guide your implementation decisions—don’t treat users as a mechanism for justifying what you already want to build because you think it’s cool or interesting.
- Remember that simply providing data to your users does not intrinsically provide them with value. Subject data-driven products to the same rigorous validation and prioritization standards that you would any other product or feature.
This post is a collaboration between O’Reilly and TIBCO Jaspersoft. See our statement of editorial independence.