Chapter 7. Machine Learning for Microscopy
In this chapter, we introduce you to deep learning techniques for microscopy. In such applications, we seek to understand the biological structure of a microscopic image. For example, we might be interested in counting the number of cells of a particular type in a given image, or we might seek to identify particular organelles. Microscopy is one of the most fundamental tools for the life sciences, and advances in microscopy have greatly advanced human science. Seeing is believing even for skeptical scientists, and being able to visually inspect biological entities such as cells builds an intuitive understanding of the underlying mechanisms of life. A vibrant visualization of cell nuclei and cytoskeletons (as in Figure 7-1) builds a much deeper understanding than a dry discussion in a textbook.
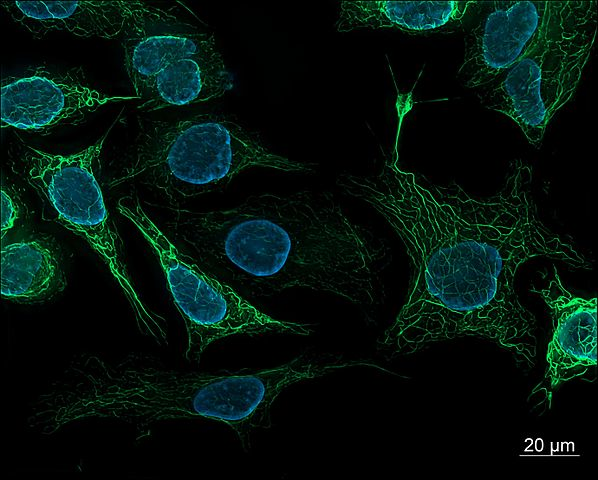
Figure 7-1. Human-derived SK8/18-2 cells. These cells are stained to highlight their nuclei and cytoskeletons and imaged using fluorescence microscopy. (Source: Wikimedia.)
The question remains how deep learning can make a difference in microscopy. Until recently, the only way to analyze microscopy images was to have humans (often graduate students or research associates) manually inspect these images for useful patterns. More recently, tools such as CellProfiler have made it possible for biologists to automatically assemble pipelines for handling ...
Get Deep Learning for the Life Sciences now with the O’Reilly learning platform.
O’Reilly members experience books, live events, courses curated by job role, and more from O’Reilly and nearly 200 top publishers.