Leveraging analytics 1.0 for the analytics 2.0 revolution
Rather than hiring data scientists from outside, consider training your proto data scientists.
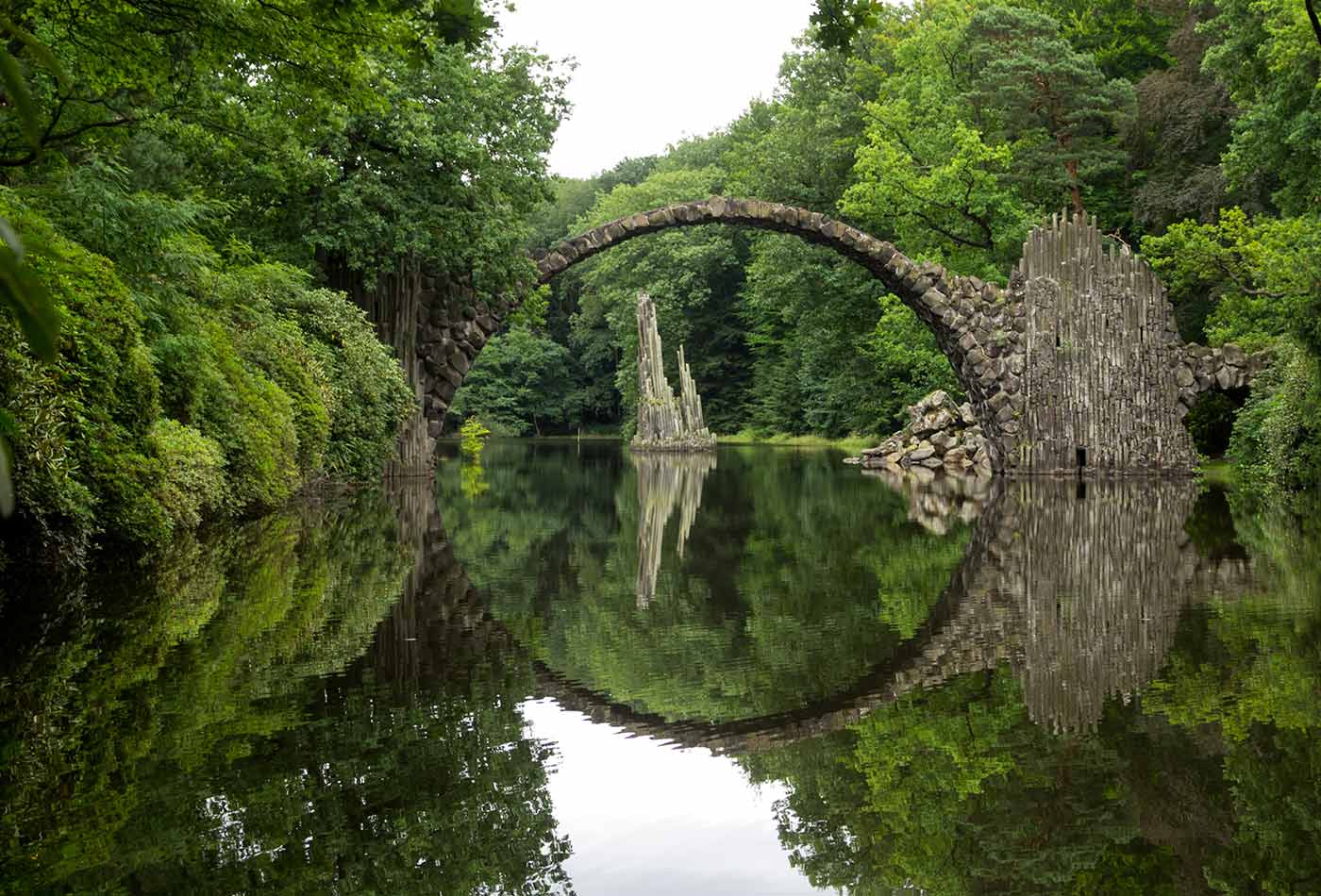
Big data and data science is so much in vogue that we often forget there were plenty of professionals analyzing data before it became fashionable. This can be thought of as a divide between Analytics 1.0, practiced by those in traditional roles like data analysts, quants, statisticians, and actuaries, and Analytics 2.0, characterized by data scientists and big data. Many companies scrambling to hire data science talent have begun to realize the wealth of latent analytics talent right at their fingertips — talent capable of becoming data scientists with a little bit of training. In other words, the divide between Analytics 1.0 and 2.0 is not as wide as you might believe.
Analytics 1.0 professionals come from many industries, including finance, health care, government, and technology. But they all share the same core technical skill sets around computation and statistics that make them ideal candidates for training to get them caught up on data science. In addition to possessing the foundational data science skills, these employees already understand the industry needs — often being corporate veterans. Of course, these advantages are not without their challenges, and from my experience, the three main challenges revolve around learning new computational techniques, new statistical techniques, and a new mindset. Let’s walk through each one.
Learning new computational techniques
Analytics 2.0 is defined by the sheer volume and variety of data available. Data scientists need strong computational skills to handle the ever-increasing size of data sets and computational complexity. One such important new skill is parallelization and distributed computing — breaking up a large computational load across a network of computers. But successfully leveraging parallelization requires understanding how to orchestrate an ensemble of computers and imposes severe limits on what tasks can and cannot be parallelized. Developing the skills to cope with the sheer scale of data is an integral part of Analytics 2.0.
The variety of data types is also a major challenge. Analytics 1.0 utilizes data sets that are clean, structured, and single-sourced. In contrast, Analytics 2.0 is focused on data sets that are messy, unstructured, and multi-sourced, and practitioners require greater software engineering capabilities to clean, structure, and merge multiple sources together.
Learning new statistical analyses
The uninitiated are often under the mistaken impression that big data is just doing the same analytics on more data. This is often wrong in two major ways.
First, larger data allows us to leverage more powerful techniques that are simply not useful for smaller data sets. Understanding highly nuanced customer preferences on relatively narrow segments is completely predicated on having enough data to be able to make statistically sound inferences on subtle effects on narrow user groups. Throwing a deep neuro-network on a tiny data set is a recipe for disaster (although that doesn’t seem to prevent some managers from asking for it).
Secondly, even if you’re still running the same analyses mathematically, the sheer scale of the data presents new challenges. How do you take a mean if you can’t fit all your data onto your laptop? How can your analyses keep up if it takes more than 24 hours to analyze 24 hours of data? Parallelizing across multiple machines is expensive and only works in certain cases. Understanding how to make the appropriate tradeoff between statistical rigor and computational feasibility is an integral skill in joining Analytics 2.0.
Finally, and in contrast to Analytics 1.0, many novel Analytics 2.0 data sources are not collected for the analysis at hand. Analyzing data byproducts requires a greater awareness of the biases in data and is just one of the many reasons data scientists have to increase their statistical knowledge.
Learning new mindsets
Beyond technical skills, Analytics 2.0 requires a completely new mindset shift. While Analytics 1.0 focused on collecting clean data tailor fit for the analysis at hand, Analytics 2.0 revolves around cleverly mining the seemingly unlimited exhaust of data collected for third-party purposes, often by other organizations, and leveraging it for novel uses in your own organization. For example: the pharmaceutical industry’s push to move from just clinical effectiveness to real-world, outside-the-laboratory effectiveness; marketing’s reliance on fine-grain app and web behavioral data; brick-and-mortar retailers’ embrace of location data from mobile users to predict demand based on address; agriculture’s use of satellite images to understand soil quality and crop yields — these are just the tip of the Analytics 2.0 iceberg, but they require a profoundly different skill set.
Rather than conducting the same canned analyses on the same types of data, new analytics professionals must seek out novel data sets and leverage their creativity to come up with these novel use cases. They don’t just determine the answers — they must determine the questions.
What this means for employers
Given the difficulty and expenses associated with hiring data scientists outright, companies are turning to their latent data science professionals to fulfill these roles. I have seen that training people skilled in the fundamentals and already immersed in the organization and industry can help companies get a jump start on building Analytics 2.0 teams. I’m always interested to hear how training has worked in practice at companies and how they connect with my experiences providing big data training. Feel free to contact me at michael.li@thedataincubator.com with your stories.