3Battery State of Charge and State of Energy Estimation
3.1 Background
Many techniques have been developed to construct state of charge (SOC) estimators for battery management systems (BMSs) in electric vehicles (EVs) [1]. Due to the closed‐loop estimation ability and strong inhibiting effect on noises, Kalman filter (KF)‐based SOC estimators are studied extensively [2]. H infinity filters (HIFs) are also studied to estimate battery SOC owing to their robustness against disturbances in battery models and noises [3].
Nevertheless, the SOC indicates only capacity state rather than energy state on which the EV driving range is dependent. Therefore, battery state of energy (SOE) estimators, which provide the essential basis and security of energy deployment, have also been developed for BMSs [4] in EVs. This chapter mainly presents model‐based SOC and SOE estimation methods using the extended Kalman filter (EKF) and HIF algorithms.
3.2 Classification
There are four major groups of battery SOC estimation methods, including a look‐up‐table‐based method, an ampere‐hour (Ah) integral method, data‐driven estimation methods, and model‐based estimation methods, as shown in Figure 3.1.
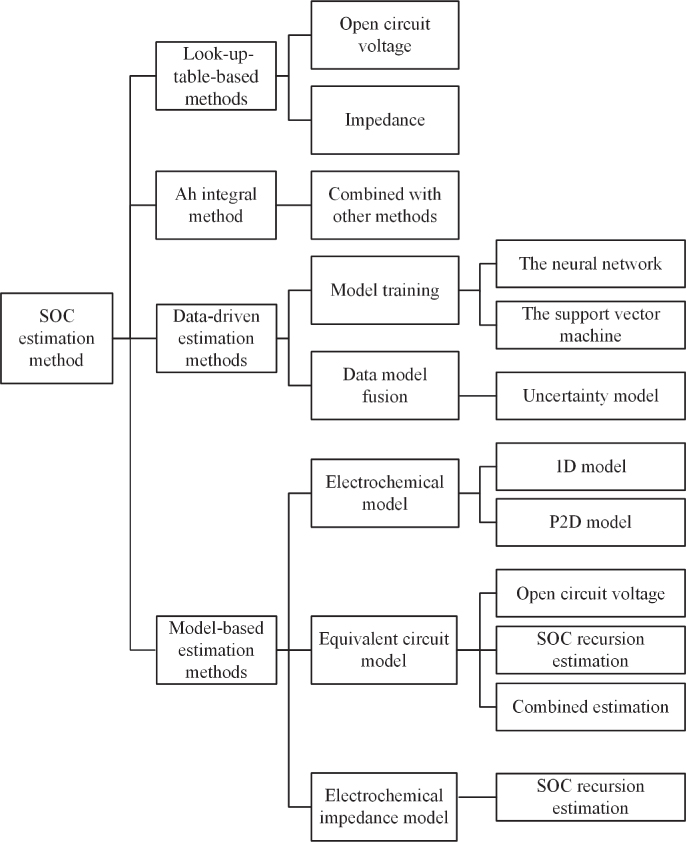
Figure 3.1 Battery SOC estimation methods.
3.2.1 Look‐Up‐Table‐Based Method
Battery SOC is strongly related to external (static) characteristic parameters, such as open circuit voltage (OCV). It can be ...
Get Advanced Battery Management Technologies for Electric Vehicles now with the O’Reilly learning platform.
O’Reilly members experience books, live events, courses curated by job role, and more from O’Reilly and nearly 200 top publishers.