Chapter 1
Introduction 1
Mathematical techniques for modeling random phenomena have found a natural application in the field of image analysis. An image is itself a noisy signal, due to the characteristics of the imaging sensor and the conditions of image acquisition or transmission. Noise reduction techniques, low-pass filtering, and Kalman filters, all of which were initially developed in signal theory, have been easily generalized to apply to the case of two-dimensional (2D) images, and thereafter to higher dimensions. Nevertheless, the random phenomena within an image are not solely the result of noise. The content of the image itself can be regarded as the product of a random process. The texture present in an image cannot be directly interpreted from the set of pixel values, but is better understood through a set of stationary statistics that characterize the texture itself, or by a probabilistic model and the values of its parameters. Thus, it is in fact the statistics of the pixels forming Lena’s famous hat (see Figure 1.1) which enable it to be characterized. In the same way, a segmented image, representing, for example, some plots of agricultural land, contains a randomness, in the sense that different parts of the same area in the image have common properties but also show certain variations (see Figure 1.2).
Figure 1.1. Lena and her feathered hat
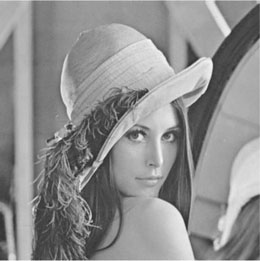
Figure 1.2. Two examples ...
Get Stochastic Geometry for Image Analysis now with the O’Reilly learning platform.
O’Reilly members experience books, live events, courses curated by job role, and more from O’Reilly and nearly 200 top publishers.