Predictive Analytics for Healthcare
Healthcare has always relied on the use of data to describe what is wrong with a patient (called descriptive analytics), what has caused the problem (diagnostic analytics), what the likely future potential outcomes and complications are (predictive analytics), and what is most likely to be a suitable course of treatment (prescriptive analytics). Each of these steps gives increasingly valuable clinical insight, but is also correspondingly difficult to achieve accurately and reliably. Figure 1 illustrates this idea.
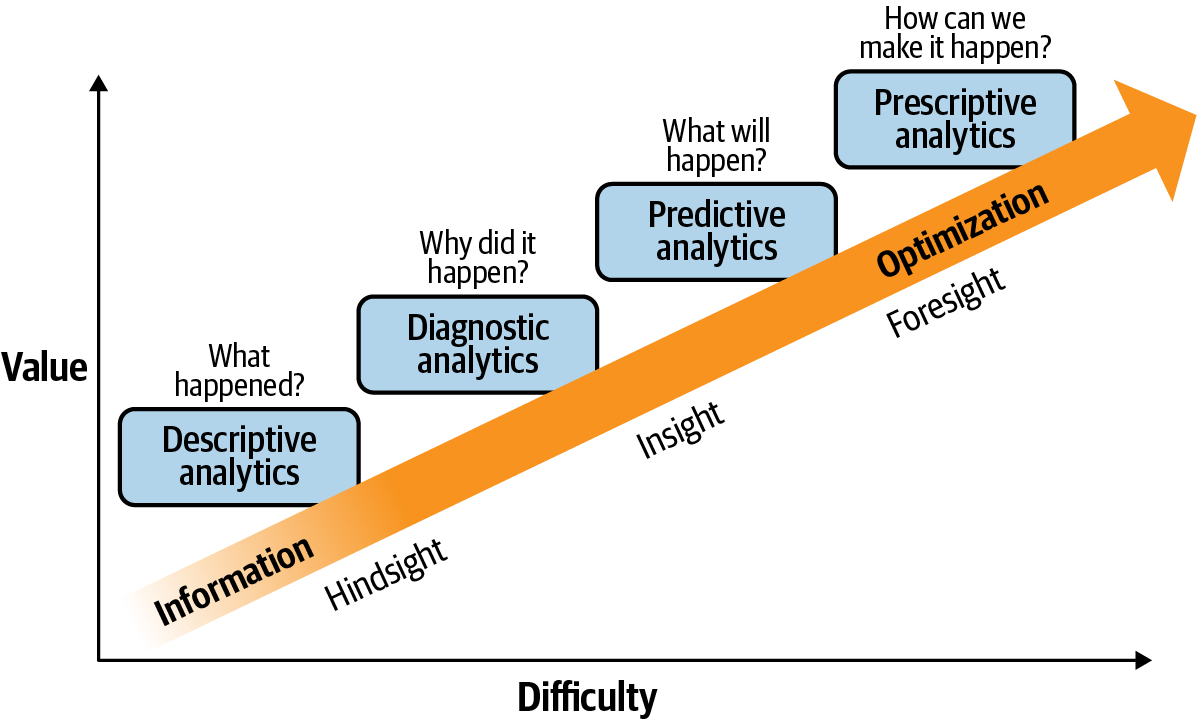
Figure 1. The Gartner analytic ascendancy model
Introducing Predictive Analytics
A key part of predictive analytics (PA) is the creation of a model that allows historical and current data to be extrapolated into the future to aid decision-making. Historically, this model has sat in the head of the physicianâbuilt on their personal range of historical data, from medical school training and experience, to keeping up with the results of the latest randomized control trials.
Unprecedented amounts of clinical data are being collected in electronic format on the characteristics of the individual patient, the treatments applied, and the resulting outcomes. At the same time, we are seeing progress in the techniques and hardware to implement neural networkâbased artificial intelligence (AI) approaches. Together these have the potential ...
Get Predictive Analytics for Healthcare now with the O’Reilly learning platform.
O’Reilly members experience books, live events, courses curated by job role, and more from O’Reilly and nearly 200 top publishers.