Chapter 1. Data I/O
Events happen all around us, continuously. Occasionally, we make a record of a discrete event at a certain point in time and space. We can then define data as a collection of records that someone (or something) took the time to write down or present in any format imaginable. As data scientists, we work with data in files, databases, web services, and more. Usually, someone has gone through a lot of trouble to define a schema or data model that precisely denotes the names, types, tolerances, and inter-relationships of all the variables. However, it is not always possible to enforce a schema during data acquisition. Real data (even in well-designed databases) often has missing values, misspellings, incorrectly formatted types, duplicate representations for the same value, and the worst: several variables concatenated into one. Although you are probably excited to implement machine-learning algorithms and create stunning graphics, the most important and time-consuming aspect of data science is preparing the data and ensuring its integrity.
What Is Data, Anyway?
Your ultimate goal is to retrieve data from its source, reduce the data via statistical analysis or learning, and then present some kind of knowledge about what was learned, usually in the form of a graph. However, even if your result is a single value such as the total revenue, most engaged user, or a quality factor, you still follow the same protocol: input data → reductive analysis → output data.
Considering that practical data science is driven by business questions, it will be to your advantage to examine this protocol from right to left. First, formalize the question you are trying to answer. For example, do you require a list of top users by region, a prediction of daily revenue for the next week, or a plot of the distribution of similarities between items in inventory? Next, explore the chain of analyses that can answer your questions. Finally, now that you have decided on your approach, exactly what data will you need to accomplish this goal? You may be surprised to find that you do not have the data required. Often you will discover that a much simpler set of analysis tools (than you originally envisioned) will be adequate to achieve the desired output.
In this chapter, you will explore the finer details of reading and
writing data from a variety of sources. It is important to ask yourself
what data model is required for any subsequent steps. Perhaps it will
suffice to build a series of numerical array types (e.g.,
double[][]
, int[]
, String[]
) to
contain the data. On the other hand, you may benefit from creating a
container class to hold each data record, and then populating a
List
or Map
with those objects. Still another
useful data model is to formulate each record as a set of key-value pairs
in a JavaScript Object Notation (JSON) document. The decision of what data
model to choose rests largely on the input requirements of the subsequent
data-consuming processes.
Data Models
What form is the data in, and what form do you need to transform it to so you
can move forward? Suppose somefile.txt contained rows
of id
, year
, and city
data.
Univariate Arrays
The simplest data model for this particular example is to create a
series of arrays for the three variables id
,
year
, and city
:
int
[]
id
=
new
int
[
1024
];
int
[]
year
=
new
int
[
1024
];
String
[]
city
=
new
String
[
1024
];
As the BufferedReader
loops through the lines of the file, values are added to each
position of the arrays with the aid of an incrementing counter. This
data model is probably adequate for clean data of known dimensions,
where all the code ends up in one executable class. It would be fairly
straightforward to feed this data into any number of statistical
analysis or learning algorithms. However, you will probably want to
modularize your code and build classes and subsequent methods suited for
each combination of data source and data model. In that case, shuttling
around arrays will become painful when you have to alter the signatures
of existing methods to accommodate new arguments.
Multivariate Arrays
Here you want each row to hold all the data for a record, but they must be the same type! So in our case, this would work only if you assigned cities a numerical, integer value:
int
[]
row1
=
{
1
,
2014
,
1
};
int
[]
row2
=
{
2
,
2015
,
1
};
int
[]
row3
=
{
3
,
2014
,
2
};
You could also make this a 2D array:
int
[][]
data
=
{{
1
,
2014
,
1
},
{
2
,
2015
,
1
},
{
3
,
2014
,
2
}};
For your first pass through a dataset, there may be a complicated data model already, or just a mixture of text, integers, doubles, and date times. Ideally, after you have worked out what will go into a statistical analysis or learning algorithm, this data is transformed into a two-dimensional array of doubles. However, it takes quite a bit of work to get to that point. On the one hand, it’s convenient to be handed a matrix of data from which you can forge ahead with machine learning. On the other, you may not know what compromises were made or what mistakes have been propagated, undetected.
Data Objects
Another option is to create a container class and then populate a collection
such as List
or Map
with those containers. The
advantages are that it keeps all the values of a particular record
together, and adding new members to a class will not break any methods
that take the class as an argument. The data in the file
somefile.txt can be represented by the following
class:
class
Record
{
int
id
;
int
year
;
String
city
;
}
Keep the class as lightweight as possible, because a collection
(List
or Map
) of these objects will add up for
a large dataset! Any methods acting on Record
could be
static methods ideally in their own class titled something like
RecordUtils
.
The collection’s structure, List
, is used to hold all the Record
objects:
List
<
Record
>
listOfRecords
=
new
ArrayList
<>();
Looping though the data file with a BufferReader
,
each line can then be parsed and its contents stored in a new
Record
instance. Each new Record
instance is
then added to List<Record> listOfRecords
. Should you
require a key to quickly look up and retrieve an individual
Record
instance, use a Map
:
Map
<
String
,
Record
>
mapOfRecords
=
new
HashMap
<>();
The key to each record should be a unique identifier for that particular record, such as a record ID or URL.
Matrices and Vectors
Matrices and vectors are
higher-level data structures composed of, respectively,
two- and one-dimensional arrays. Usually, a dataset contains multiple
columns and rows, and we can say that these variables form a
two-dimensional array (or matrix) X in which there are rows and
columns. We choose
to be the row index, and
to be the column index, such that each element of
the
matrix is
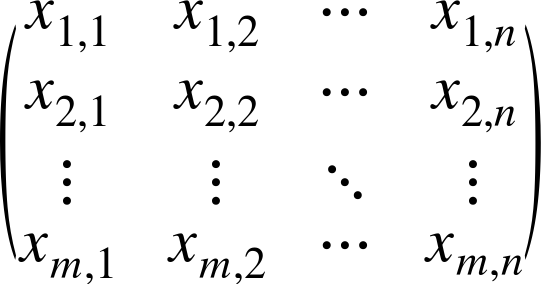
When we put values into a data structure like a matrix, we can gain convenience. In many situations, we will be performing mathematical operations on our data. A matrix instance can have abstract methods for performing these operations, with implementation details that are suited for the task at hand. We will explore matrices and vectors in detail in Chapter 2.
JSON
JavaScript Object Notation (JSON) has
become a prevalent form of representing data. In general,
JSON data is represented by simple rules at
json.org: double quotes! No trailing commas! A JSON
object has outer curly braces and can have any valid set of key-value
pairs separated by commas (the order of contents is not guaranteed, so
treat it as a HashMap
type):
{
"city"
:
"San Francisco"
,
"year"
:
2020
,
"id"
:
2
,
"event_codes"
:
[
20
,
22
,
34
,
19
]}
A JSON array has outer square brackets with valid JSON contents
separated by commas (the order of array contents is guaranteed, so treat
it as an ArrayList
type):
[
40
,
50
,
70
,
"text"
,
{
"city"
:
"San Francisco"
}]
There are two main categories you will find. Some data files contain complete JSON objects or arrays. These are usually configuration files. However, another type of data structure that is common is a text file of independent JSON objects, one per line. Note that this type of data structure (list of JSONs) is technically not a JSON object or array because there are no closing braces or commas between lines, and as such, trying to parse the whole data structure as one JSON object (or array) will fail.
Dealing with Real Data
Real data is messy, incomplete, incorrect, and sometimes incoherent. If you are working with a “perfect” dataset, it’s because someone else spent a great deal of time and effort in getting it that way. It is also possible that your data is, in fact, not perfect, and you are unwittingly performing analyses on junk data. The only way to be sure is to get data from the source and process it yourself. This way, if there is a mistake, you know who to blame.
Nulls
Null values appear in a variety of forms. If the data is being passed
around inside Java, it’s entirely possible to have a null
.
If you are parsing strings from a text file, a null
value
may be represented by a variety of the literal string
"null"
, "NULL"
, or other string such as
"na"
, or even a dot. In either case (a null type or null
literal), we want to keep track of these:
private
boolean
checkNull
(
String
value
)
{
return
value
==
null
||
"null"
.
equalsIgnoreCase
(
value
);
}
Often a null value has been recorded as a blank space or series of blank spaces. Although this is sometimes a nuisance, it may serve a purpose, because encoding a 0 is not always appropriate to represent the concept that the data point does not exist. For example, if we were tracking binary variables, 0 and 1, and came across an item for which we did not know the value, then wrongly assigning 0 to the value (and writing it to the file) would incorrectly assign a true negative value. When writing a null value to a text file, my preference is for a zero-length string.
Blank Spaces
Blank spaces abound in real data. It is straightforward to check for an
empty string by using the String.isEmpty()
method. However, keep in
mind that a string of blank spaces (even one blank space) is not empty!
First, we use the String.trim()
method to remove any leading or trailing spaces around the input
value and then check its length. String.isEmpty()
returns
true
only if the string has zero length:
private
boolean
checkBlank
(
String
value
)
{
return
value
.
trim
().
isEmpty
();
}
Parse Errors
Once we know the string value is neither null nor blank, we parse it into the type we require. We’ll leave the parsing of strings to strings out of this, because there is nothing to parse!
When dealing with numeric types, it is unwise to cast strings to a
primitive type such as double
, int
, or
long
. It is recommended to use the object wrapper classes
such as Double
, Integer
, and
Long
, which have a string-parsing method that throws a NumberFormatException
should
something go wrong. We can catch that exception and update a parsing
error counter. You can also print or log the error:
try
{
double
d
=
Double
.
parseDouble
(
value
);
// handle d
}
catch
(
NumberFormatException
e
)
{
// increment parse error counter etc.
}
Similarly, date times formatted as a string can be parsed by
the OffsetDateTime.parse()
method; the
DateTimeParseException
can be caught and logged should something be wrong with the input
string:
try
{
OffsetDateTime
odt
=
OffsetDateTime
.
parse
(
value
);
// handle odt
}
catch
(
DateTimeParseException
e
)
{
// increment parse error counter etc.
}
Outliers
Now that our data is cleaned and parsed, we can check whether the value is acceptable given our requirements. If we were expecting a value of either 0 or 1 and we get a 2, the value is clearly out of range and we can designate this data point as an outlier. As in the case with nulls and blanks, we can perform a Boolean test on the value to determine whether it is within an acceptable range of values. This is good for numeric types as well as strings and date times.
In the case of checking ranges with numeric types, we need to know the
minimum and maximum acceptable values and whether they are inclusive or
exclusive. For example, if we set minValue = 1.0
and
minValueInclusive = true
, all values greater than or equal
to 1.0 will pass the test. If we set minValueInclusive =
false
, only values greater than 1.0 will pass the test. Here is
the code:
public
boolean
checkRange
(
double
value
)
{
boolean
minBit
=
(
minValueInclusive
)
?
value
>=
minValue
:
value
>
minValue
;
boolean
maxBit
=
(
maxValueInclusive
)
?
value
<=
maxValue
:
value
<
maxValue
;
return
minBit
&&
maxBit
;
}
Similar methods can be written for integer types.
We can also check whether a string value is in an acceptable range
by setting an enumeration of valid strings. This can be done by creating
a Set
instance of valid strings called, for example,
validItems
, where the Set.contains()
method
can be used to test the validity of an input value:
private
boolean
checkRange
(
String
value
)
{
return
validItems
.
contains
(
value
);
}
For DateTime
objects, we can check whether a date is
after a minimum date and before a maximum date. In this case, we define
the min and max as OffsetDateTime
objects and then test
whether the input date time is between the min and max. Note that OffsetDateTime.isBefore()
and
OffsetDateTime.isAfter()
are exclusive. If the input date time is equal to either the
min or max, the test will fail. Here is the code:
private
boolean
checkRange
(
OffsetDateTime
odt
)
{
return
odt
.
isAfter
(
minDate
)
&&
odt
.
isBefore
(
maxDate
);
}
Managing Data Files
This is where the art of data science begins! How you choose to build a dataset is not
only a matter of efficiency, but also one of flexibility. There are many
options for reading and writing files. As a bare minimum, the entire
contents of the file can be read into a String
type by using a FileReader
instance, and then the
String
can be parsed into the data model. For large files,
I/O errors are avoided by using a BufferedReader
to read each
line of the file separately. The strategy presented here is to parse each
line as it is read, keeping only the values that are required and
populating a data structure with those records. If there are 1,000
variables per line, and only three are required, there is no need to keep
all of them. Likewise, if the data in a particular line does not meet
certain criteria, there is also no need to keep it. For large datasets,
this conserves resources compared to reading all the lines into a string
array (String[]
) and parsing it later. The more consideration
you put into this step of managing data files, the better off you will be.
Every step you take afterward, whether it’s statistics, learning, or
plotting, will rely on your decisions when building a dataset. The old
adage of “garbage in, garbage out” definitely applies.
Understanding File Contents First
Data files come in a bewildering array of configurations, with some undesirable
features as a result. Recall that ASCII files are just a collection of ASCII characters
printed to each line. There is no guarantee on the format or precision
of a number, the use of single or double quotes, or the inclusion (or
exclusion) of numerous space, null, and newline characters. In short,
despite your assumptions as to the contents of the file, there can be
almost anything on each line. Before reading in the file with Java, take
a look at it in a text editor or with the command line. Note the number,
position, and type of each item in a line. Pay close attention to how
missing or null values are represented. Also note the type of
delimiter and any headers describing the data. If the file is small
enough, you can scan it visually for missing or incorrectly formatted
lines. For example, say we look at the file
somefile.txt with the Unix command
less
in a bash shell:
bash$ less somefile.txt "id","year","city" 1,2015,"San Francisco" 2,2014,"New York" 3,2012,"Los Angeles" ...
We see a comma-separated values (CSV) dataset with the columns
id
, year
, and city
. We can
quickly check the number of lines in the file:
bash$
wc -l somefile.txt
1025
This indicates that there are 1,024 lines of data plus one line more for the header. Other formats are possible, such as tab-separated values (TSV), a “big string” format in which all the values are concatenated together, and JSON. For large files, you may want to take the first 100 or so lines and redirect them to an abridged file for purposes of developing your application:
bash$
head -100 filename > new_filename
In some cases, the data file is just too big for a pair of eyes to scan it for structure or errors. Clearly, you would have trouble examining a data file with 1,000 columns of data! Likewise, you are unlikely to find an error in formatting by scrolling through one million lines of data. In this case it is essential that you have an existing data dictionary that describes the format of the columns and the data types (e.g., integer, float, text) that are expected for each column. You can programmatically check each line of data as you parse the file via Java; exceptions can be thrown, and, perhaps, the entire contents of the offending line printed out so you can examine what went wrong.
Reading from a Text File
The general approach for reading a text file is to create a FileReader
instance surrounded by a BufferedReader
that
enables reading each line. Here, FileReader
takes the argument of String
filename
, but FileReader
can
also take a File
object as its argument. The
File
object is useful when filenames and paths are
dependent on the operating system. This is the generic form for reading
files line by line with a BufferedReader
:
try
(
BufferedReader
br
=
new
BufferedReader
(
new
FileReader
(
"somefile.txt"
))
)
{
String
columnNames
=
br
.
readline
();
// ONLY do this if it exists
String
line
;
while
((
line
=
br
.
readLine
())
!=
null
)
{
/* parse each line */
// TODO
}
}
catch
(
Exception
e
)
{
System
.
err
.
println
(
e
.
getMessage
());
// or log error
}
We can do the exact same thing if the file exists somewhere remotely:
URL
url
=
new
URL
(
"http://storage.example.com/public-data/somefile.txt"
);
try
(
BufferedReader
br
=
new
BufferedReader
(
new
InputStreamReader
(
url
.
openStream
()))
)
{
String
columnNames
=
br
.
readline
();
// ONLY do this if it exists
String
line
;
while
((
line
=
br
.
readLine
())
!=
null
)
{
// TODO parse each line
}
}
catch
(
Exception
e
)
{
System
.
err
.
println
(
e
.
getMessage
());
// or log error
}
We just have to worry about how to parse each line.
Parsing big strings
Consider a file in which each row is a “big string” of concatenated values, and any substring with starting and stopping positions encodes a particular variable:
0001201503 0002201401 0003201202
The first four digits are the id
number, the second
four are the year
, and the last two are the
city
code. Keep in mind that each line can be thousands
of characters long, and the position of character substrings is
critical. It is typical that numbers will be padded with zeros, and
empty spaces may be present for null values. Note that periods
occurring inside a float (e.g., 32.456) count as a space, as will any
other “strange” character! Usually, text strings are encoded as
values. For example, in this case, New York = 01
,
Los Angeles = 02
, and San Francisco =
03
.
In this case, the values from each line can be accessed with the
method String.substring(int beginIndex, int
endIndex)
. Note that the substring starts at
beginIndex
and goes up to (but not including)
endIndex
:
/* parse each line */ int id = Integer.parseInt(line.substring(0, 4)); int year = Integer.parseInt(line.substring(4, 8)); int city = Integer.parseInt(line.substring(8, 10));
Parsing delimited strings
Considering the popularity of spreadsheets and database dumps, it is highly likely you will be given a CSV dataset at some point. Parsing this kind of file could not be easier! Consider the data in our example formatted as a CSV file:
1,2015,"San Francisco" 2,2014,"New York" 3,2012,"Los Angeles"
Then all we need to do is parse with String.split(",")
and utilize
String.trim()
to remove any pesky leading or trailing whitespaces. It also will be necessary to
remove any quotes around strings with String.replace("\"",
"")
:
/* parse each line */
String
[]
s
=
line
.
split
(
","
);
int
id
=
Integer
.
parseInt
(
s
[
0
].
trim
());
int
year
=
Integer
.
parseInt
(
s
[
1
].
trim
());
String
city
=
s
[
2
].
trim
().
replace
(
"\""
,
""
);
In the next example, the data in somefile.txt has been separated by tabs:
1 2015 "San Francisco" 2 2014 "New York" 3 2012 "Los Angeles"
Splitting tab-delimited data is achieved by replacing code for
String.split(",")
in the preceding example with
this:
String
[]
s
=
line
.
split
(
"\t"
);
At some point, you will undoubtedly come across CSV files with fields that contain commas. One example is text taken from a user blog. Yet another example occurs when denormalized data is put into a column—for example, “San Francisco, CA” instead of having separate columns for city and state. This is quite tricky to parse and requires regex. Instead, why not use the Apache Commons CSV parser library?
/* parse each line */
CSVParser
parser
=
CSVParser
.
parse
(
line
,
CSVFormat
.
RFC4180
);
for
(
CSVRecord
cr
:
parser
)
{
int
id
=
cr
.
get
(
1
);
// columns start at 1 not 0 !!!
int
year
=
cr
.
get
(
2
);
String
city
=
cr
.
get
(
3
);
}
The Apache Commons CSV library also handles common formats including CSVFormat.EXCEL, CSVFormat.MYSQL, and CSVFormat.TDF.
Parsing JSON strings
JSON is a protocol for serializing JavaScript objects and can be extended
to data of all types. This compact, easy-to-read format is ubiquitous
in Internet data APIs (in particular, RESTful services) and is the
standard format for many NoSQL solutions such as MongoDB and CouchDB.
As of version 9.3, the PostgreSQL database offers a JSON data type and
can query native JSON fields. The clear advantage is human
readability; the structure of the data is readily visible, and with
“pretty print,” even more so. In terms of Java, JSON is nothing more
than a collection of HashMaps
and
ArrayLists
, in any nested configuration imaginable. Each
line of the data from the prior examples can be formatted as a JSON
string by placing the values into key-value pairs; strings are in
double quotes (not single quotes), and
no trailing commas are allowed:
{
"id"
:
1
,
"year"
:
2015
,
"city"
:
"San Francisco"
}
{
"id"
:
2
,
"year"
:
2014
,
"city"
:
"New York"
}
{
"id"
:
3
,
"year"
:
2012
,
"city"
:
"Los Angeles"
}
Note that the entire file itself is not technically a JSON
object, and parsing the whole file as such will fail. To be valid JSON
format, each line would need to be separated by a comma and then the
entire group enclosed with square brackets. This would comprise a JSON
array. However, writing this kind of structure would be inefficient
and not useful. It is much more convenient and usable as is: a
line-by-line stack of JSON objects in string representation. Note that
the JSON parser does not know the type of the values in the key-value
pairs. So get the String
representation and then parse it
to its primitive type by using the boxed methods. It is
straightforward to build our dataset now, using org.simple.json
:
/* create JSON parser outside while loop */
JSONParser
parser
=
new
JSONParser
();
...
/* create an object by casting the parsed string */
JSONObject
obj
=
(
JSONObject
)
parser
.
parse
(
line
);
int
id
=
Integer
.
parseInt
(
j
.
get
(
"id"
).
toString
());
int
year
=
Integer
.
parseInt
(
j
.
get
(
"year"
).
toString
());
String
city
=
j
.
get
(
"city"
).
toString
();
Reading from a JSON File
This section covers files that are stringified JSON objects or arrays. You have to
know beforehand whether the file is a JSON object or an array. If you look at the file with, for
example, ls
on the command line, you can tell if it has
curly braces (object) or square braces (array):
{{
"id"
:
1
,
"year"
:
2015
,
"city"
:
"San Francisco"
},
{
"id"
:
2
,
"year"
:
2014
,
"city"
:
"New York"
},
{
"id"
:
3
,
"year"
:
2012
,
"city"
:
"Los Angeles"
}}
Then you use the Simple JSON library:
JSONParser
parser
=
new
JSONParser
();
try
{
JSONObject
jObj
=
(
JSONObject
)
parser
.
parse
(
new
FileReader
(
"data.json"
));
// TODO do something with jObj
}
catch
(
IOException
|
ParseException
e
)
{
System
.
err
.
println
(
e
.
getMessage
());
}
And if it’s an array,
[{
"id"
:
1
,
"year"
:
2015
,
"city"
:
"San Francisco"
},
{
"id"
:
2
,
"year"
:
2014
,
"city"
:
"New York"
},
{
"id"
:
3
,
"year"
:
2012
,
"city"
:
"Los Angeles"
}]
then you can parse the entire JSON array:
JSONParser
parser
=
new
JSONParser
();
try
{
JSONArray
jArr
=
(
JSONArray
)
parser
.
parse
(
new
FileReader
(
"data.json"
));
// TODO do something with jObj
}
catch
(
IOException
|
ParseException
e
)
{
System
.
err
.
println
(
e
.
getMessage
());
}
Warning
If you really have a file with one JSON object per line, the file is not technically a qualified JSON data structure. Refer back to “Reading from a Text File” where we read text files, parsing JSON objects one line at a time.
Reading from an Image File
When using images as input for learning, we need to convert from the image
format (e.g., PNG) to a data structure that is appropriate, such as a
matrix or vector. There are several points to consider
here. First, an image is a two-dimensional array with coordinates,
{x1, x2}, and a set of
associated color or intensity values, {y1…}, that
may be stored as a single, integer value. If all we want is the raw
value stored in a 2D integer array (labeled data
here), we
read in the buffered image with this:
BufferedImage
img
=
null
;
try
{
img
=
ImageIO
.
read
(
new
File
(
"Image.png"
));
int
height
=
img
.
getHeight
();
int
width
=
img
.
getWidth
();
int
[][]
data
=
new
int
[
height
][
width
];
for
(
int
i
=
0
;
i
<
height
;
i
++)
{
for
(
int
j
=
0
;
j
<
width
;
j
++)
{
int
rgb
=
img
.
getRGB
(
i
,
j
);
// negative integers
data
[
i
][
j
]
=
rgb
;
}
}
}
catch
(
IOException
e
)
{
// handle exception
}
We may want to convert the integer into its RGB (red, green, blue) components by bit shifting the integer:
int
blue
=
0x0000ff
&
rgb
;
int
green
=
0x0000ff
&
(
rgb
>>
8
);
int
red
=
0x0000ff
&
(
rgb
>>
16
);
int
alpha
=
0x0000ff
&
(
rgb
>>
24
);
However, we can get this information natively from the raster with this:
byte
[]
pixels
=
((
DataBufferByte
)
img
.
getRaster
().
getDataBuffer
()).
getData
();
for
(
int
i
=
0
;
i
<
pixels
.
length
/
3
;
i
++)
{
int
blue
=
Byte
.
toUnsignedInt
(
pixels
[
3
*
i
]);
int
green
=
Byte
.
toUnsignedInt
(
pixels
[
3
*
i
+
1
]);
int
red
=
Byte
.
toUnsignedInt
(
pixels
[
3
*
i
+
2
]);
}
Color may not be important. Perhaps grayscale is really all that’s needed:
//convert rgb to grayscale (0 to 1) where colors are on a scale of 0 to 255
double
gray
=
(
0.2126
*
red
+
0.7152
*
green
+
0.0722
*
blue
)
/
255.0
Also, in some cases the 2D representation is not necessary. We convert the matrix to a vector by concatenating each row of the matrix onto the new vector such that xn = x1, x2, ..., where the length n of the vector is m × p of the matrix, the number of rows times the number of columns. In the well-known MNIST dataset of handwritten images, the data has already been corrected (centered and cropped) and then converted into a binary format. So reading in that data requires a special format (see Appendix A), but it is already in vector (1D) as opposed to matrix (2D) format. Learning techniques on the MNIST dataset usually involve this vectorized format.
Writing to a Text File
Writing data to files has a general form of using the FileWriter
class, but once again
the recommended practice is to use the BufferedWriter
to avoid any I/O
errors. The general concept is to format all the data you want to write
to file as a single string. For the three variables in our example, we
can do this manually with a delimiter of choice (either a comma or
\t
):
/* for each instance Record record */
String
output
=
Integer
.
toString
(
record
.
id
)
+
","
+
Integer
.
toString
(
record
.
year
)
+
","
+
record
.
city
;
When using Java 8, the method String.join(delimiter, elements)
is
convenient!
/* in Java 8 */
String
newString
=
String
.
join
(
","
,
{
"a"
,
"b"
,
"c"
});
/* or feed in an Iterator */
String
newString
=
String
.
join
(
","
,
myList
);
Otherwise, you can instead use the Apache Commons Lang
StringUtils.join(elements, delimiter)
or the native StringBuilder
class in a loop:
/* in Java 7 */
String
[]
strings
=
{
"a"
,
"b"
,
"c"
};
/* create a StringBuilder and add the first member */
StringBuilder
sb
;
sb
.
append
(
strings
[
0
]);
/* skip the first string since we already have it */
for
(
int
i
=
1
;
i
<
strings
.
length
,
i
++){
/* choose a delimiter here ... could also be a \t for tabs */
sb
.
append
(
","
);
sb
.
append
(
strings
[
i
]);
}
String
newString
=
sb
.
toString
();
Note that successively using myString +=
myString_part
calls the StringBuilder
class, so you
might as well use StringBuilder
anyway (or not). In any
case, the strings are written line by line. Keep in mind that the
method BufferedWriter.write(String)
does not
write a new line! You will have to include a call to
BufferedWriter.newLine()
if you would like each data record to be on its own line:
try
(
BufferedWriter
bw
=
new
BufferedWriter
(
new
FileWriter
(
"somefile.txt"
))
)
{
for
(
String
s
:
myStringList
){
bw
.
write
(
s
);
/* don't forget to append a new line! */
bw
.
newLine
();
}
}
catch
(
Exception
e
)
{
System
.
out
.
println
(
e
.
getMessage
());
}
The preceding code overwrites all existing data in the file
designated by filename. In some situations, you will want to append data
to an existing file. The FileWriter
class takes an optional
Boolean field append
that defaults to false
if
it is excluded. To open a file for appending to the next available line,
use this:
/* setting FileWriter append bit keeps existing data and appends new data */
try
(
BufferedWriter
bw
=
new
BufferedWriter
(
new
FileWriter
(
"somefile.txt"
,
true
)
)
)
{
for
(
String
s
:
myStringList
)
{
bw
.
write
(
s
)
;
/* don't forget to append a new line! */
bw
.
newLine
(
)
;
}
}
catch
(
Exception
e
)
{
System
.
out
.
println
(
e
.
getMessage
(
)
)
;
}
Still another option is to use the PrintWriter
class, which wraps around the
BufferedWriter
. PrintWriter
and has a method
println()
that uses the native newline character of
whatever operating system you are on. So the \n
can be
excluded in the code. This has the advantage that you don’t have to
worry about adding those pesky newline characters. This could also be
useful if you are generating text files on your own computer (and
therefore OS) and will be consuming these files yourself. Here is an
example using PrintWriter
:
try
(
PrintWriter
pw
=
new
PrintWriter
(
new
BufferedWriter
(
new
FileWriter
(
"somefile.txt"
)))
)
{
for
(
String
s
:
myStringList
){
/* adds a new line for you! */
pw
.
println
(
s
);
}
}
catch
(
Exception
e
)
{
System
.
out
.
println
(
e
.
getMessage
());
}
Any of these methods work just fine with JSON data. Convert each
JSON object to a String
with the
JSONObject.toString()
method and write the String
. If you are writing one
JSON object, such as a configuration file, then it is as simple as
this:
JSONObject
obj
=
...
try
(
BufferedWriter
bw
=
new
BufferedWriter
(
new
FileWriter
(
"somefile.txt"
))
)
{
bw
.
write
(
obj
.
toString
());
}
}
catch
(
Exception
e
)
{
System
.
out
.
println
(
e
.
getMessage
());
}
When creating a JSON data file (a stack of JSON objects), loop
through your collection of JSONObjects
:
List
<
JSONObject
>
dataList
=
...
try
(
BufferedWriter
bw
=
new
BufferedWriter
(
new
FileWriter
(
"somefile.txt"
))
)
{
for
(
JSONObject
obj
:
dataList
){
bw
.
write
(
obj
.
toString
());
/* don't forget to append a new line! */
bw
.
newLine
();
}
}
catch
(
Exception
e
)
{
System
.
out
.
println
(
e
.
getMessage
());
}
Don’t forget to set the append-bit in FileWriter
if
this file is accumulative! You can add more JSON records to the end of
this file simply by setting the append-bit in the FileWriter
:
try
(
BufferedWriter
bw
=
new
BufferedWriter
(
new
FileWriter
(
"somefile.txt"
,
true
))
)
{
...
}
Mastering Database Operations
The robustness and flexibility of relational databases such as MySQL make them the ideal technology for a wide range of use cases. As a data scientist, you will most likely interact with relational databases in connection to a larger application, or perhaps you will generate tables of condensed and organized data specific to the tasks of the data science group. In either case, mastering the command line, Structured Query Language (SQL), and Java Database Connectivity (JDBC) are critical skills.
Command-Line Clients
The command line is a great environment for managing the database as well as performing queries. As an interactive shell, the client enables rapid iteration of commands useful for exploring the data. After you work out queries on the command line, you can later transfer the SQL to your Java program, where the query can be parameterized for more flexible use. All of the popular databases such as MySQL, PostgreSQL, and SQLite have command-line clients. On systems where MySQL has been installed for development purposes (e.g., your personal computer), you should be able to connect with an anonymous login with an optional database name:
bash$
mysql <database>
However, you might not be able to create a new database. You can log in as the database administrator:
bash$
mysql -u root <database>
Then you can have full access and privileges. In all other cases (e.g., you are connecting to a production machine, remote instance, or cloud-based instance), you will need the following:
bash$
mysql -h host -P port -u user -p password <database>
Upon connecting, you will be greeted with the MySQL shell, where you can make queries for showing all the databases you have access to, the name of the database you are connected to, and the username:
mysql> SHOW DATABASES;
To switch databases to a new database, the command is USE
dbname
:
mysql> USE myDB;
mysql> CREATE TABLE my_table(
id INT PRIMARY KEY, stuff VARCHAR(
256))
;
Even better, if you have those table creation scripts stored away as files, the following will read in and execute the file:
mysql> SOURCE <filename>;
Of course, you may want to know what tables are in your database:
mysql> SHOW TABLES;
You may also want to get a detailed description of a table, including column names, data types, and constraints:
mysql> DESCRIBE <tablename>;
Structured Query Language
Structured Query Language (SQL) is a powerful tool for exploring data. While object-relational mapping (ORM) frameworks have a place in enterprise software applications, you may find them too restrictive for the kinds of tasks you will face as a data scientist. It is a good idea to brush up on your SQL skills and be comfortable with the basics presented here.
Select
A generalized bare-bones SELECT
statement will have this
form:
SELECT
[
DISTINCT
]
col_name
,
col_name
,
...
col_name
FROM
table_name
[
WHERE
where_condition
]
[
GROUP
BY
col_name
[
ASC
|
DESC
]]
[
HAVING
where_condition
]
[
ORDER
BY
col_name
[
ASC
|
DESC
]]
[
LIMIT
row_count
OFFSET
offset
]
[
INTO
OUTFILE
'file_name'
]
A few tricks may come in handy. Suppose your dataset contains
millions of points, and you just want to get a general idea of the
shape. You can return a random sample by using ORDER BY
:
ORDER
BY
RAND
();
And you can set LIMIT
to the sample size you would
like back:
ORDER
BY
RAND
()
LIMIT
1000
;
Insert
Inserting data into a new row is implemented via the following:
INSERT
INTO
tablename
(
col1
,
col2
,
...)
VALUES
(
val1
,
val2
,
...);
Note that you can drop the column name entirely if the values account for all the columns and not just a subset:
INSERT
INTO
tablename
VALUES
(
val1
,
val2
,
...);
You can also insert multiple records at once:
INSERT
INTO
tablename
(
col1
,
col2
,
...)
VALUES
(
val1
,
val2
,
...),(
val1
,
val2
,
...),
(
val1
,
val2
,
...);
Update
On some occasions, you will need to alter an existing record. A lot of times this occurs quickly, on the command line, when you need to patch a mistake or correct a simple typo. Although you will undoubtedly access databases in production, analytics, and testing, you may also find yourself in an ad hoc DBA position. Updating records is common when dealing with real users and real data:
UPDATE
table_name
SET
col_name
=
'value'
WHERE
other_col_name
=
'other_val'
;
In the realm of data science, it is hard to envision a situation where you will be programmatically updating data. There will be exceptions, of course, such as the aforementioned typo corrections or when building a table piecemeal, but for the most part, updating important data sounds like a recipe for disaster. This is particularly true if multiple users are relying on the same data and have already written code, and subsequent analyses depend on a static dataset.
Delete
Deleting data is probably unnecessary in these days of cheap storage, but
just like UPDATE
, deleting will come in handy when you’ve
made an error and don’t want to rebuild your whole database.
Typically, you will be deleting records based on certain criteria,
such as a user_id
or record_id
, or before a
certain date:
DELETE
FROM
<
tablename
>
WHERE
<
col_name
>
=
'col_value'
;
Another useful command is TRUNCATE
, which deletes
all the data in a table but keeps the table
intact. Essentially, TRUNCATE
wipes a table clean:
TRUNCATE
<
tablename
>
;
Java Database Connectivity
The Java Database Connectivity (JDBC) is a protocol connecting Java applications with any SQL-compliant database. The JDBC drivers for each database vendor exist as a separate JAR that must be included in build and runtime. The JDBC technology strives for a uniform layer between applications and databases regardless of the vendor.
Connections
Connecting to a database with JDBC is extremely easy and convenient. All you need is a properly formed URI for the database that takes this general form:
String
uri
=
"jdbc:<dbtype>:[location]/<dbname>?<parameters>"
The DriverManager.getConnection()
method will throw an exception, and you have two choices for
dealing with this. The modern Java way is to put the connection inside
the try
statement, known as a
try with resource. In this way, the connection
will be automatically closed when the block is done executing, so you
do not have to explicitly put in a call to Connection.close()
.
Remember that if you decide to put the connection statement in the
actual try
block, you will need to explicitly close the
connection, probably in a finally
block:
String
uri
=
"jdbc:mysql://localhost:3306/myDB?user=root"
;
try
(
Connection
c
=
DriverManager
.
getConnection
(
uri
))
{
// TODO do something here
}
catch
(
SQLException
e
)
{
System
.
err
.
println
(
e
.
getMessage
());
}
Now that you have a connection, you need to ask yourself two questions:
Are there any variables in the SQL string (will the SQL string be altered in any way)?
Am I expecting any results to come back from the query other than an indicator that it was successful or not?
Start by assuming that you will create a Statement
. If the
Statement
will take a variable (e.g., if the SQL will be
appended to by an application variable), then use a PreparedStatement
instead. If you do
not expect any results back, you are OK. If you are expecting results
to come back, you need to use ResultSets
to contain and process the
results.
Statements
When executing an SQL statement, consider the following example:
DROP
TABLE
IF
EXISTS
data
;
CREATE
TABLE
IF
NOT
EXISTS
data
(
id
INTEGER
PRIMARY
KEY
,
yr
INTEGER
,
city
VARCHAR
(
80
));
INSERT
INTO
data
(
id
,
yr
,
city
)
VALUES
(
1
,
2015
,
"San Francisco"
),
(
2
,
2014
,
"New York"
),(
3
,
2012
,
"Los Angeles"
);
All of the SQL statements are hardcoded strings with
no varying parts. They return no values (other
than a Boolean return code) and can be executed, individually, inside
the above try-catch
block with this:
String
sql
=
"<sql string goes here>"
;
Statement
stmt
=
c
.
createStatement
();
stmt
.
execute
(
sql
);
stmt
.
close
();
Prepared statements
You will probably not be hardcoding all your data into an SQL statement.
Likewise, you may create a generic update statement for updating a
record’s city
column given an id
by using an
SQL WHERE
clause. Although you may be tempted to build
SQL strings by concatenating them, this is not a recommended practice.
Anytime external input is substituted into an SQL expression, there is
room for an SQL injection attack. The proper method is to use
placeholders (as question marks) in the SQL statement and then use the
class PreparedStatement
to properly quote the input
variables and execute the query. Prepared statements not only have a
security advantage but one of speed as well. The
PreparedStatement
is compiled one time, and for a large
number of inserts, this makes the process extremely efficient compared
to compiling a new SQL statement for each and every insertion. The
preceding INSERT
statement, with corresponding Java can
be written as follows:
String
insertSQL
=
"INSERT INTO data(id, yr, city) VALUES(?, ?, ?)"
;
PreparedStatement
ps
=
c
.
prepareStatement
(
insertSQL
);
/* set the value for each placeholder ? starting with index = 1 */
ps
.
setInt
(
1
,
1
);
ps
.
setInt
(
2
,
2015
);
ps
.
setString
(
3
,
"San Francisco"
);
ps
.
execute
();
ps
.
close
();
But what if you have a lot of data and need to loop through a
list? This is where you execute in batch mode.
For example, suppose you have a List
of
Record
objects obtained from an import of CSV:
String
insertSQL
=
"INSERT INTO data(id, yr, city) VALUES(?, ?, ?)"
;
PreparedStatement
ps
=
c
.
prepareStatement
(
insertSQL
);
List
<
Record
>
records
=
FileUtils
.
getRecordsFromCSV
();
for
(
Record
r:
records
)
{
ps
.
setInt
(
1
,
r
.
id
);
ps
.
setInt
(
2
,
r
.
year
);
ps
.
setString
(
3
,
r
.
city
);
ps
.
addBatch
();
}
ps
.
executeBatch
();
ps
.
close
();
Result sets
SELECT
statements return results! Anytime you find yourself writing
SELECT
you will need to properly call Statement.executeQuery()
instead of
execute()
and assign the return value to a
ResultSet
. In database-speak, the ResultSet
is a cursor that is an iterable data structure. As such, the Java
class ResultSet
implements the Java Iterator class and
the familiar while-next
loop can be used:
String
selectSQL
=
"SELECT id, yr, city FROM data"
;
Statement
st
=
c
.
createStatement
();
ResultSet
rs
=
st
.
executeQuery
(
selectSQL
);
while
(
rs
.
next
())
{
int
id
=
rs
.
getInt
(
"id"
);
int
year
=
rs
.
getInt
(
"yr"
);
String
city
=
rs
.
getString
(
"city"
));
// TODO do something with each row of values
}
rs
.
close
();
st
.
close
();
As in the case with reading files line by line, you must choose
what to do with the data. Perhaps you will store each value in an
array of that type, or perhaps you will store each row of data into a
class, and build a list with that class. Note that we are retrieving
the values from the ResultSet
instance by calling column
values by their column names according to the database schema. We can
instead increment through the column indices starting with 1.
Visualizing Data with Plots
Data visualization is an important and exciting component of data science. The combination of broadly available, interesting data and interactive graphical technologies has led to stunning visualizations, capable of telling complex stories. Many times, our visualizations are the eye candy that everyone has been anticipating. Of utmost importance is to realize that the same source of data can be used to tell completely different stories depending on not only the segment of the data you choose to show, but also the graphical styling utilized.
Keeping in mind that data visualization should always take into consideration the audience, there are roughly three kinds of consumers of a visualization. The first is yourself, the all-knowing expert who is most likely iterating quickly on an analysis or algorithm development. Your requirements are to see the data as plainly and quickly as possible. Things such as setting plot titles, axis labels, smoothing, legends, or date formatting might not be important, because you are intimately aware of what you are looking at. In essence, we often plot data to get a quick overview of the data landscape, without concerning ourselves with how others will view it.
The second consumer of data visualizations is the industry expert. After you have solved a data science problem and you think it’s ready to share, it’s essential to fully label the axis, put a meaningful, descriptive title on it, make sure any series of data are described by a legend, and ensure that the graphic you have created can mostly tell a story on its own. Even if it’s not visually stunning, your colleagues and peers will probably not be concerned with eye candy, but rather the message you are trying to convey. In fact, it will be much easier to make a scientific evaluation on the merits of the work if the visualization is clear of graphical widgets and effects. Of course, this format is also essential for archiving your data. One month later, you will not remember what those axes are if you don’t label them now!
The third category of visualization consumer is everybody else. This is the time to get creative and artistic, because a careful choice of colors and styles can make good data seem great. Be cautious, however, of the tremendous amount of time and effort you will spend preparing graphics at this level of consumer. An added advantage of using JavaFX is the interactivity allowed via mouse options. This enables you to build a graphical application similar to many of the web-based dashboards you are accustomed to.
Creating Simple Plots
Java contains native graphics capabilities in the JavaFX package. Since version 1.8, scientific plotting is
enabled with charts of many types such as scatter, line, bar, stacked
bar, pie, area, stacked area, or bubble via the
javafx.scene.chart
package. A Chart
object is
contained in a Scene
object, which is
contained in a Stage
object. The general form is to extend an executable
Java class with Application
and place all the plotting
directives in the overridden method Application.start()
. The
Application.launch()
method must be called in the main
method to create
and display the chart.
Scatter plots
An example of a simple plot is a scatter chart, which plots a set of x-y
pairs of numbers as points on a grid. These charts utilize the javafx.scene.chart.XYChart.Data
and
javafx.scene.chart.XYChart.Series
classes. The
Data
class is a container that holds any dimension of mixed types
of data, and the Series
class contains an
ObservableList
of Data
instances. There are
factory methods in the javafx.collections.FXCollections
class for creating instances of ObservableList
directly, should you prefer that route. However, for scatter, line,
area, bubble, and bar charts, this is unnecessary because they all
utilize the Series
class:
public
class
BasicScatterChart
extends
Application
{
public
static
void
main
(
String
[]
args
)
{
launch
(
args
);
}
@Override
public
void
start
(
Stage
stage
)
throws
Exception
{
int
[]
xData
=
{
1
,
2
,
3
,
4
,
5
};
double
[]
yData
=
{
1.3
,
2.1
,
3.3
,
4.0
,
4.8
};
/* add Data to a Series */
Series
series
=
new
Series
();
for
(
int
i
=
0
;
i
<
xData
.
length
;
i
++)
{
series
.
getData
().
add
(
new
Data
(
xData
[
i
],
yData
[
i
]));
}
/* define the axes */
NumberAxis
xAxis
=
new
NumberAxis
();
xAxis
.
setLabel
(
"x"
);
NumberAxis
yAxis
=
new
NumberAxis
();
yAxis
.
setLabel
(
"y"
);
/* create the scatter chart */
ScatterChart
<
Number
,
Number
>
scatterChart
=
new
ScatterChart
<>(
xAxis
,
yAxis
);
scatterChart
.
getData
().
add
(
series
);
/* create a scene using the chart */
Scene
scene
=
new
Scene
(
scatterChart
,
800
,
600
);
/* tell the stage what scene to use and render it! */
stage
.
setScene
(
scene
);
stage
.
show
();
}
}
Figure 1-1 depicts the default graphics window that is displayed when rendering a JavaFX chart for a simple set of data.

Figure 1-1. Scatter plot example
The ScatterChart
class can readily be replaced with LineChart
,
AreaChart
, or BubbleChart
in the
preceding example.
Bar charts
As an x-y chart, the bar chart utilizes the Data
and Series
classes.
In this case, however, the only difference is that the x-axis must be
a string type (as opposed to a numeric type) and utilizes the
CategoryAxis
class instead of the NumberAxis
class. The y-axis
remains as a NumberAxis
. Typically, the categories
in a bar chart are something like days of the week or market segments.
Note that the BarChart
class takes a
String
, Number
pair of types inside the
diamonds. These are useful for making histograms, and we show one in Chapter 3:
public
class
BasicBarChart
extends
Application
{
public
static
void
main
(
String
[]
args
)
{
launch
(
args
);
}
@Override
public
void
start
(
Stage
stage
)
throws
Exception
{
String
[]
xData
=
{
"Mon"
,
"Tues"
,
"Wed"
,
"Thurs"
,
"Fri"
};
double
[]
yData
=
{
1.3
,
2.1
,
3.3
,
4.0
,
4.8
};
/* add Data to a Series */
Series
series
=
new
Series
();
for
(
int
i
=
0
;
i
<
xData
.
length
;
i
++)
{
series
.
getData
().
add
(
new
Data
(
xData
[
i
],
yData
[
i
]));
}
/* define the axes */
CategoryAxis
xAxis
=
new
CategoryAxis
();
xAxis
.
setLabel
(
"x"
);
NumberAxis
yAxis
=
new
NumberAxis
();
yAxis
.
setLabel
(
"y"
);
/* create the bar chart */
BarChart
<
String
,
Number
>
barChart
=
new
barChart
<>(
xAxis
,
yAxis
);
barChart
.
getData
().
add
(
series
);
/* create a scene using the chart */
Scene
scene
=
new
Scene
(
barChart
,
800
,
600
);
/* tell the stage what scene to use and render it! */
stage
.
setScene
(
scene
);
stage
.
show
();
}
}
Plotting multiple series
Multiple series of any type of plot are easily implemented. In the case
of the scatter plot example, you need only to create multiple Series
instances:
Series
series1
=
new
Series
();
Series
series2
=
new
Series
();
Series
series3
=
new
Series
();
The series are then added in all at once using the
addAll()
method instead of the add()
method:
scatterChart
.
getData
().
addAll
(
series1
,
series2
,
series3
);
The resultant plot will show the points superimposed in various
colors with a legend denoting their label name. The same holds true
for line, area, bar, and bubble charts. An interesting feature here is
the StackedAreaChart
and StackedBarChart
classes, which operate the same way as their respective AreaChart
and
BarChart
superclasses, except that the data are stacked
one above the other so they do not overlap visually.
Of course, sometimes a visualization would benefit from mixing
data from multiple plot types, such as a scatter plot of data with a
line plot running through the data. Currently, the Scene
class accepts only charts of one type. However, we will
demonstrate some workarounds later in this chapter.
Basic formatting
There are useful options for making your plot look really professional. The first place to cleanup might be the axes. Often the minor ticks are overkill. We can also set the plot range with minimum and maximum values:
scatterChart
.
setBackground
(
null
);
scatterChart
.
setLegendVisible
(
false
);
scatterChart
.
setHorizontalGridLinesVisible
(
false
);
scatterChart
.
setVerticalGridLinesVisible
(
false
);
scatterChart
.
setVerticalZeroLineVisible
(
false
);
At some point, it might be easier to keep the plotting mechanics simple and include all the style directives in a CSS file. The default CSS for JavaFX8 is called Modena and will be implemented if you don’t change the style options. You can create your own CSS and include it in the scene with this:
scene
.
getStylesheets
().
add
(
"chart.css"
);
The default path is in the src/main/resources directory of your Java package.
Plotting Mixed Chart Types
Often we want to display multiple plot types in one graphic—for example,
when you want to display the data points as an x-y scatter plot and then
overlay a line plot of the best fitted model. Perhaps you will also want
to include two more lines to represent the boundary of the model,
probably one, two, or three multiples of the standard deviation σ, or
the confidence interval 1.96 × σ. Currently, JavaFX does not allow
multiple plots of the different types to be displayed simultaneously on
the same scene. There is a workaround, however! We can use a
LineChart
class to plot multiple series of LineChart
instances
and then use CSS to style one of the lines to show only points, one to
only show a solid line, and two to show only a dashed line. Here is the
CSS:
.default-color0.chart-series-line
{
-
fx
-
stroke
:
transparent
;
}
.default-color1.chart-series-line
{
-
fx
-
stroke
:
blue
;
-
fx
-
stroke-width
:
1
;
}
.default-color2.chart-series-line
{
-
fx
-
stroke
:
blue
;
-
fx
-
stroke-width
:
1
;
-
fx
-
stroke
-
dash
-
array
:
1
4
1
4
;
}
.default-color3.chart-series-line
{
-
fx
-
stroke
:
blue
;
-
fx
-
stroke-width
:
1
;
-
fx
-
stroke
-
dash
-
array
:
1
4
1
4
;
}
/*.default-color0.chart-line-symbol {
-fx-background-color: white, green;
}*/
.default-color1.chart-line-symbol
{
-
fx
-
background-color
:
transparent
,
transparent
;
}
.default-color2.chart-line-symbol
{
-
fx
-
background-color
:
transparent
,
transparent
;
}
.default-color3.chart-line-symbol
{
-
fx
-
background-color
:
transparent
,
transparent
;
}
The plot looks like Figure 1-2.
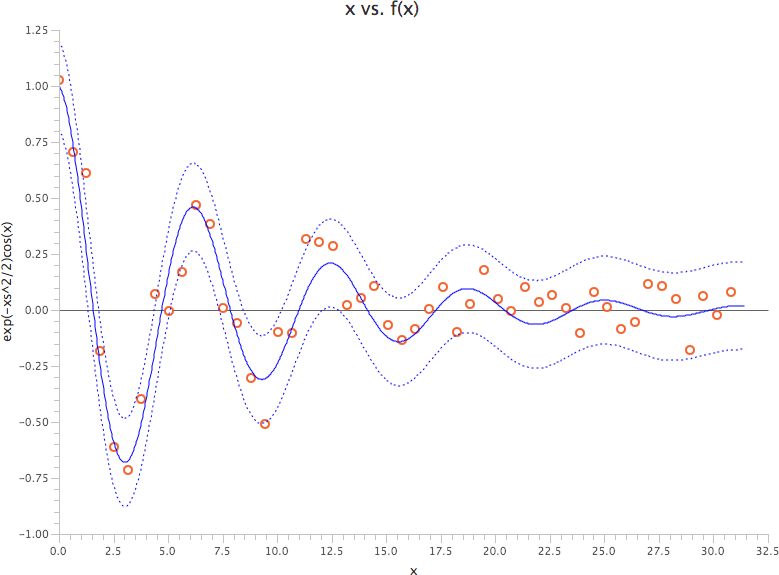
Figure 1-2. Plot of mixed line types with CSS
Saving a Plot to a File
You will undoubtedly have an occasion to save a plot to a file. Perhaps you will be sending the plot off in an email or including it in a presentation. With a mixture of standard Java classes and JavaFX classes, you can easily save plots to any number of formats. With CSS, you can even style your plots to have publication-quality graphics. Indeed, the figures in this chapter (and the rest of the book) were prepared this way.
Each chart type subclasses the abstract class Chart
, which inherits the method
snapshot()
from the Node
class. Chart.snapshot()
returns a WritableImage
. There is one catch that must be addressed:
in the time it takes the scene to render the data on the chart, the
image will be saved to a file without the actual data on the plot. It is
critical to turn off animation via Chart.setAnimated(false)
someplace after
the chart is instantiated and before data is added to the chart with
Chart.getData.add()
or its equivalent:
/* do this right after the chart is instantiated */
scatterChart
.
setAnimated
(
false
);
...
/* render the image */
stage
.
show
();
...
/* save the chart to a file AFTER the stage is rendered */
WritableImage
image
=
scatterChart
.
snapshot
(
new
SnapshotParameters
(),
null
);
File
file
=
new
File
(
"chart.png"
);
ImageIO
.
write
(
SwingFXUtils
.
fromFXImage
(
image
,
null
),
"png"
,
file
);
Note
All the data plots in this book were created with JavaFX 8.
Get Data Science with Java now with the O’Reilly learning platform.
O’Reilly members experience books, live events, courses curated by job role, and more from O’Reilly and nearly 200 top publishers.