7Identification of State Space Models
7.1 Introduction
In this chapter, we address the problem o black box identification of state space models, namely the problem of estimating the system matrices directly from input–output observations. To face it, we introduce a new estimation paradigm, different from the ideas around which the previous approach of parametric identification via the prediction error rationale is hinged. The new estimation paradigm is rooted in system notions such as reachability, observability, and system realization. The readers who are not familiar with such topics are referred to Appendix A.
Actually, many methods have been studied in this field, giving rise to a branch of system identification known in our days as subspace identification methods. Herein we present a basic technique, the roots of which go back to the celebrated paper by Ho and Kalman (1965). We focus on SISO deterministic models:

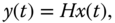
where
- input
and output
are scalar,
- state
is a vector ...
Get Model Identification and Data Analysis now with the O’Reilly learning platform.
O’Reilly members experience books, live events, courses curated by job role, and more from O’Reilly and nearly 200 top publishers.