For a deeper understanding of how GANs work, we'll use a GAN to solve a simple problem in TensorFlow, namely, learning to approximate a one-dimensional Gaussian distribution.
First, we will create the actual data distribution, a simple Gaussian with a mean of four and standard deviation of 0.5. It has a sample function that returns a given number of samples (sorted by value) from the distribution. The data distribution that we learn will look like the following diagram:
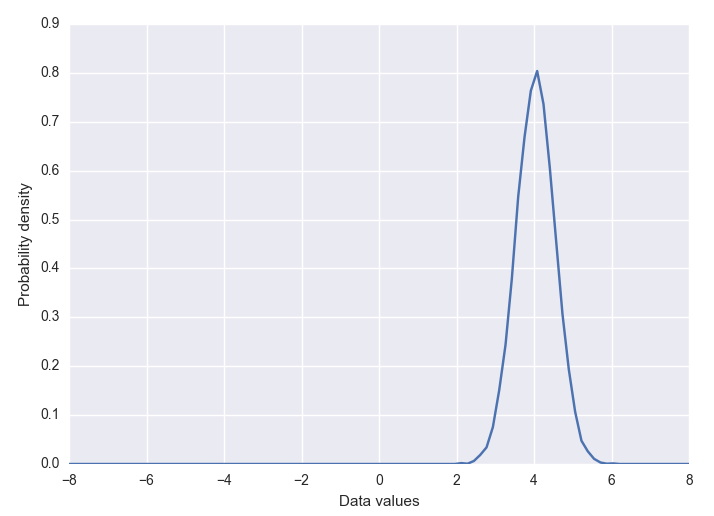
Generator input noise distribution is also defined with a similar sample function used for actual data.
Both generator and discriminator networks are very ...