Chapter 5. Machine Learning (ML)
AI relies on large volumes of historical data and advanced ML methods to generate insights. In the last chapter, we focused on data’s importance to your organization’s success when implementing AI. If data is the fuel that powers your AI applications, then ML is its engine.
The previous two chapters discuss how to coordinate AI analytic development with DataOps and key stakeholders, such as data owners and risk managers. This section will discuss AI development itself and how its tooling and process can evolve toward a more sustainable, scalable, and coordinated capability.
When considering how ML fits within the wider AI domain, it’s important to first understand the data analytics spectrum. Figure 5-1 illustrates the differentiation between conventional statistical analysis (i.e., forensic analytics, which examines past and present phenomena) and anticipatory analytics (i.e., forward-looking analysis).
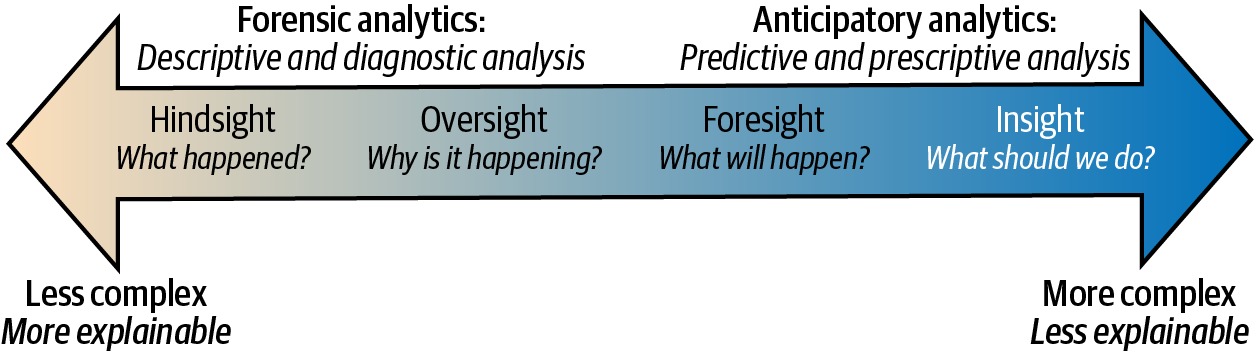
Figure 5-1. Complexity versus explainability for analyses
Each analytic approach is associated with specific challenges, with more advanced approaches providing the decision support and cognitive analysis typically associated with robust AI capabilities. A key distinction is the interplay between complexity and explainability. In most cases, forensic analysis is far less complex and more explainable than anticipatory analytics, which is rapidly ...
Get Enterprise AIOps now with the O’Reilly learning platform.
O’Reilly members experience books, live events, courses curated by job role, and more from O’Reilly and nearly 200 top publishers.