Chapter 2. Data and Sampling Distributions
A popular misconception holds that the era of big data means the end of a need for sampling. In fact, the proliferation of data of varying quality and relevance reinforces the need for sampling as a tool to work efficiently with a variety of data and to minimize bias. Even in a big data project, predictive models are typically developed and piloted with samples. Samples are also used in tests of various sorts (e.g., pricing, web treatments).
Figure 2-1 shows a schematic that underpins the concepts in this chapter. The lefthand side represents a population that, in statistics, is assumed to follow an underlying but unknown distribution. The only thing available is the sample data and its empirical distribution, shown on the righthand side. To get from the lefthand side to the righthand side, a sampling procedure is used (represented by an arrow). Traditional statistics focused very much on the lefthand side, using theory based on strong assumptions about the population. Modern statistics has moved to the righthand side, where such assumptions are not needed.
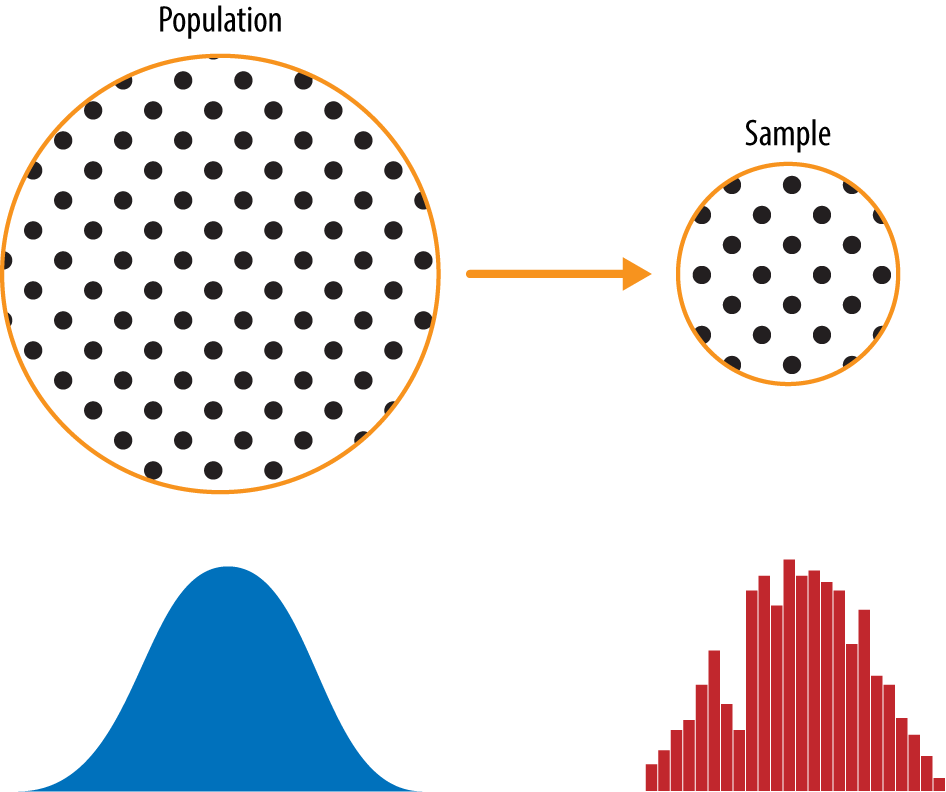
Figure 2-1. Population versus sample
In general, data scientists need not worry about the theoretical nature of the lefthand side, and instead should focus on the sampling procedures and the data at hand. There are some notable exceptions. Sometimes data is generated from a physical ...
Get Practical Statistics for Data Scientists now with the O’Reilly learning platform.
O’Reilly members experience books, live events, courses curated by job role, and more from O’Reilly and nearly 200 top publishers.