Where should you put your data scientists?
Stand-alone, embedded, or integrated teams? It depends on what you value.
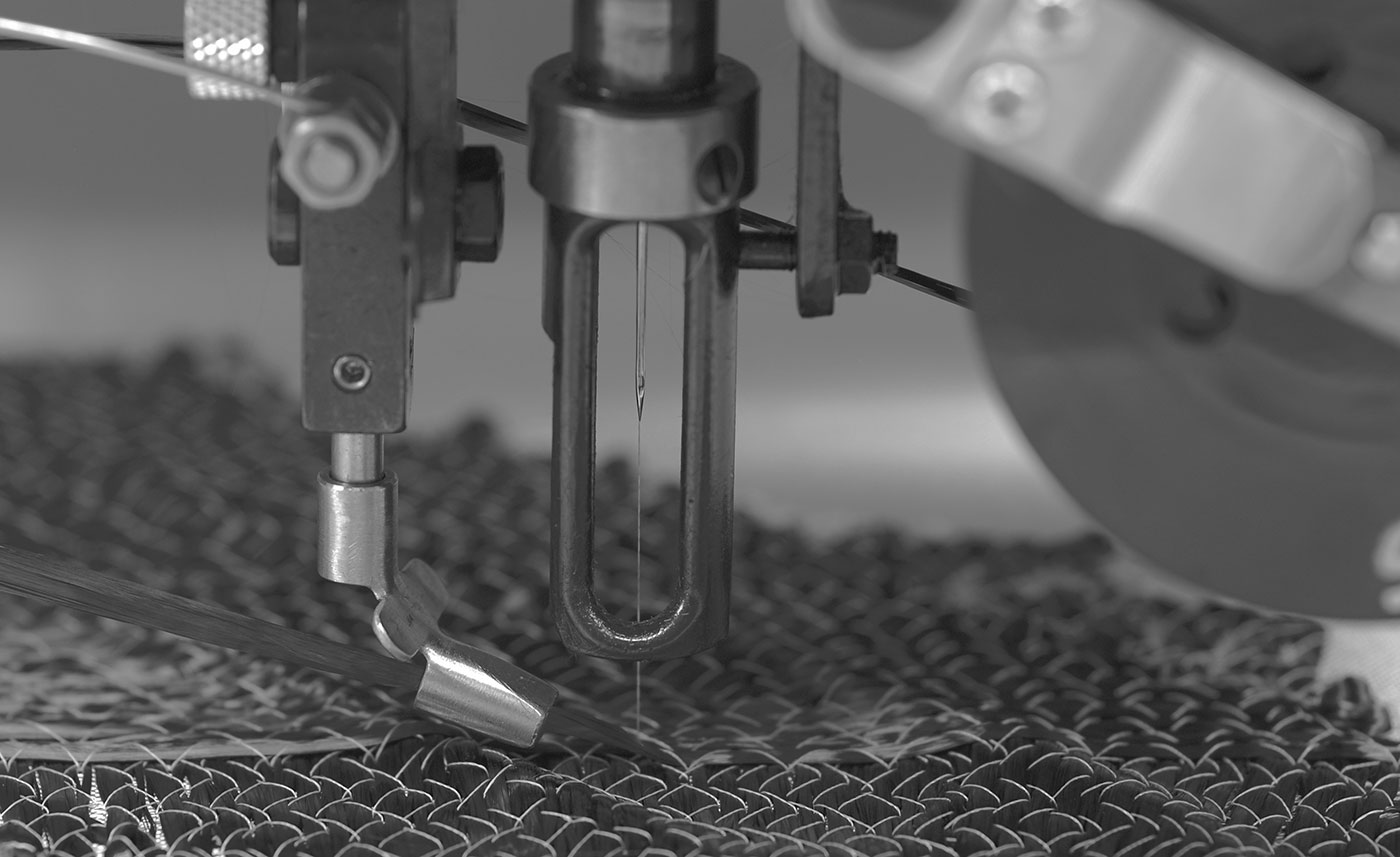
Editor’s note: This is the third in a three-part series of posts by Daniel Tunkelang dedicated to data science as a profession. In this series, Tunkelang covers the recruiting, essential functions, and organization of data science teams.
It’s hard to recruit data scientists. But once you have them, where should you put them? What is the best way to unleash their value? Every org structure has trade-offs. Let’s walk through a few possibilities and explore their pros and cons.
Stand-alone data science teams
LinkedIn and Facebook, companies that pioneered “data scientist” as a job description, established stand-alone data science teams. In this org structure, data science acts an autonomous unit, parallel to engineering. There is a head of data science who reports to a product or technical executive—or directly to the CEO.
The main advantage of the stand-alone model is the autonomy it grants to data scientists. Since data science has broad applicability across the company, the team can apply its talents to whatever problems it deems most valuable. Making data science a top-level organization also has the symbolic benefit of demonstrating that the company sees data as a first-class asset. This symbolism helps companies attract world-class data scientist leadership and enables them to assemble highly talented teams.
But this autonomy comes with a price: stand-alone data science teams risk marginalization. In many companies, engineering teams cherish their autonomy. Even when they could benefit from collaboration with data scientists, they don’t want to depend on resources they don’t control. In some cases, those teams hire their own data scientists, perhaps hiding them by using inconspicuous job titles, such as “research engineer.” In the worst case, the stand-alone data science team becomes an orphan at the organization’s periphery.
Embedded data science teams
The antithesis of the stand-alone team is an embedded model, where the data science team brings in talented people and farms them out to the rest of the company. There’s still a head of data science, but he or she acts primarily as a hiring manager. The embedded model is quite popular—in my experience, it is the most common model among companies that have data science teams.
The embedded model addresses the key weakness of the stand-alone team: embedding data scientists throughout the company ensures utilization. Indeed, product managers create a queue of projects for data scientists, and thus have a vested interest in the data scientists’ success. Best of all, the embedded model allows product managers to assign data science tasks to the people most qualified to work on them.
Unfortunately, the embedded model takes away the autonomy of the data science team, causing it to become less of a team and more of a body shop. Data scientists work on the tasks assigned to them by the teams in which they’re embedded. In addition, there’s a risk that data scientists have second-class status as embedded team members and miss opportunities to work on the team’s most exciting projects. For this reason, the embedded model turns off some of the most talented data scientists as well as the most talented data science leaders. One way to address this risk is to embed data science managers along with the data scientists, but that approach only works at a large enough scale.
Integrated data scientists
If we can’t accept the drawbacks of stand-alone and embedded data science teams, is there another alternative? A radically different approach is to not have a data scientist team at all, but rather to integrate data scientists into the teams that need them. The head of data science, if there is one, is an architect rather than a manager. Product teams hire and manage their own data scientists.
I’m partial to this approach, and it’s the way I ran the Query Understanding team at LinkedIn. It optimizes for organizational alignment and makes data scientists first-class members of their teams. Like magic, integration address the biggest problems with stand-alone and embedded teams. An integrated data scientist has as much opportunity as any other team member to work on the team’s most exciting projects. Within the team’s scope, a data scientist’s ability to contribute is only limited by his or her skills—and a supportive team environment is a great place to learn new skills. In short, the success of integrated data scientists is aligned with that of their teams.
But magic always comes with a price. Integrated data scientists lack the autonomy and visibility they would have in a stand-alone team, and the head of data science (if there is one) risks being a figurehead rather than a true leader. Indeed, the leader of an integrated team needs to be someone who can effectively manage both engineers and data scientists. In addition, integrating data scientists into established teams is a less flexible approach than embedding them on an as-needed basis. Finally, the lack of a core data science team in an organization can create challenges around hiring, knowledge sharing, and career development. Specifically, if data scientists are a minority within an organization dominated by engineers, there’s a risk that they’ll get the short end of the cultural stick.
Conclusion
So, which approach should you use? To steal a phrase from George Box: all organizational models are wrong, but some models are more useful than others. I prefer the integrated approach, because I feel that the benefits of organizational alignment outweigh all other considerations.
But every organization has to decide its own trade-offs. For some, the benefits of an autonomous stand-alone team outweigh the risk of that team being marginalized. For others, the organizational alignment of an integrated team doesn’t justify the challenges that model creates around hiring and culture.
It’s up to you to pick the model that works best for your company. Finally, remember that org structure is important, but what matters most is the people you hire and the culture you create around them. So, hire great people and give them the opportunity to do great things!