Strengthen your digital defenses with AI and supervised machine learning
Use cases of AI and ML to help businesses build better defenses today and in the near future.
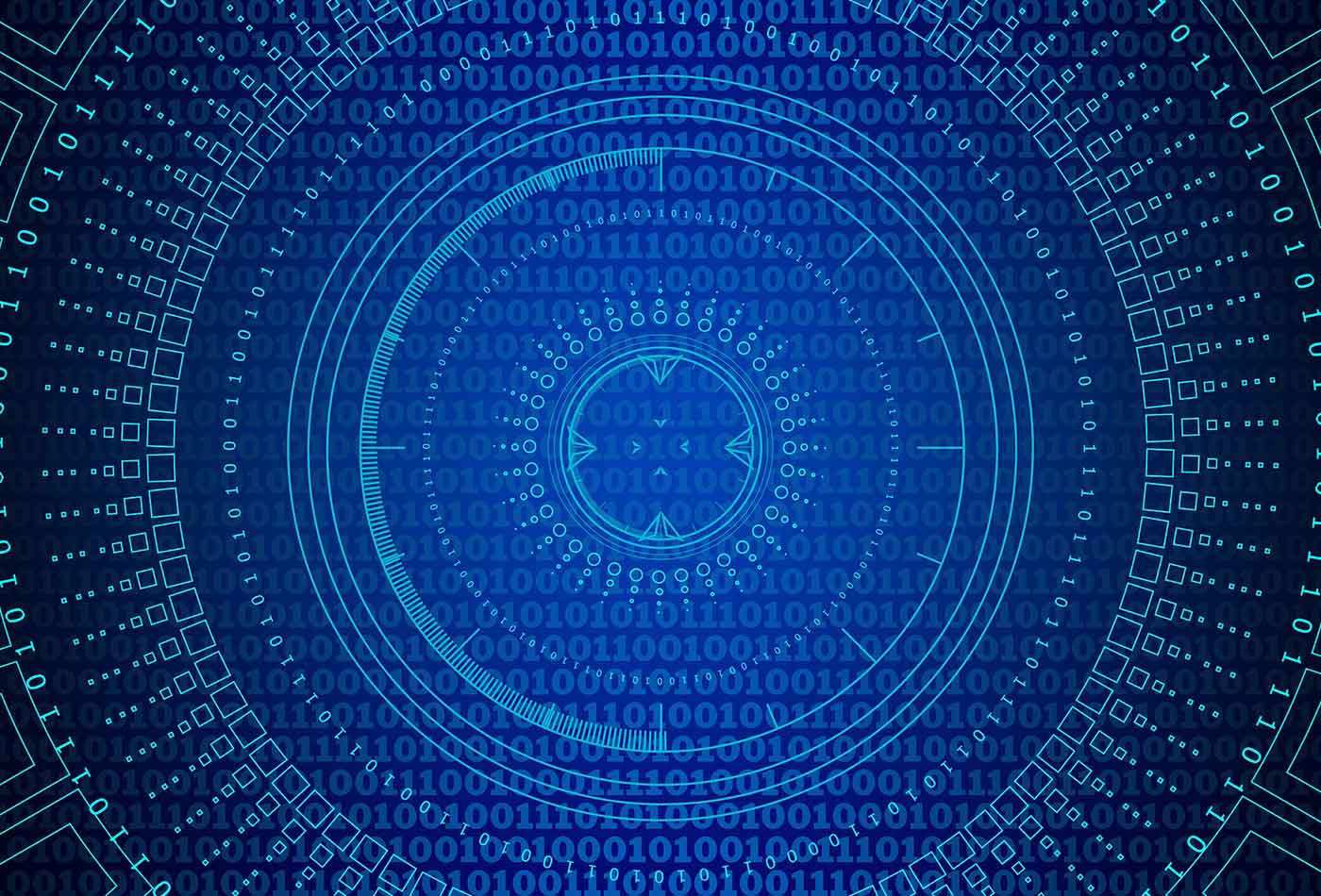
In the context of security, there are many discussions on how artificial intelligence (AI) and machine learning (ML) can be leveraged for malicious purposes. For example, in the recently released report The Malicious Use of Artificial Intelligence: Forecasting, Prevention, and Mitigation, you will find a list of scenarios where AI and ML have the potential to be used to attack business assets physically, politically, and digitally. Possible cybersecurity threat scenarios include sophisticated and automated malicious hacking, human-like denial-of-services attacks, prioritized and targeted ML attacks, and the exploitative use of AI in applications related to information security.
While there is much discourse on how AI and ML can be leveraged by attackers, significantly less focus has been given as to how these advanced analytics tools can be leveraged for improved digital defenses. However, it is valuable to shift the lens to see how AI and ML can detect and block attacks. A prime example of this is an AI system designed by security researchers at MIT called AI2 that is able to detect 85% of cybersecurity attacks. This article outlines possible use cases of AI and ML that can realistically help your business build better defenses today and in the near future.
Defining AI and ML: Tightly intertwined but separate
First, definitions for AI and ML are needed to set the framework for subsequent discussion in this article. Although the IT industry has a tendency to use the terms AI and ML interchangeably, they are not the same thing. AI is defined as the theory and development of computer systems able to perform tasks that normally require human intelligence, such as visual perception, speech recognition, decision-making, and translation between languages. The term “AI” is applied when a machine mimics cognitive functions that humans associate with human cognitive functions, such as learning and problem solving.
Machine learning is an application of artificial intelligence that provides systems the ability to automatically learn and improve from experience without being explicitly programmed. Machine learning focuses on the development of computer programs that can access data and use it to learn for themselves. The more machines are trained, the “smarter” they become. In the current defense landscape, it is ML that is more established and therefore more likely to be used defensively as compared to AI. ML is frequently referred to as supervised machine learning (SML), as opposed to unsupervised machine learning. With supervised ML, the human is responsible for training the machine, and the machine is capable of learning with the help of humans as feedback systems. The uses cases presented in this article are primarily supervised ML.
Starting with supervised ML-based defenses for security events and log analysis
The use of supervised ML can greatly speed and assist the work of security analysts and engineers to identify and mitigate exact threat sources. These analysts and engineers are tasked with viewing security events and logs, and then analyzing multitudes of data points generated from the deployed security controls such as firewalls, IPSs, IDSs, sandboxes, WAFs, EPP solutions, and PAM solutions. Combing through collected data to pinpoint specific security threats can take weeks, even months, to accomplish. Taking steps to mitigate the threats takes even longer. Most analysts and engineers already carry a full workload, making it harder for them to expand responsibilities. Hiring more resources to ferret out attacks is often not possible. All the while, malicious activities are wreaking havoc on your online presence and performance—and damaging your business revenue and reputation along the way.
Products are already on the market that employ human-based supervised ML. For most of these products, an IT security analyst initially provides feedback to the AI engines that are at work scanning massive amounts of log entries to identify anomalous behaviors. The supervised ML system augments the analyst by being trained to improve detection of “significant events” in the logs and to immediately bring those events to the analyst’s attention, which means that your business is more quickly on the path to threat resolution.
Automating analyst responses and communications around identified threats
Once an analyst detects something of interest in the company’s security logs, the most likely next step is alerting an IT security engineer. Using the data provided by the security analyst, the engineer may opt to make configuration changes on various security controls and quarantine systems that appear to be security risks. The analyst might also share the source address of the offending machine to a threat intelligence feed of some sort. These activities do not always happen quickly, and can leave your business at risk in the meantime.
The reality is, analysts and engineers often replicate these processes daily. An AI solution that could automate these repeated actions to identify threats more quickly could greatly strengthen digital security posture. It is also quite likely that AI and ML can be used to replicate the interaction performed between the analyst and the engineer, as well as the interaction between the engineer and the security controls. Looking ahead, it is easy to envision a solution that acts on security alerts and uses AI to automatically make configuration changes to various security controls in the same fashion as the analyst and the engineer. While human oversight is still a critical part of the solution, less time is required from the analysts and engineers, thus freeing them to perform other critical activities.
Taking proactive steps to “train” security controls
Integrating ML-enabled engines into security controls like firewalls, WAFs, and IPSs during the ongoing configuration and tuning processes is increasingly a viable cybersecurity tool. Today, there are already AI-enabled WAFs that can be trained to identify both good and malicious traffic as well as traffic logs to “teach” the machine what is considered good versus malicious. The logs generate what is considered training data, but you can also add real-time request data and/or requests generated by web application scanners to the training data.
This training data is then used to generate and run a security model. Once the model is run, a human then begins to train the AI engine to improve the accuracy of what it deems benign or malicious. The model is then run again, sometimes with additional training data. This approach is actively supervised learning, and it requires human and machine working together to reduce (and eventually eliminate) false positives while improving the model’s accuracy over time.
Building a realistic machine-aided digital defense
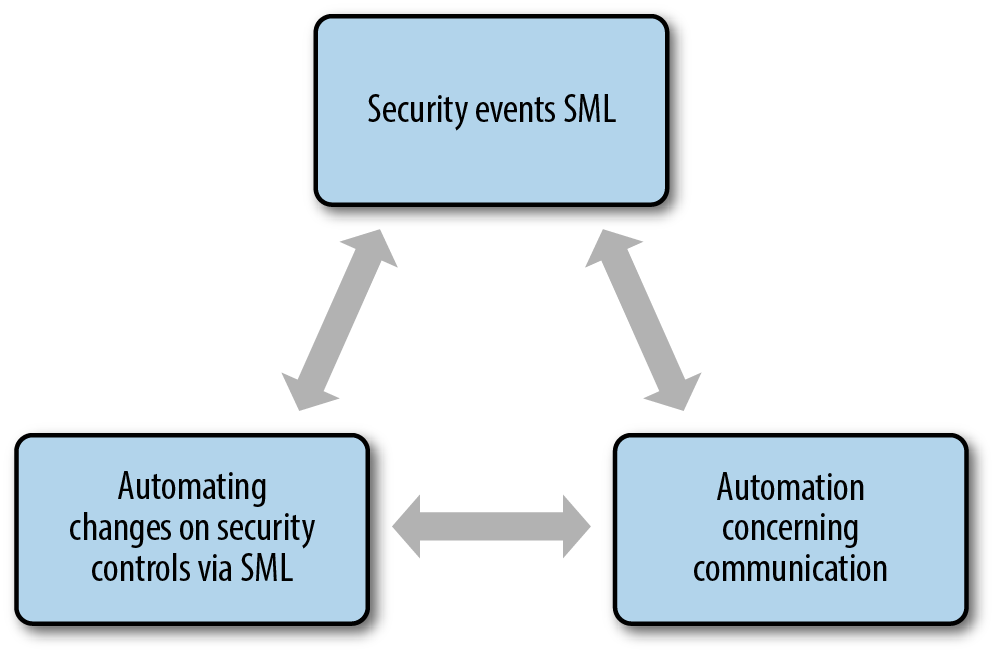
The ultimate goal is to increasingly add automation via supervised ML into the three key phases of digital defense—security events and analysis, security responses and communications, and security controls—with active human involvement at the core. It’s true that some of the defensive approaches discussed here for battling the onslaught of attacks are not yet available solutions. But all bolster the realistic promise of how AI and ML can and will be used increasingly for cybersecurity, with a very high likelihood of more products coming to market in the current decade.
This post is a collaboration between O’Reilly and Oracle Dyn. See our statement of editorial independence.