Streaming data + stream processing = information
John Hugg discusses the business payoffs of stream processing with transactions.
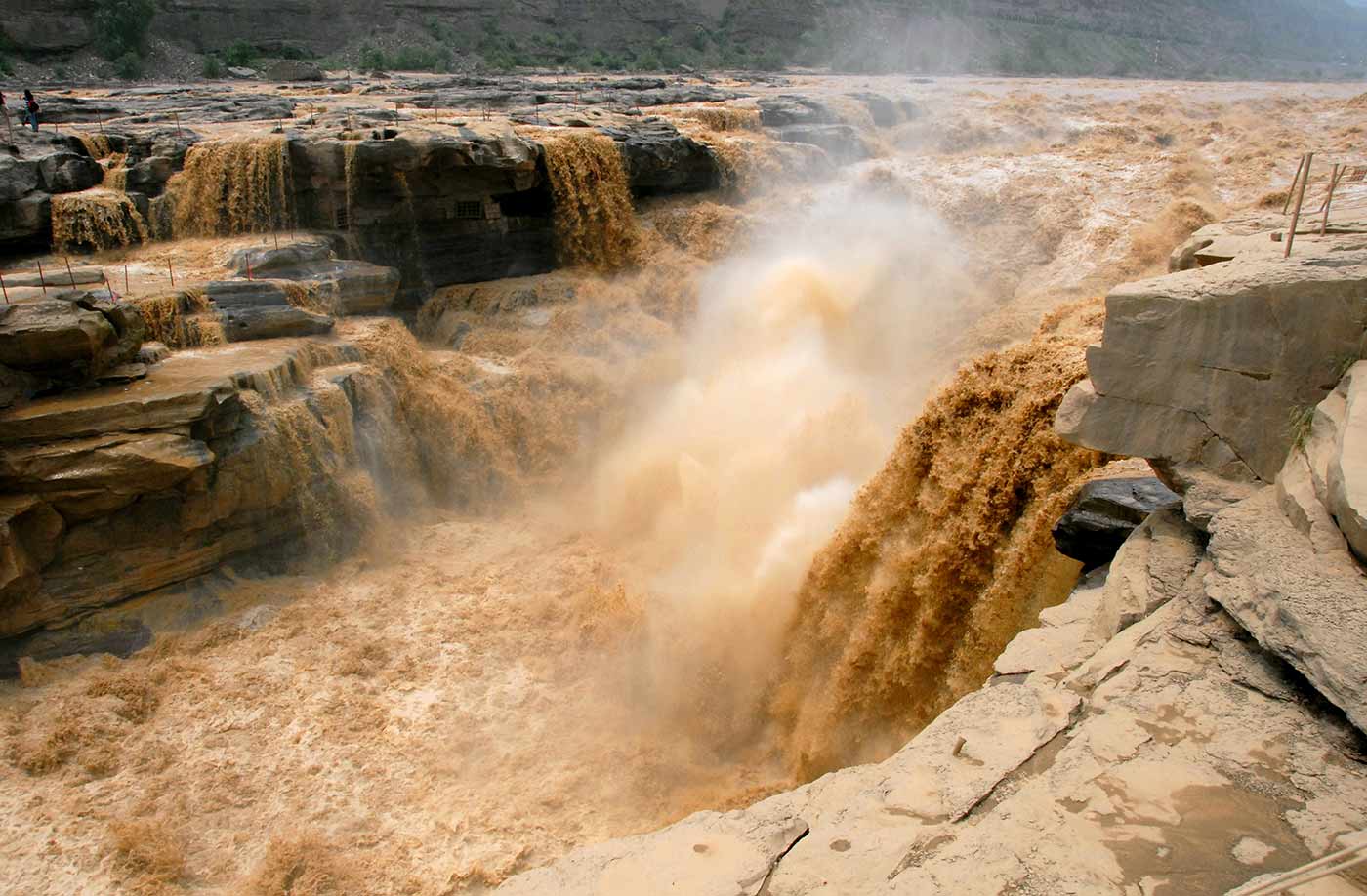
In this O’Reilly Podcast, Ben Lorica talks with John Hugg, founding engineer and manager of developer outreach at VoltDB. From the deluge of today’s diverse data, Hugg describes how to extract meaningful information to make things cheaper and faster.
Streaming vs. traditional analytics
One of the main themes Hugg and Lorica cover is the tradeoff between time-consuming batch processing of a large, already collected data set and fast processing of streaming data, supported by transactions on that live data. Hugg explains:
With streams, you typically need to know the kinds of questions, the kinds of actions, the alerts before you go and collect that data. As the data is being collected, you apply analysis and you get the answers to those questions, you get the answers from the data, but you need to set up the questions in advance. Whereas with traditional analytics, you collect the data and then you ask the questions.
Streaming is all about fresh understanding, being able to leverage the power of big data analytics at the time that the event happens, often so that you can use that information you get at ingestion time to take some action, to change how you respond to that event. You can’t do that with batch.
Low latency, real time
When response times can be brought down to the millisecond, there are interesting real-time applications, such as fraud detection and compliance verification.
Our goal is to achieve a millisecond or two response. That lets Volt be injected into the active path of a lot of kinds of applications. We have fraud detection applications in production, where between swiping a credit card and the transaction being approved or denied, a Volt transaction is made to decide if this is a transaction we want to let go through.
Exactly once: Have I done this before?
In applications like billing, there is significant value in reliably knowing the answer to the question, “Have I done this before?”
What [MaxCDN, a leading content delivery network] told us is that the number of look-ups they had to do using HBase and Storm was about a million times higher [than] with Volt, and we thought they were exaggerating, but they weren’t—we did the math. Because they only have to check once for these updates in VoltDB as compared to running many, many back and forth checks into HBase to see if they’ve done these counters before. It allows them to get consistent, accurate billing on a much, much, much smaller cluster, and they’ve got CPU headroom to spare.
Micro-personalization
When content generators can customize experiences per event, per consumer, during the live event, the result can be beneficial to both generators and consumers.
Trying to predict where things are going and being able to handle the flood of the user doing a bazillion things—some small number of these things are interesting. To be able to pick those interesting things out, combine them with the other interesting things based on trends going on globally and being able to inject yourself into the path of drawing a new Web page or a new app screen for this user, and customize that based on all those different variables allows you to be more accurate.
This post and podcast is part of a collaboration between O’Reilly an d VoltDB. See our statement of editorial independence.