What is a reactive microservice?
Explore the factors that make up a reactive microservice.
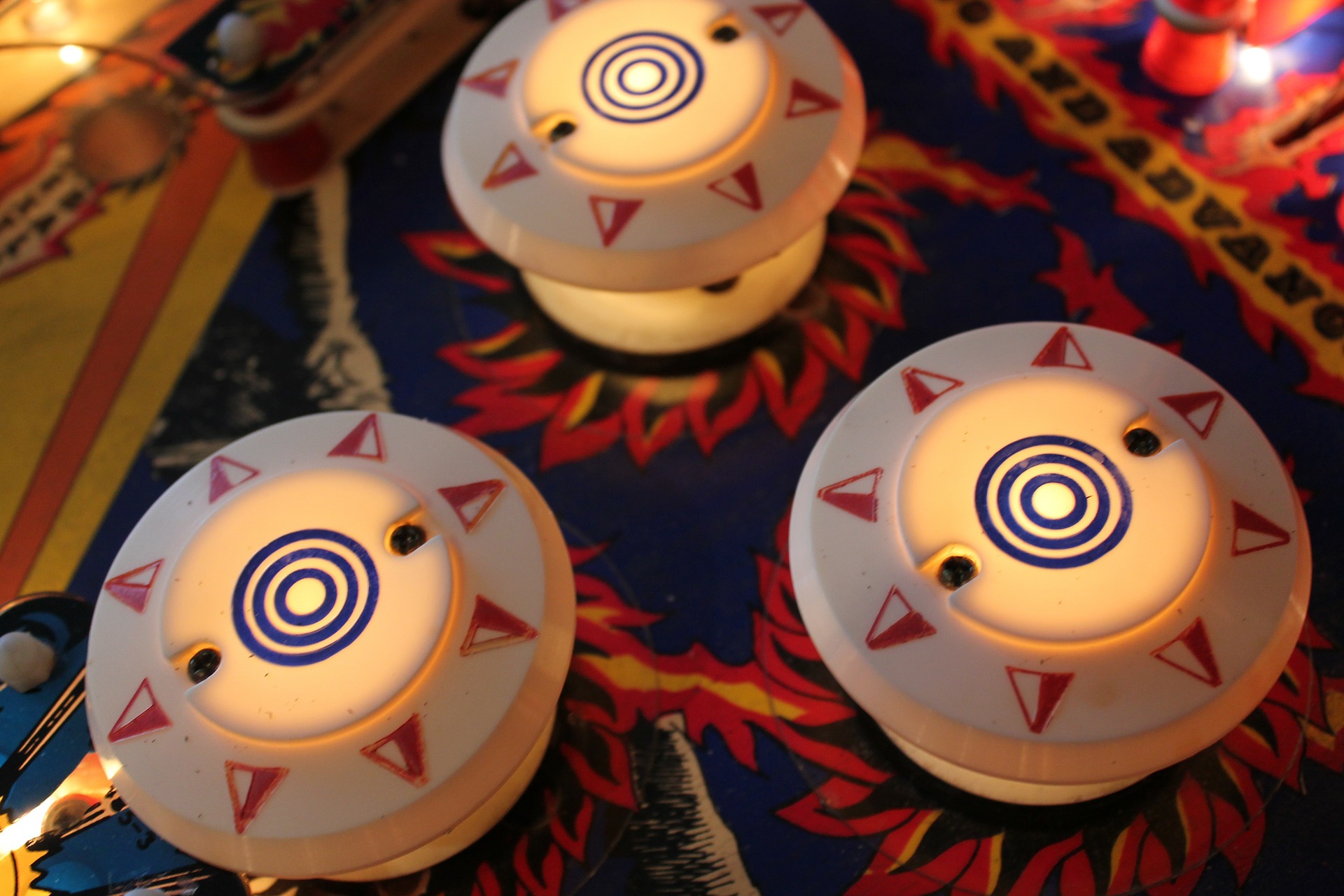
One of the key principles in employing a Microservices-based Architecture is Divide and Conquer: the decomposition of the system into discrete and isolated subsystems communicating over well-defined protocols.
Isolation is a prerequisite for resilience and elasticity and requires asynchronous communication boundaries between services to decouple them in:
- Time
- Allowing concurrency
- Space
- Allowing distribution and mobility—the ability to move services around
When adopting Microservices, it is also essential to eliminate shared mutable state1 and thereby minimize coordination, contention and coherency cost, as defined in the Universal Scalability Law2 by embracing a Share-Nothing Architecture.
At this point in our journey, it is high time to discuss the most important parts that define a Reactive Microservice.
Isolate All the Things
Without great solitude, no serious work is possible.
Pablo Picasso
Isolation is the most important trait. It is the foundation for many of the high-level benefits in Microservices. But it is also the trait that has the biggest impact on your design and architecture. It will, and should, slice up the whole architecture, and therefore it needs to be considered from day one. It will even impact the way you break up and organize the teams and their responsibilities, as Melvyn Conway discovered and was later turned into Conway’s Law in 1967:
Any organization that designs a system (defined broadly) will produce a design whose structure is a copy of the organization’s communication structure.
Failure isolation—to contain and manage failure without having it cascade throughout the services participating in the workflow—is a pattern sometimes referred to as Bulkheading.
Bulkheading has been used in the ship construction for centuries as a way to “create watertight compartments that can contain water in the case of a hull breach or other leak.”3 The ship is divided into distinct and completely isolated watertight compartments, so that if compartments are filled up with water, the leak does not spread and the ship can continue to function and reach its destination.
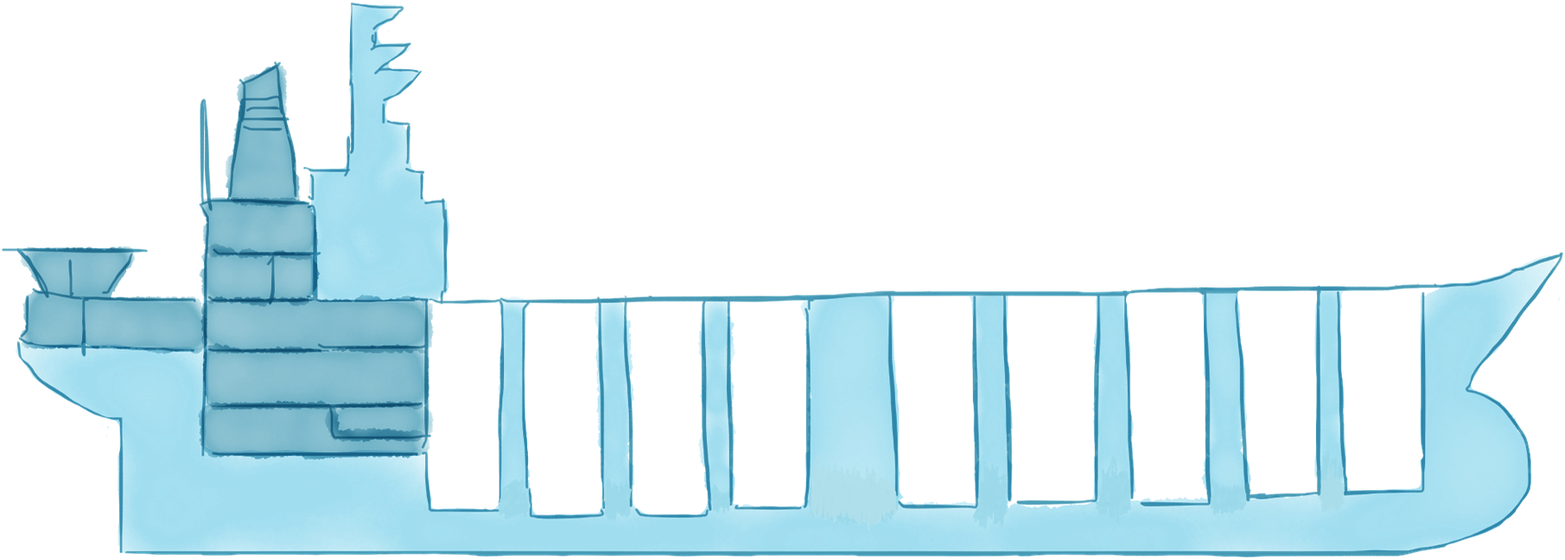
Some people might come to think of the Titanic as a counter-example. It is actually an interesting study4 in what happens when you don’t have proper isolation between the compartments and how that can lead to cascading failures, eventually taking down the whole system. The Titanic did use bulkheads, but the walls that were suppose to isolate the compartments did not reach all the way up to the ceiling. So when 6 out of its 16 compartments were ripped open by the iceberg, the ship started to tilt and water spilled over from one compartment to the next, until all of the compartments were filled with water and the Titanic sank, killing 1500 people.
Resilience—the ability to heal from failure—depends on compartmentalization and containment of failure, and can only be achieved by breaking free from the strong coupling of synchronous communication. Microservices communicating over a process boundary using asynchronous message-passing enable the level of indirection and decoupling necessary to capture and manage failure, orthogonally to the regular workflow, using service supervision.5
Isolation between services makes it natural to adopt Continuous Delivery. This allows you to safely deploy applications and roll out and revert changes incrementally—service by service.
Isolation also makes it easier to scale each service, as well as allowing them to be monitored, debugged and tested independently—something that is very hard if the services are all tangled up in the big bulky mess of a monolith.
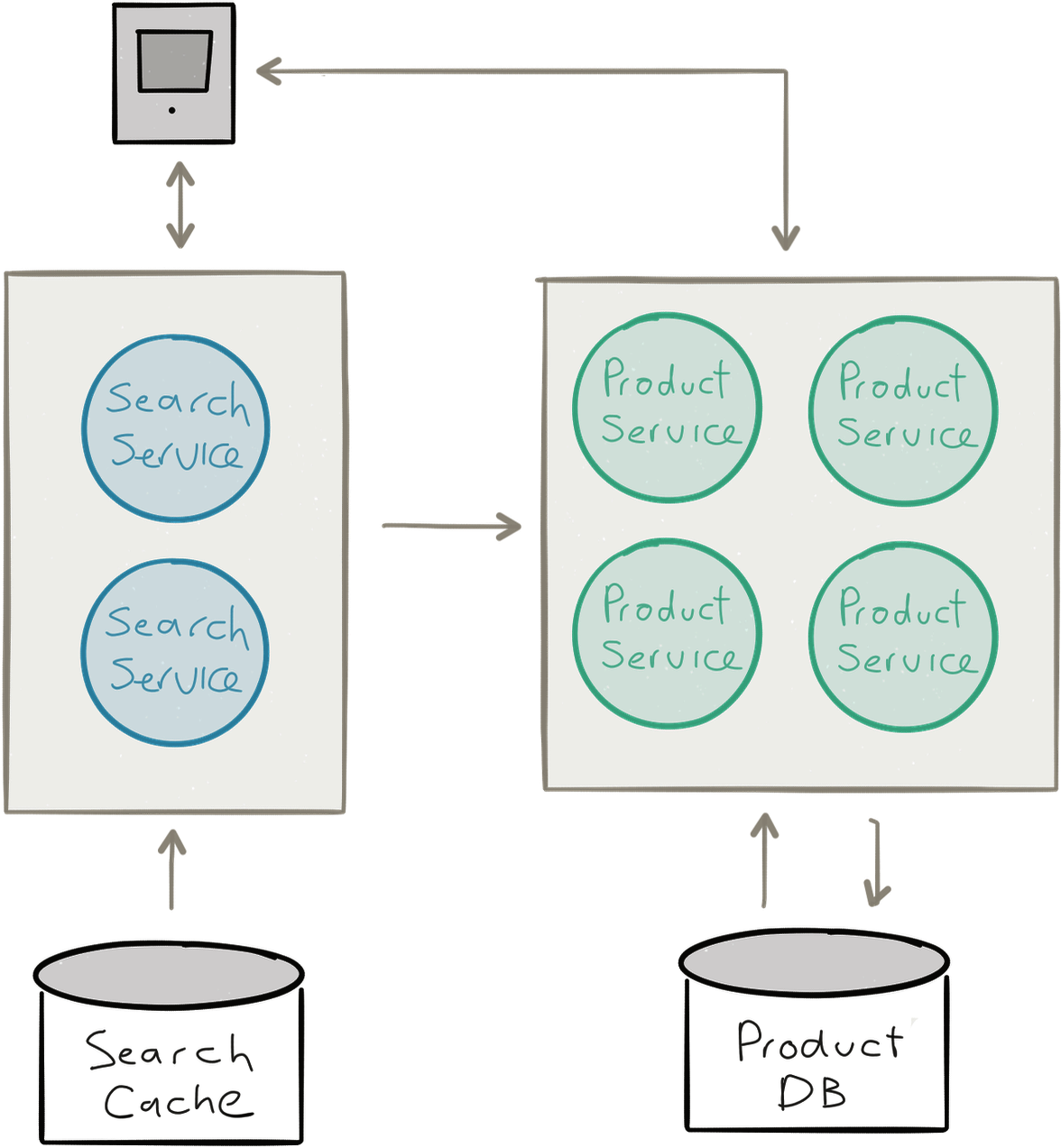
Act Autonomously
Insofar as any agent acts on reason alone, that agent adopts and acts only on self-consistent maxims that will not conflict with other maxims any such agent could adopt. Such maxims can also be adopted by and acted on by all other agents acting on reason alone.
Law of Autonomy by Immanuel Kant
Isolation is a prerequisite for autonomy. Only when services are isolated can they be fully autonomous and make decisions independently, act independently, and cooperate and coordinate with others to solve problems.
An autonomous service can only promise6 its own behaviour by publishing its protocol/API. Embracing this simple yet fundamental fact has profound impact on how we can understand and model collaborative systems with autonomous services.
Another aspect of autonomy is that if a service only can make promises about its own behavior, then all information needed to resolve a conflict or to repair under failure scenarios are available within the service itself, removing the need for communication and coordination.
Working with autonomous services opens up flexibility around service orchestration, workflow management and collaborative behavior, as well as scalability, availability and runtime management, at the cost of putting more thought into well-defined and composable APIs that can make communication—and consensus—a bit more challenging—something we will discuss shortly.
Do One Thing, and Do It Well
This is the Unix philosophy: Write programs that do one thing and do it well. Write programs to work together.
Doug McIlroy
The Unix philosophy7 and design has been highly successful and still stands strong decades after its inception. One of its core principles is that developers should write programs that have a single purpose, a small well-defined responsibility and compose well with other small programs.
This idea was later brought into the Object-Oriented Programming community by Robert C. Martin and named the Single Responsibility Principle (SRP),8 which states a class or component should “only have one reason to change.”
There has been a lot of discussion around the true size of a Microservice. What can be considered “micro”? How many lines of code can it be and still be a Microservice? These are the wrong questions. Instead, “micro” should refer to scope of responsibility, and the guiding principle here is the Unix philosophy of SRP: let it do one thing, and do it well.
If a service only has one single reason to exist, providing a single composable piece of functionality, then business domains and responsibilities are not tangled. Each service can be made more generally useful, and the system as a whole is easier to scale, make resilient, understand, extend and maintain.
Own Your State, Exclusively
Without privacy there was no point in being an individual.
Jonathan Franzen
Up to this point, we have characterized Microservices as a set of isolated services, each one with a single area of responsibility. This forms the basis for being able to treat each service as a single unit that lives and dies in isolation—a prerequisite for resilience—and can be moved around in isolation—a prerequisite for elasticity.
While this all sounds good, we are forgetting the elephant in the room: state.
Microservices are often stateful entities: they encapsulate state and behavior, in similar fashion to an Object or an Actor, and isolation most certainly applies to state and requires that you treat state and behavior as a single unit.
Unfortunately, ignoring the problem by calling the architecture “stateless”—by having “stateless” controller-style services that are pushing their state down into a big shared database, like many web frameworks do—won’t help as much as you would like and only delegate the problem to a third-party, making it harder to control—both in terms of data integrity guarantees as well as scalability and availability guarantees (see Figure 1-3).
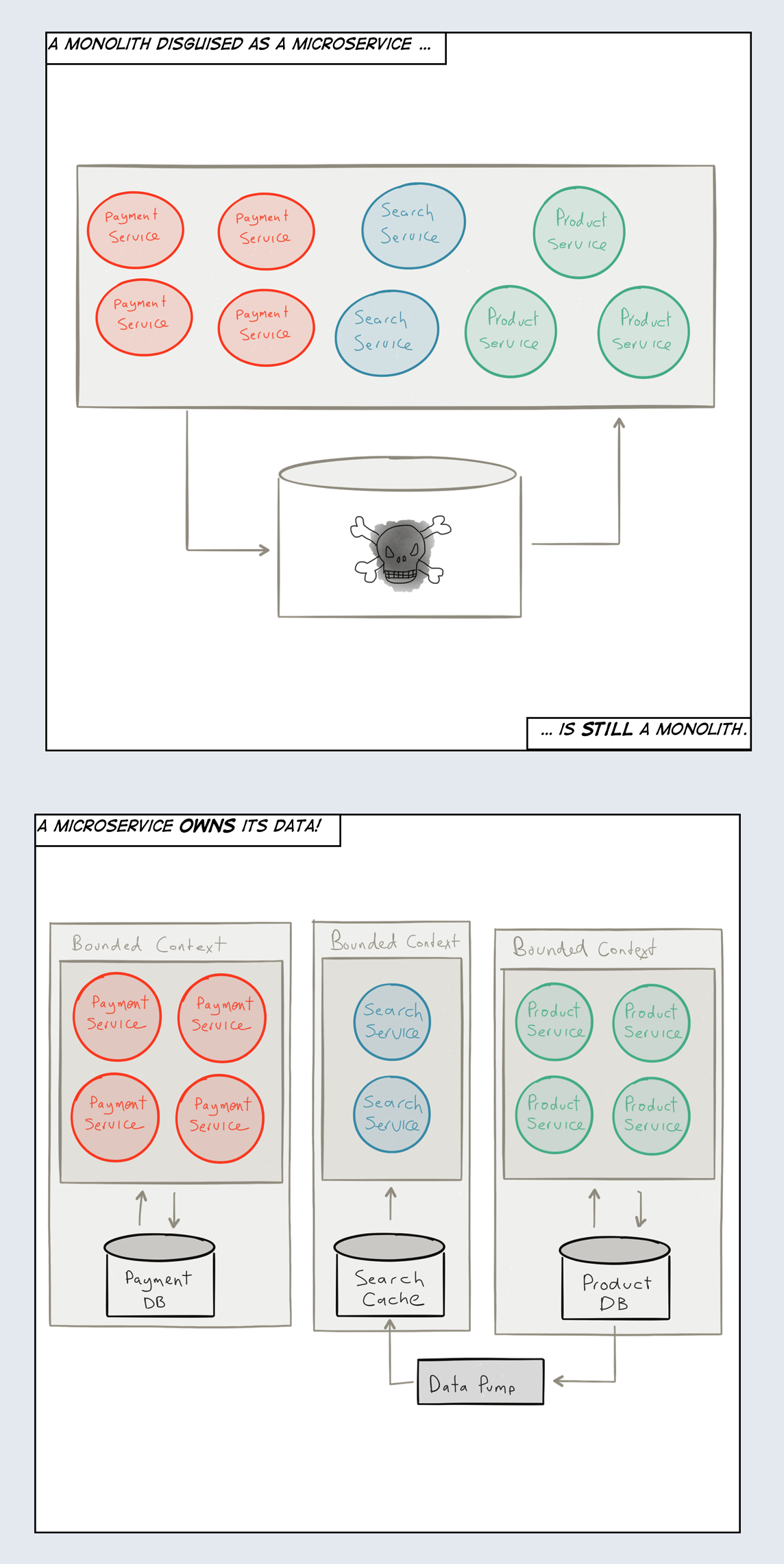
What is needed is that each Microservice take sole responsibility for their own state and the persistence thereof. Modeling each service as a Bounded Context9 can be helpful since each service usually defines its own domain, each with its own Ubiquitous Language. Both these techniques are taken from the Domain-Driven Design (DDD)10 toolkit of modeling tools. Of all the new concepts introduced here, consider DDD a good place to start learning. Microservices are heavily influenced by DDD and many of the terms you hear in context of Microservices come from DDD.
When communicating with another Microservice, across Bounded Contexts, you can only ask politely for its state—you can’t force it to reveal it. Each service responds to a request at its own will, with immutable data (facts) derived from its current state, and never exposes its mutable state directly.
This gives each service the freedom to represent its state in any way it wants, and store it in the format and medium that is most suitable. Some services might choose a traditional Relational Database Management System (RDBMS) (examples include Oracle, MySQL and Postgres), some a NoSQL database (for example Cassandra and Riak), some a Time-Series database (for example InfluxDB and OpenTSDB) and some to use an Event Log11 (good backends include Kafka, Amazon Kinesis and Cassandra) through techniques such as Event Sourcing12 and Command Query Responsibility Segregation (CQRS).
There are benefits to reap from decentralized data management and persistence—sometimes called Polyglot Persistence. Conceptually, which storage medium is used does not really matter; what matters is that a service can be treated as a single unit—including its state and behavior—and in order to do that each service needs to own its state, exclusively. This includes not allowing one service to call directly into the persistent storage of another service, but only through its API—something that might be hard to enforce programmatically and therefore needs to be done using conventions, policies and code reviews.
An Event Log is a durable storage for the messages. We can either choose to store the messages as they enter the service from the outside, the Commands to the service, in what is commonly called called Command Sourcing. We can also choose to ignore the Command, let it perform its side-effect to the service, and if the side effect triggers a state change in the service then we can capture the state change as a new fact in an Event to be stored in the Event Log using Event Sourcing.
The messages are stored in order, providing the full history of all the interactions with the service and since messages most often represent service transactions, the Event Log essentially provides us with a transaction log that is explicitly available to us for querying, auditing, replaying messages (from an arbitrary point in time) for resilience, debugging and replication—instead of having it abstracted away from the user as seen in RDBMSs. Pat Helland puts it very well:
“Transaction logs record all the changes made to the database. High-speed appends are the only way to change the log. From this perspective, the contents of the database hold a caching of the latest record values in the logs. The truth is the log. The database is a cache of a subset of the log. That cached subset happens to be the latest value of each record and index value from the log.”13
Command Sourcing and Event Sourcing have very different semantics. For example, replaying the Commands means that you are also replaying the side effects they represent; replaying the Events only performs the state-changing operations, bringing the service up to speed in terms of state. Deciding the most appropriate technique depends on the use case.
Using an Event Log also avoids the Object-Relational Impedance Mismatch, a problem that occurs when using Object-Relational Mapping (ORM) techniques and instead builds on the foundation of message-passing and the fact that it is already there as the primary communication mechanism. Using an Event Log is often the best persistence model for Microservices due to its natural fit with Asynchronous Message-Passing (see Figure 1-4).
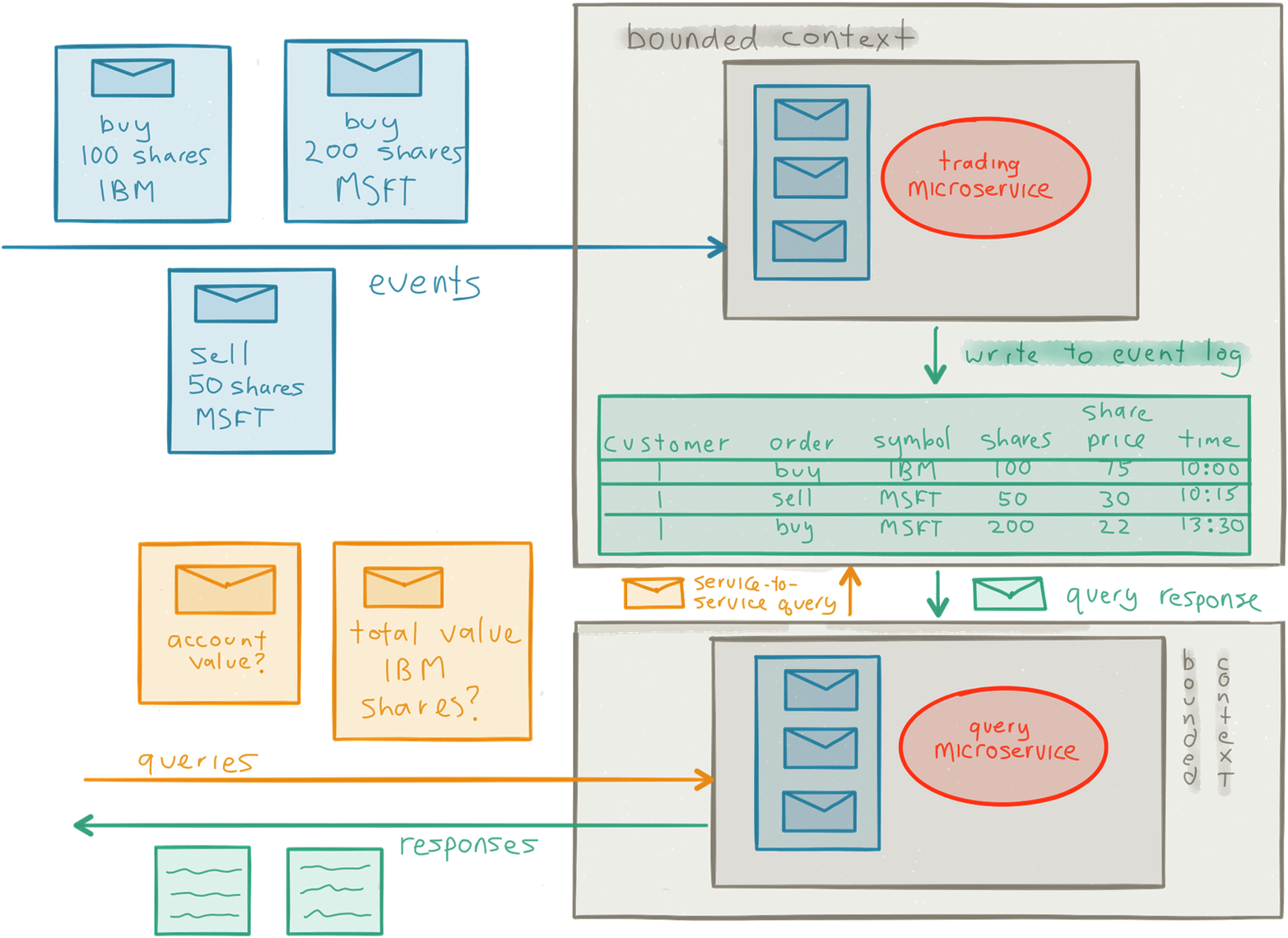
Embrace Asynchronous Message-Passing
Smalltalk is not only NOT its syntax or the class library, it is not even about classes. I’m sorry that I long ago coined the term “objects” for this topic because it gets many people to focus on the lesser idea. The big idea is ‘messaging’.
Alan Kay
Communication between Microservices needs to be based on Asynchronous Message-Passing (while the logic inside each Microservice is performed in a synchronous fashion). As was mentioned earlier, an asynchronous boundary between services is necessary in order to decouple them, and their communication flow, in time—allowing concurrency—and in space—allowing distribution and mobility. Without this decoupling it is impossible to reach the level of compartmentalization and containment needed for isolation and resilience.
Asynchronous and non-blocking execution and IO is often more cost-efficient through more efficient use of resources. It helps minimizing contention (congestion) on shared resources in the system, which is one of the biggest hurdles to scalability, low latency, and high throughput.
As an example, let’s take a service that needs to make 10 requests to 10 other services and compose their responses. Let’s say that each requests takes 100 milliseconds. If it needs to execute these in a synchronous sequential fashion the total processing time will be roughly 1 second (Figure 1-5).
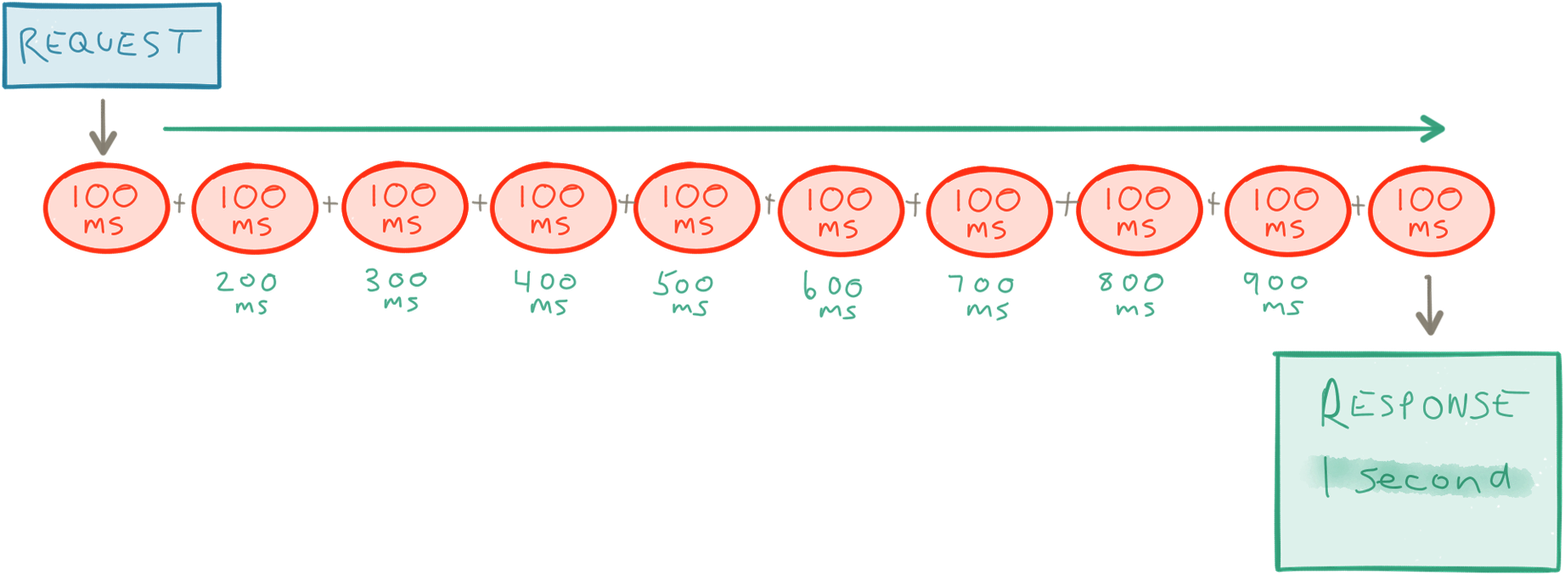
Whereas if it is able to execute them all asynchronously the processing time will just be 100 milliseconds—an order of magnitude difference for the client that made the initial request (Figure 1-6).
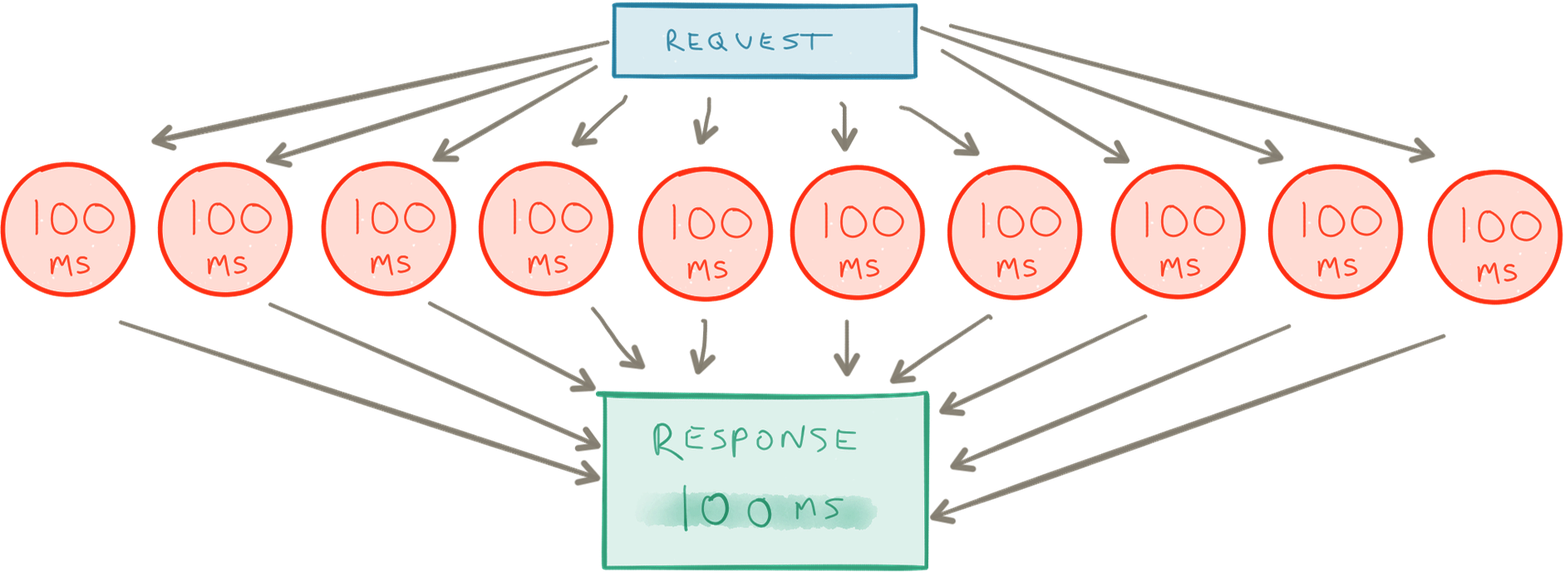
But why is blocking so bad?
It’s best illustrated with an example. If a service makes a blocking call to another service—waiting for the result to be returned—it holds the underlying thread hostage. This means no useful work can be done by the thread during this period. Threads are a scarce resource and need to be used as efficiently as possible. If the service instead performs the call in an asynchronous and non-blocking fashion, it frees up the underlying thread to be used by someone else while waiting for the result to be returned. This leads to much more efficient usage—in terms of cost, energy and performance—of the underlying resources (Figure 1-7).
It is also worth pointing out that embracing asynchronicity is as important when communicating with different resources within a service boundary as it is between services. In order to reap the full benefits of non-blocking execution all parts in a request chain needs to participate—from the request dispatch, through the service implementation, down to the database and back.
Asynchronous message-passing helps making the constraints—in particular the failure scenarios—of network programming first-class, instead of hiding them behind a leaky abstraction14 and pretending that they don’t exist—as seen in the fallacies15 of synchronous Remote Procedure Calls (RPC).
Another benefit of asynchronous message-passing is that it tends to shift focus to the workflow and communication patterns in the application and helps you think in terms of collaboration—how data flows between the different services, their protocols, and interaction patterns.
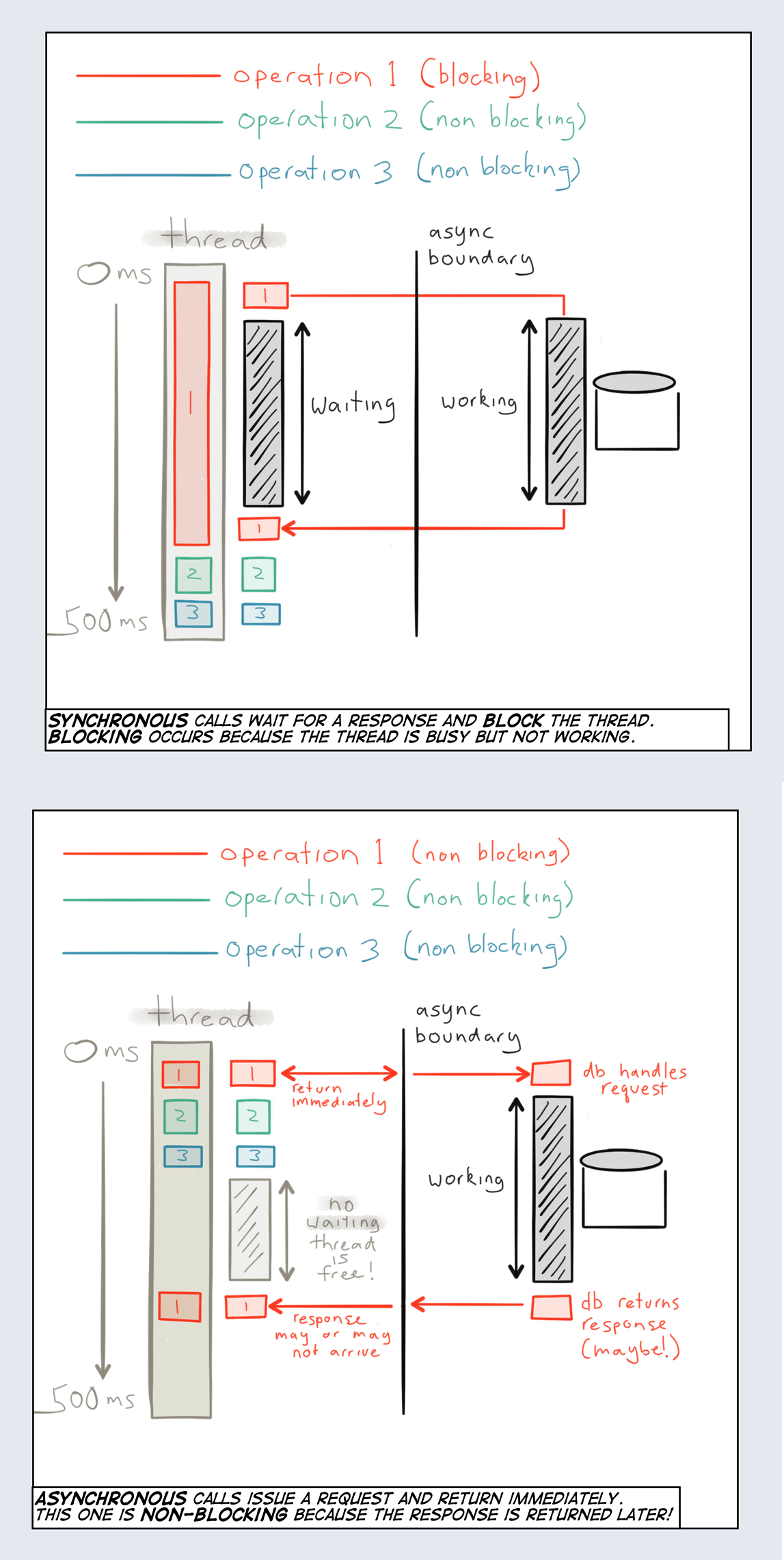
It is unfortunate that REST is widely considered as the default Microservice communication protocol. It’s important to understand that REST is most often synchronous16 which makes it a very unfitting default protocol for inter-service communication. REST might be a reasonable option when there will only ever be a handful of services, or in situations between specific tightly coupled services. But use it sparingly, outside the regular request/response cycle, knowing that it is always at the expense of decoupling, system evolution, scale and availability.17
The need for asynchronous message-passing does not only include responding to individual messages or requests, but also to continuous streams of messages, potentially unbounded streams. Over the past few years the streaming landscape has exploded in terms of both products and definitions of what streaming really means.18
The fundamental shift is that we’ve moved from “data at rest” to “data in motion.” The data used to be offline and now it’s online. Applications today need to react to changes in data in close to real time—when it happens—to perform continuous queries or aggregations of inbound data and feed it—in real time—back into the application to affect the way it is operating.
The first wave of big data was “data at rest.” We stored massive amounts in HDFS or similar and then had offline batch processes crunching the data over night, often with hours of latency.
In the second wave, we saw that the need to react in real time to the “data in motion”—to capture the live data, process it, and feed the result back into the running system within seconds and sometimes even subseconds response time—had become increasingly important.
This need instigated hybrid architectures such as the Lambda Architecture, which had two layers: the “speed layer” for real-time online processing and the “batch layer” for more comprehensive offline processing; this is where the result from the real-time processing in the “speed layer” was later merged with the “batch layer.” This model solved some of the immediate need for reacting quickly to (at least a subset of) the data. But it added needless complexity with the maintenance of two independent models and data processing pipelines, as well as a data merge in the end.
The third wave—that we have already started to see happening—is to fully embrace “data in motion” and for most use cases and data sizes, move away from the traditional batch-oriented architecture altogether towards pure stream processing architecture.
This is the model that is most interesting to Microservices-based architectures because it gives us a way to bring the power of streaming and “data in motion” into the services themselves—both as a communication protocol as well as a persistence solution (through Event Logging, as discussed in the previous section)—including both client-to-service and service-to-service communication.
Stay Mobile, but Addressable
To move, to breathe, to fly, to float,
To gain all while you give,
To roam the roads of lands remote,
To travel is to live.H.C. Andersen
With the advent of cloud computing, virtualization, and Docker containers, we have a lot of power at our disposal to efficiently manage hardware resources. The problem is that none of this matters if our Microservices and its underlying platform cannot make efficient use of it. What we need are services that are mobile, allowing them to be elastic.
We have talked about asynchronous message-passing, and that it provides decoupling in time and space. The latter, decoupling in space, is what we call Location Transparency,19 the ability to, at runtime, dynamically scale the Microservice—either on multiple cores or on multiple nodes—without changing the code. This is service distribution that enables elasticity and mobility; it is needed to take full advantage of cloud computing and its pay-as-you-go models.
For a service to become location transparent it needs to be addressable. But what does that really mean?
First, addresses need to be stable in the sense that they can be used to refer to the service indefinitely, regardless of where it is currently located. This should hold true if the service is running, has been stopped, is suspended, is being upgraded, has crashed, and so on. The address should always work (Figure 1-8). This means a client can always send messages to an address. In practice they might sometimes be queued up, resubmitted, delegated, logged, or sent to a dead letter queue.
Second, an address needs to be virtual in the sense that it can, and often does, represent not just one, but a whole set of runtime instances that together defines the service. Reasons this can be advantageous include:
- Load-balancing between instances of a stateless service
- If a service is stateless then it does not matter to which instance a particular request is sent and a wide variety of routing algorithms can be employed, such as round-robin, broadcast or metrics-based.
- Active-Passive20 state replication between instances of a stateful service
- If a service is stateful then sticky routing needs to be used—sending every request to a particular instance. This scheme also requires each state change to be made available to the passive instances of the service—the replicas—each one ready to take over serving the requests in case of failover.
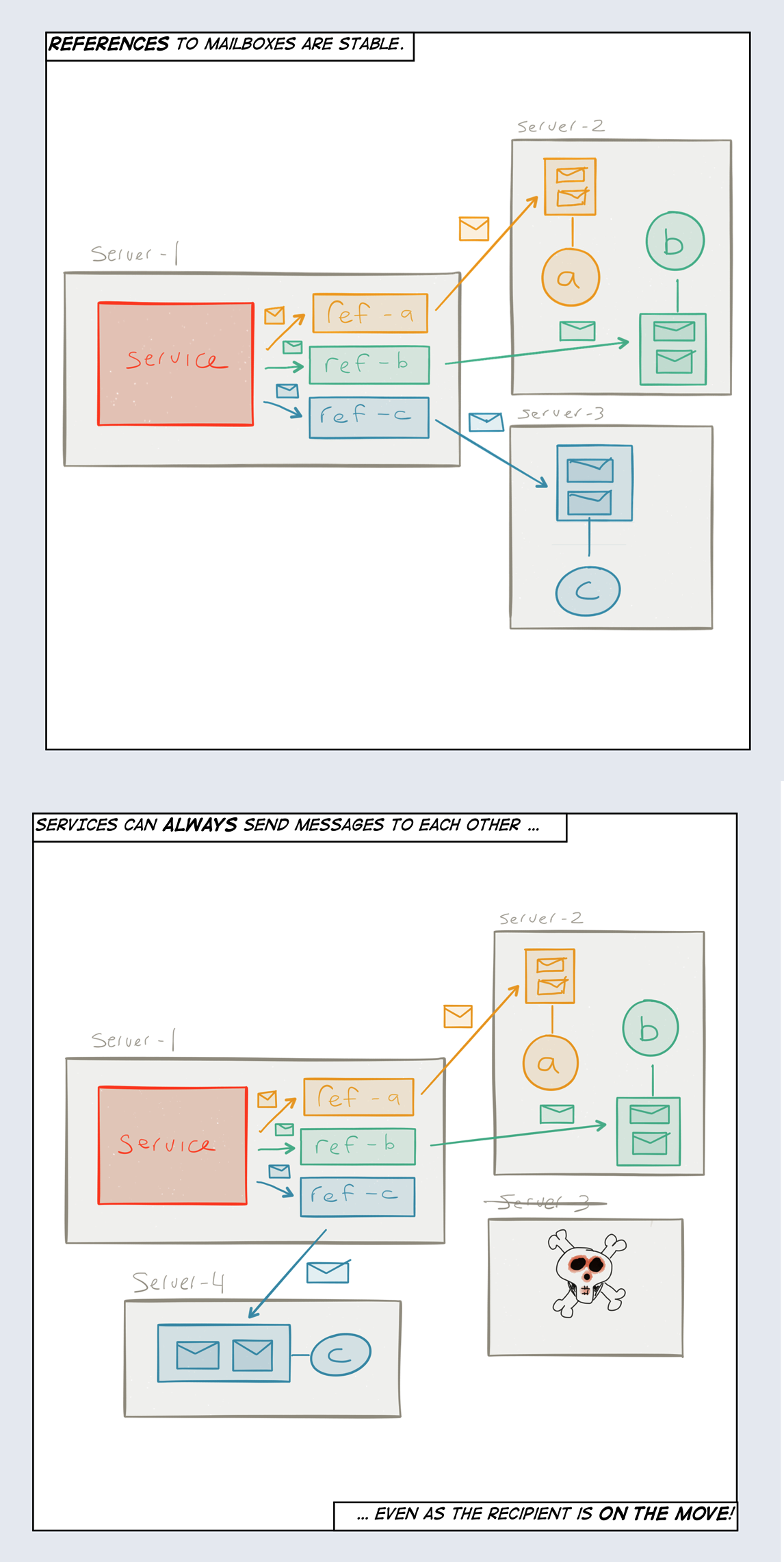
- Relocation of a stateful service
- It can be beneficial to move a service instance from one location to another in order to improve locality of reference21 or resource efficiency.
Using virtual addresses means that the client can stay oblivious to all of these low-level runtime concerns—it communicates with a service through an address and does not have to care about how and where the service is currently configured to operate.
1For an insightful discussion on the problems caused by a mutable state, see John Backus’ classic Turing Award Lecture “Can Programming Be Liberated from the von Neumann Style?”
2Neil Gunter’s Universal Scalability Law is an essential tool in understanding the effects of contention and coordination in concurrent and distributed systems.
3For a discussion on the use of bulkheads in ship construction, see the Wikipedia page https://en.wikipedia.org/wiki/Bulkhead_(partition).
4For an in-depth analysis of what made Titanic sink see the article “Causes and Effects of the Rapid Sinking of the Titanic.”
5Process (service) supervision is a construct for managing failure used in Actor languages (like Erlang) and libraries (like Akka). Supervisor hierarchies is a pattern where the processes (or actors/services) are organized in a hierarchical fashion where the parent process is supervising its subordinates. For a detailed discussion on this pattern see “Supervision and Monitoring.”
6Our definition of a promise is taken from the chapter “Promise Theory” from Thinking in Promises by Mark Burgess (O’Reilly), which is a very helpful tool in modeling and understanding reality in decentralized and collaborative systems. It shows us that by letting go and embracing uncertainty we get on the path towards greater certainty.
7The Unix philosophy is captured really well in the classic book The Art of Unix Programming by Eric Steven Raymond (Pearson Education, Inc.).
8For an in-depth discussion on the Single Responsibility Principle see Robert C. Martin’s website “The Principles of Object Oriented Design.”
9Visit Martin Fowler’s website For more information on how to use the Bounded Context and Ubiquitous Language modeling tools.
10Domain-Driven Design (DDD) was introduced by Eric Evans in his book Domain-Driven Design: Tackling Complexity in the Heart of Software (Addison-Wesley Professional).
11See Jay Kreps’ epic article “The Log: What every software engineer should know about real-time data’s unifying abstraction.”
12Martin Fowler has done a couple of good write-ups on Event Sourcing and CQRS.
13The quote is taken from Pat Helland’s insightful paper “Immutability Changes Everything.”
14As brilliantly explained by Joel Spolsky in his classic piece “The Law of Leaky Abstractions.”
15The fallacies of RPC has not been better explained than in Steve Vinoski’s “Convenience over Correctness.”
16Nothing in the idea of REST itself requires synchronous communication, but it is almost exclusively used that way in the industry.
17See the Integration section in Chapter 3 for a discussion on how to interface with clients that assumes a synchronous protocol.
18We are using Tyler Akidau’s definition of streaming, “A type of data processing engine that is designed with infinite data sets in mind” from his article “The world beyond batch: Streaming 101.”
19Location Transparency is an extremely important but very often ignored and under-appreciated principle. The best definition of it can be found in the glossary of the Reactive Manifesto—which also puts it in context: http://www.reactivemanifesto.org/glossary#Location-Transparency.
20Sometimes referred to as “Master-Slave,” “Executor-Worker,” or “Master-Minion” replication.
21Locality of Reference is an important technique in building highly performant systems. There are two types of reference locality: temporal, reuse of specific data; and spatial, keeping data relatively close in space. It is important to understand and optimize for both.