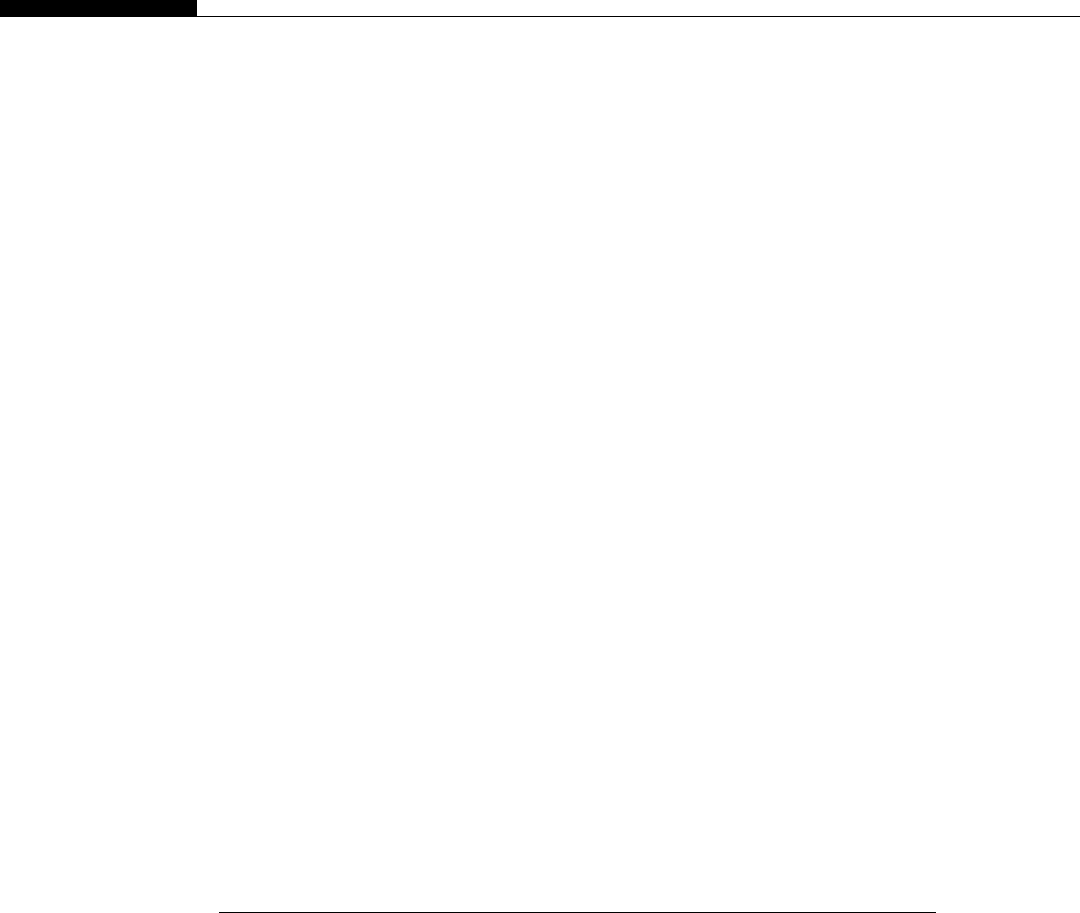
in the form of either perceptual representations or control schemas [2, 4, 5, 46, 48].
Here is the critical point. If we can make a language out of the sensorimotor represen-
tations that arise from our actions (in general, interactions with our environment),
then we can obtain abstract descriptions of human activity from non text (language)
data (sensory and motor). These representations are immediately useful since they
can ground basic verbs (e.g., walk, turn, sit, kick). It is intuitively clear that we
humans understand a sentence like “Joe ran to the store” not because we check
“ran” in the dictionary but because we have a sensorimotor experience of running.
We know what it means to “run,” we can “run” if we wish, we can think of “run ning.”
We have functional representations of running that our language of action provides.
While such physical descriptions are useful for some classes of words (e.g., col-
ors, shapes, physical movements), they may not be sufficient for more abstract lan-
guage, such as that for intentional action. This insufficiency stems from the fact that
intentional actions (i.e., actions performed with the purpose of achieving a goal) are
highly ambiguous when described only in terms of their physically observable char-
acteristics. Imagine a situation in which one person moves a cup toward another
person and says the unknown word “trackot.” Based only on the physical description
of this action, one might come to think of “trackot” as meaning anything from “give
cup” to “offer drink” to “ask for change.” This ambiguity stems from the lack of con-
textual information that strictly perceptual descriptions of action provide.
A language of action provides a methodology for grounding the meaning of actions,
ranging from simple movement to intentional acts (e.g., “walk to the store” versus “go
to the store,” “slide the cup to him” versus “give him the cup”), by combining the
grammatical structure of action (motoric and visual) with the well-known grammatical
structure of planning or intent. Specifically, one can combine the bottom-up structure
discovered from movement data with the top-down structure of annotated intentions.
The bottom-up process can give us the actual hierarchical composition of behavior;
the top-down process gives us intentionally laden interpretations of those structures.
It is likely that top-down annotations will not reach down to visual-motor phonology,
but they will perhaps be aligned at the level of visuo-motor morphology or even visuo-
motor clauses.
5.8 CONCLUSIONS
Human-centric interfaces not only promise to dominate our futu re in many applica-
tions, but might also begin a new phase in artificial intelligence by studying meaning
through the utilization of both sensorimotor and symbolic representations, using
machine learning techniques on the gargantuan amounts of data collected. This will
lead eventually to the creation of the praxicon, an extension of the lexicon that con-
tains sensorimotor abstractions of the items in the lexicon [1]. The entire enterprise
may be seen in light of the emerging network science, the study of human behavior
not in isolation but in rel ation to other humans and the environment. In this
endeavor, languages of human action will play a very important role.
128 CHAPTER 5 The Language of Action
Get Human-Centric Interfaces for Ambient Intelligence now with the O’Reilly learning platform.
O’Reilly members experience books, live events, courses curated by job role, and more from O’Reilly and nearly 200 top publishers.