How You Spend Your Time
ANOTHER SET OF QUESTIONS on the survey asked for the approximate amount of hours spent on certain tasks, such as data cleansing, ETL, and machine learning. For managers, directors, VPs, and executives (even at small companies), the task breakdown is very different, as we would expect: fewer technical tasks, more meetings. Removing their responses gives us a general idea of how people spend their time in the data space.
Even among non-managers, it appears that the more time spent in meetings, the more a data scientist (/analyst/engineer) earns. About half of the respondents report spending at least one hour per day on average in a meeting, with 12% spending at least four hours per day in meetings. This pattern is confirmed when we add the task features to the salary model.
Among technical tasks, basic exploratory analysis occupies more time than any other, with 46% of the sample spending one to three hours per day on this task and 12% spending four hours or more. After this, data cleaning eats up the most hours: 39% spend at least one hour per day cleaning data.
To put these hour figures into context, it may help to know the length of the entire work week. Most (75%) of respondents work between 40 and 50 hours per week, with the remaining 25% split evenly between those who work fewer than 40 and more that 50 hours per week. Working longer hours does, in fact, correspond to higher salary.
A final variable will be introduced for the second salary model: bargaining skills. While not exactly an objective rubric, the one-to-five scale (“poor” to “excellent”) is a simple way of estimating an incontrovertibly valuable skill. The distribution of answers was symmetric, with 40% choosing the middling “3” and 8% each choosing the extreme values of “1” and “5.”
A Revised Model, Including Tasks
With the new features on top of the ones used previously, we create a new model. This time, however, we restrict the pool of respondents further: not only do we take out (full-time) students, but professors, managers, and upper management as well. This second model has an R2 of 0.408:
TASK COUNTS
Percentages are taken from non-managers
(i.e., mostly data scientists, analysts, engineers, programmers, architects)
TIME SPENT ON ETL
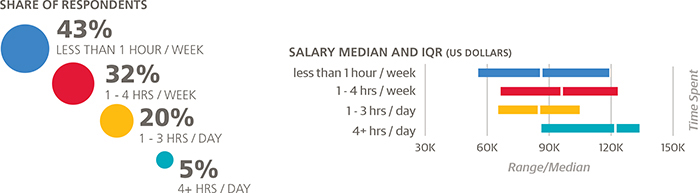
TIME SPENT ON DATA CLEANING
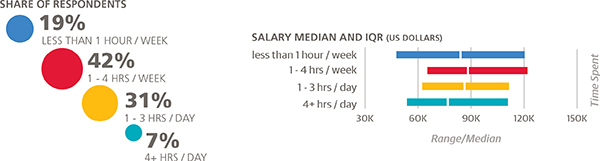
TIME SPENT ON BASIC EXPLORATORY DATA ANALYSIS
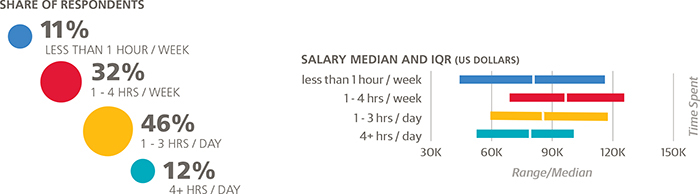
TIME SPENT ON MACHINE LEARNING, STATISTICS
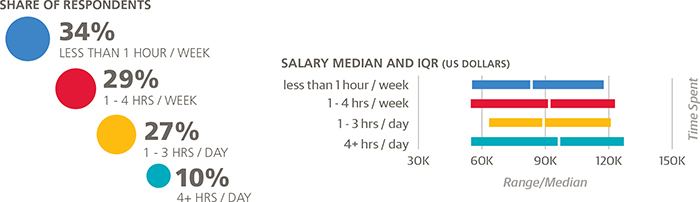
14595 intercept
+1449 age (per year of age above 18)
+7205 bargaining skills (times 1 for "poor" skills
to 5 for "excellent" skills)
+663 work_week (times # hours in week, e.g., 40
hours = $26,520)
-4207 gender=Female
+6593 industry=Software (incl. security, cloud services)
-7696 industry=Education
+1787 company size: 2500+
+13429 PhD
+3496 master's degree (but no PhD)
+2991 academic specialty in computer science
+17264 California
+9511 Northeast US
+1752 Southern US
-1623 Canada
-3073 UK/Ireland
-20139 Europe (except UK/I)
-24026 Latin America
-27823 Asia
+9416 Meetings: 1 - 3 hours / day
+11282 Meetings: 4+ hours / day
+4652 Basic exploratory data analysis: 1 - 4 hours
/ week
-6609 Basic exploratory data analysis: 4+ hours / day
-1273 Creating visualizations: 1 - 3 hours / day
-2241 Creating visualizations: 4+ hours / day
+130 Data cleaning: 1 - 4 hours / week
+1733 Machine learning, statistics: 1 - 3 hours / day
Geography
As we reduce the sample under consideration and add new features, some of the old features change or even drop out, as is the case with “company size < 500”. Changes are apparent in the geographic variables: the penalty for Europe is reduced, coefficients for UK/Ireland and the Southern US appear, and the California boost grows even more, to $17,000.
The intercept has been transformed to $14,595, but this is because we now add $663 per hour in our work week and $7,205 per bargaining skill “point” (1 to 5). So with a 40-hour work week and middling bargaining skills (i.e., a “3”), a 38-year-old man from the US Midwest would begin the calculation of base salary at $91,710.
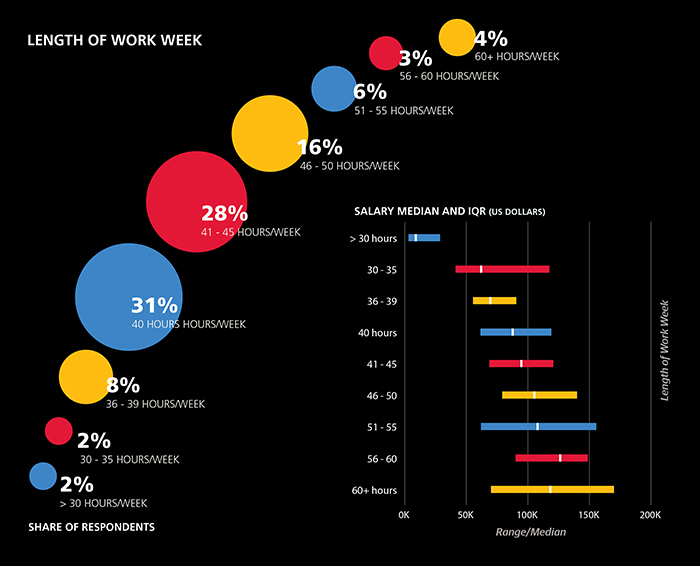
TIME SPENT ON CREATING VISUALIZATIONS
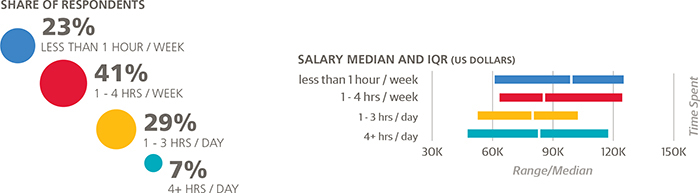
TIME SPENT ON PRESENTING ANALYSIS
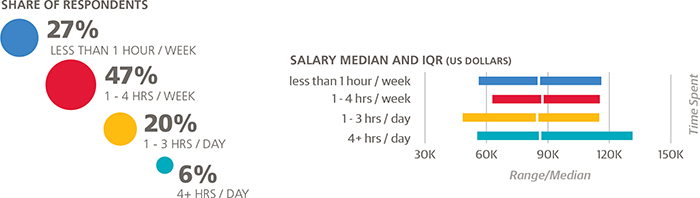
Education
Other changes include a reduction in the “Education” penalty, presumably because we no longer include professors, and a significant boost in the value of a PhD to $13,429. Readers holding a master’s degree should be relieved to learn that, unlike the first, basic model, the second one does not ignore their degree and places a respectable value on it of $3,496. Computer science (as an academic specialty) appears as a feature in this model with a coefficient of $2,991.
Gender
The coefficient for women has decreased in magnitude, although this is largely because of the correlation between gender and certain features that heavily influence salary, and does not really constitute an “improvement” on the picture painted by the first model. For example, 37% of women reported below-average bargaining skills (a score of 1 or 2), while the corresponding figure for men was only 25%.
Time spent on tasks
The estimated effect on salary of various tasks in various time quantities was slightly different than what might be expected by looking at the median salaries of those respondents who spent a certain amount of time on the tasks. For example, the median salary of respondents who spend at least four hours per day on ETL was an impressive $123,000, but no variable for ETL proved significant in the model.
As mentioned above, more meeting time emphatically correlates with higher salary, even among non-managers. According to the model, spending over four hours per day on any one technical task never increases expected salary. In the case of basic exploratory analysis and creating visualizations, spending half of each day on these tasks decreases expected salary by $6,609 and $2,241, respectively. Interestingly, spending one to fourhours per week on basic exploratory analysis is the sweet spot for this task, boosting expected salary by $4,652.
Machine learning/statistics appears to be the only technical task for which a commitment of greater than one hour per day is rewarded in the model (not penalized or ignored): spending one to three hours per day on machine learning raises expected salary by $1,733.
Spending one to four hours per week on basic exploratory analysis is the sweet spot for this task, boosting expected salary by $4,652.
BARGAINING SKILLS SELF-EVALUATION FROM 1-5 (1 Being Poor, 5 Being Excellent)
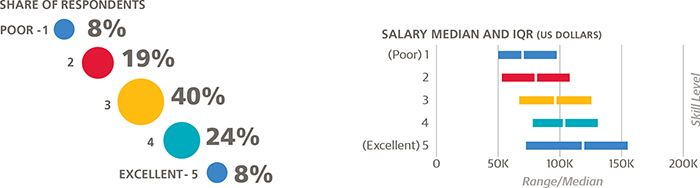
TIME SPENT ON MEETINGS
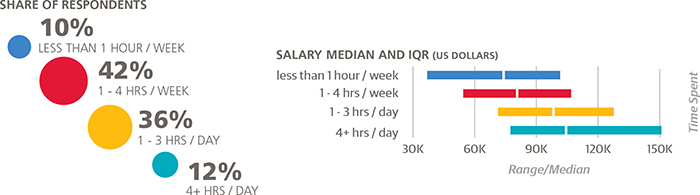
Get 2015 Data Science Salary Survey now with the O’Reilly learning platform.
O’Reilly members experience books, live events, courses curated by job role, and more from O’Reilly and nearly 200 top publishers.