How AI is used to infer human emotion
Rana el Kaliouby discusses the techniques, possibilities, and challenges around emotion AI today.
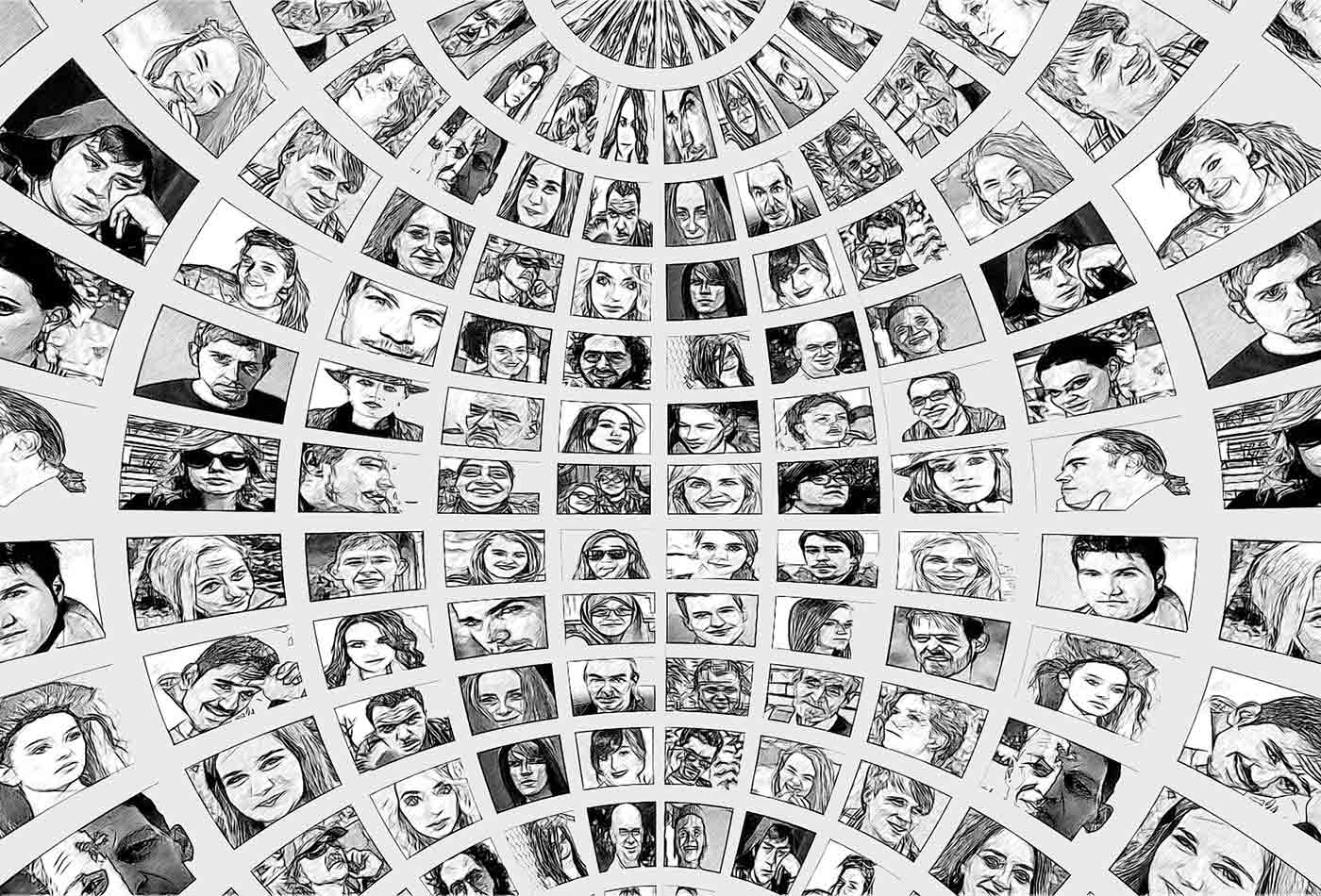
Rana el Kaliouby is the co-founder and CEO of Affectiva, an emotion measurement technology company that grew out of MIT’s Media Lab. Rana is giving a talk, The science and applications of the emerging field of artificial emotional intelligence, at the Artificial Intelligence Conference in New York City on June 28, 2017. We recently caught up with Rana to discuss the techniques, possibilities, and challenges around emotion AI today. Our conversation has been edited for clarity.
For those less familiar with emotion AI, can you describe what the field encompasses?
Emotion AI is the idea that devices should sense and adapt to emotions like humans do. This can be done in a variety of ways—understanding changes in facial expressions, gestures, physiology, and speech. Our relationship with technology is changing, as it’s becoming a lot more conversational and relational. If we are trying to build technology to communicate with people, that technology should have emotional intelligence (EQ). This manifests in a broad range of applications: from Siri on your phone to social robots, even applications in your car.
How is emotion AI related to sentiment analysis for natural language processing?
Social scientists who have studied how people portray emotions in conversation found that only 7-10% of the emotional meaning of a message is conveyed through the words. We can mine Twitter, for example, on text sentiment, but that only gets us so far. About 35-40% is conveyed in tone of voice—how you say something—and the remaining 50-60% is read through facial expressions and gestures you make. Technology that reads your emotional state, for example by combining facial and voice expressions, represents the emotion AI space. They are the subconscious, natural way we communicate emotion, which is nonverbal and which complements our language. What we say is also very cognitive—we have to think about what we are going to say. Facial expressions and speech actually deal more with the subconscious, and are more unbiased and unfiltered expressions of emotion.
What techniques and training data do machines use to perceive emotion?
At Affectiva, we use a variety of computer vision and machine learning approaches, including deep learning. Our technology, like many computer vision approaches, relies on machine learning techniques in which algorithms learn from examples (training data). Rather than encoding specific rules that depict when a person is making a specific expression, we instead focus our attention on building intelligent algorithms that can be trained to recognize expressions.
Through our partnerships across the globe, we have amassed an enormous emotional database from people driving cars, watching media content, etc. A portion of the data is then passed on to our labeling team, who are certified in the Facial Action Coding System (FACS). Their day-to-day job is to take video from a repository and label it as training data for the algorithms. We are continuously investing in approaches such as active learning (human-assisted machine learning) and transfer learning (the idea that a model-specific modality or data set can then transfer to a different data set, so a video analyzed for facial expression can also be labeled for the speech modality).
Where is emotion AI currently seeing the most market traction?
We got our start in ad content testing applications. We work with a third of the Fortune 500 companies, helping them to understand consumers’ emotional responses to their ads. The problem we were able to solve was to assist them in developing deep emotional content with their customers, then help them understand if that content was successful or not. The recent Pepsi ad is a great example of how emotion AI may be used—they created this ad, and it caused a huge backlash. Think of how much incentive there was to find this out earlier in order to intervene; they could have pulled the campaign, edited, and re-shot before the PR disaster. Our technology provides a moment-by-moment readout of the viewers’ emotional journey to online ads, a tv show, or a movie trailer. We recently released the capability to test a full-length movie.
More recently, we have seen the most traction around conversational interfaces. There are the obvious devices—Amazon’s Alexa or Google Home—but we’ve also seen an increasing need for emotion AI manifesting in automotive, social robotics, and the Internet of Things (IoT). The idea is to better connect with these devices so they can more efficiently do things for you. We are in the proof-of-concept phase for many of these markets and are actively figuring out platform integrations.
As Affectiva has grown from a research project at MIT to a company, what has been most surprising to you?
When we first started as company, I underestimated the value of the data we collected—it has a number of dimensions. Affectiva’s emotion database has grown to nearly six million faces analyzed in 75 countries. To be precise, we have gathered 5,313,751 face videos, for a total of 38,944 hours of data, representing nearly two billion facial frames analyzed. Our data is also global, spanning ages and ethnicities, in a variety of contexts (from people sitting on their couches to driving a car).
The wealth of data we collected also allowed us to mine our robust data set for cross-cultural and gender differences in expressing emotions. We recently released findings from the largest cross-cultural study on gender differences in facial expressions, which used our technology. In this paper, for example, we found that women smile more and longer than men, while men show expressions that are indicative of anger more frequently and longer than women.
Lastly, this data gives us unique business insights around how emotions in ads vary across the world and by product category. For example, we’ve seen that pet care and baby ads in the U.S. elicit more enjoyment than cereal ads—which see the most enjoyment in Canada.
Your Ph.D. is in computer science. Are there any specialized skills you needed to learn to enable computers to recognize human expressions?
Experience in machine learning was key, as was computer vision, pattern recognition, and just being really curious. I had no degree in psychology, but had to brush up on the basics of psych, the science of emotion, and neuroscience to really understand how humans and the human brain process emotion. We borrow a lot of that science and embed it into our technology.
Tell us about the use case for emotion AI that has been personally most compelling or fun for you to see.
Personally, the most compelling use case for me tackles mental health applications: the capabilities here are quite powerful. Early on, we partnered with a company called Brain Power to bring use cases to market. They use our technology and Google Glass to help autistic children with emotion recognition and understanding, an area of identified need in many children and adults with autism. Outside of that use case, I am particularly interested in applications that monitor mental health for emotional well-being and suicide prevention. In our current world of the quantified self—featuring fitness data from wearables and fitness apps—what if your emotional health played a role as well? We are exploring product concepts that will be able to do just that, so users can better monitor their emotional health and well-being around the clock, and can flag when they are having a bad day.