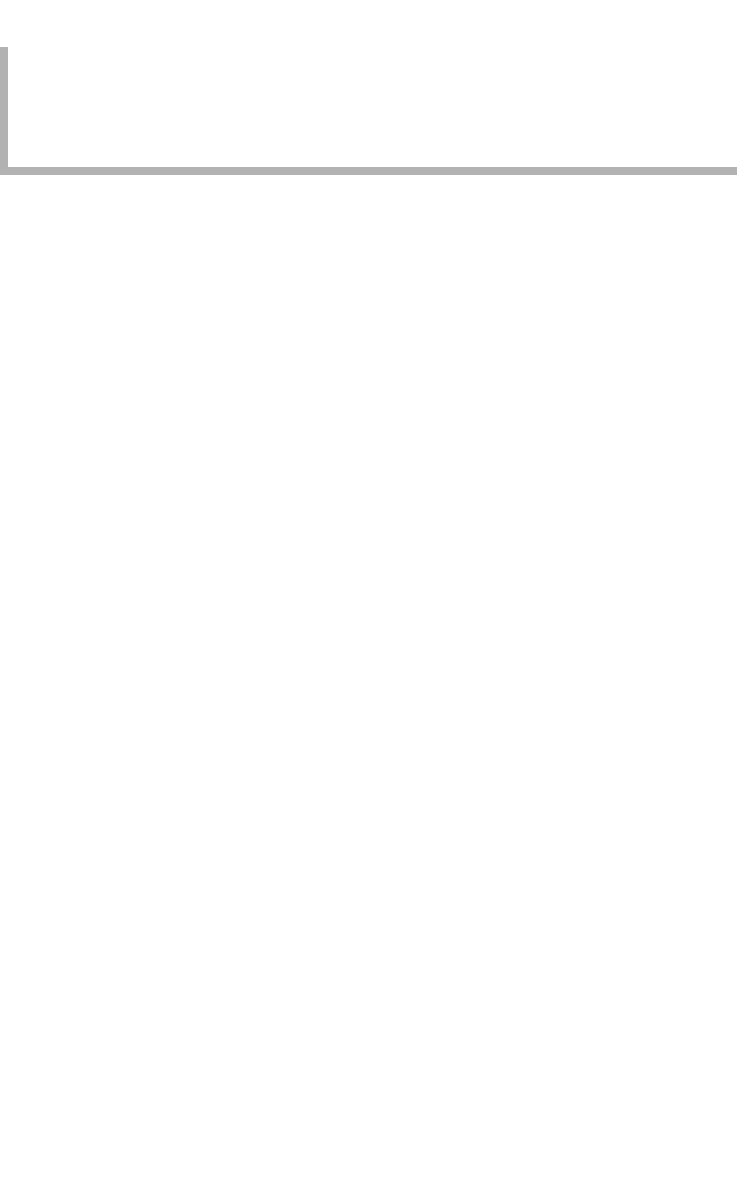
Data analysis 163
7. The way you should report this finding when you are writing about it
in your report is as follows. The results reveal a statistically significant
correlation between age and attitude towards mobile phone use in
public places (r 0.15, p 0.01).
One thing that you should be aware of when you are trying to interpret
your correlation results is that correlation does not imply causation.
What does this mean? I hear you ask. It means that you can’t assume
that one variable has a causal effect on the other one. For example,
although you might find there is a relationship between age and attitude
towards mobile phone use in public places, this could be related to the
fact that older people may not have thought they need to use the types
of services that people normally use mobile phones in public for.
ANALYSING CATEGORICAL DATA
This type of analysis requires you to allocate your participants to specific
category scales (i.e. nominal data scales) rather than acquiring data from
them in ways similar to what we’ve already discussed in this chapter (e.g.
attitude scores or performance measures such as time taken to complete
a task). For example, this type of data can be obtained from information
recorded by the researcher in an observational coding form (observa-
tional coding forms were one of the research approaches discussed in
Chapter 3). A test that can be used to assess for statistical significance for
data collected in this way is called the CHI-square Test (also written as
2
). This will be the first test discussed in this section of the chapter.
Alternatively, you can collect qualitative information from partici-
pants in the form of open-ended questions (e.g. what did you like about
the mobile tourist information guide?), and attempt to put the qualita-
tive information obtained from these questions into categories in order
to quantify the data. This approach is known as content analysis and it
the second technique to be discussed in this section of the chapter.
CHI-square test
The CHI-square test is used when you have collected data in the form
of frequencies and it is traditionally used in an unrelated design. As
ever, the best way to explain this test is to work through an example
together and this is shown in Technical Tip 7.
H6352-Ch08.qxd 7/18/05 3:40 PM Page 163
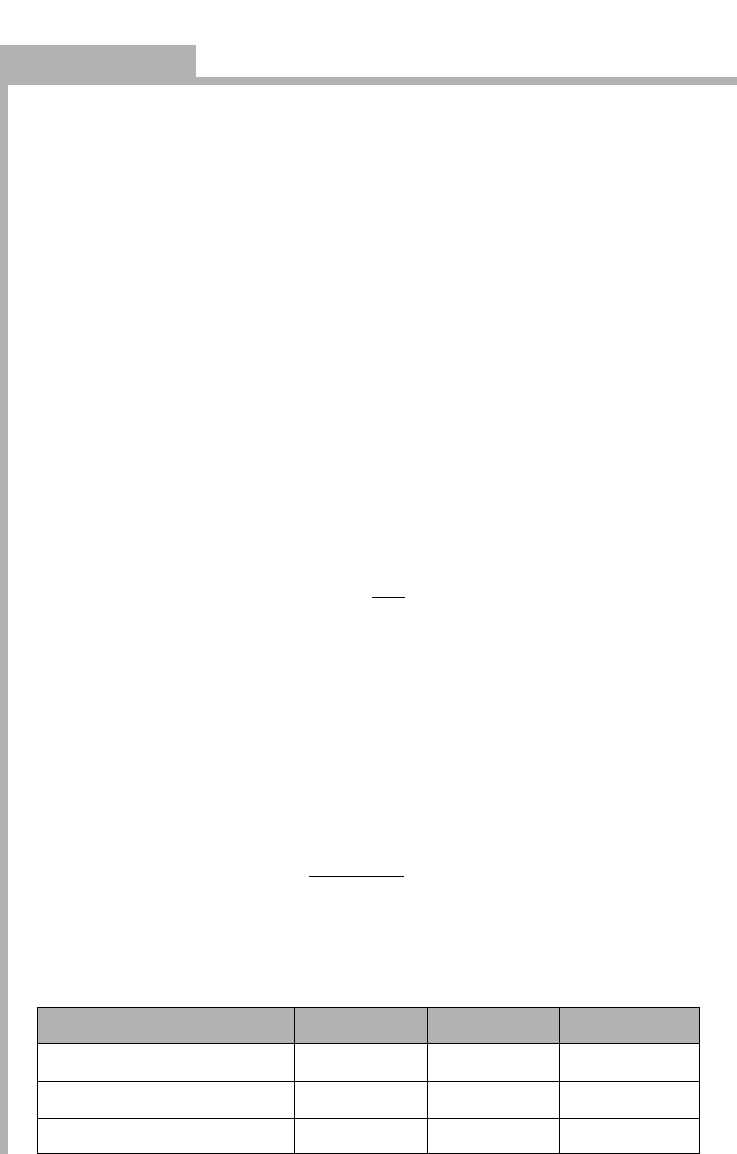
Technical Tip 7
Calculating a CHI-square test
The data you have collected for this study comes from the observations
made by a researcher over a period of two days in a busy café. The
researcher was interested in assessing if there were any gender differences
in relation to whether or not an individual would answer their mobile
phone if they were in the company of other people. In order to carry out
this study, the researcher followed the instructions given in Chapter 3
to create an observational coding form. At the end of the observation
period, the researcher had collected the following data displayed here in
Table 8.15.
1. The first thing that you have to do is to give the observed frequencies
a letter for each cell (this should explain any confusion you may have
had about the letters a-d appearing in Table 8.15).
2. The next step is to calculate the expected frequencies for each cell
using this formula:
E expected frequency for a particular cell (a,b,c or d)
R total of row cells (a b) or (c d)
C total of column cells (a c) or (b d)
T total of all cells (a b c d)
So, the Expected frequency for the four cells in this example should be
as follows:
E
a
129 131
257
65 8.
E
RC
T
164 Understanding Mobile Human–Computer Interaction
Female Male Total
Had conversation 40 (a) 89 (b) 129
Did not have conversation 91 (c) 37 (d) 128
Total 131 126 257
Table 8.15 Frequency Data Obtained from Observational Coding Form
H6352-Ch08.qxd 7/18/05 3:40 PM Page 164
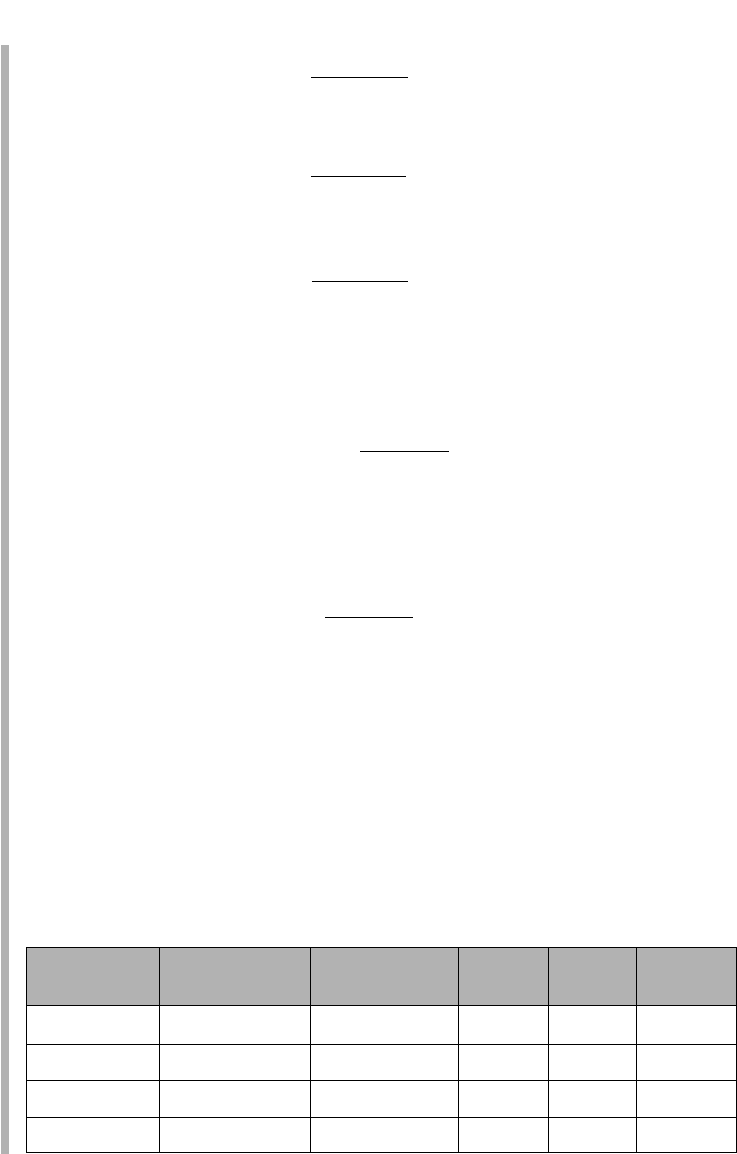
Data analysis 165
The next step is to calculate the value of the
2
by using the following
formula:
3. Remember that you have to calculate the following part of the formula
for each of the four cells first:
To make this step easier to calculate, arrange your data similar to
Table 8.16.
4. According to my calculations the final calculation should look some-
thing like:
2
10.1 10.5 10.2 10.5 43.3
()OE
E
2
2
2
()OE
E
E
d
128 126
257
62.8
E
c
128 131
257
65.2
E
b
129 126
257
63.2
Cell Number Observed Expected (O E)(O E)
2
(O E)
2
/E
Frequency (O) Frequency (E)
A 40 65.8 25.8 665.64 10.1
B 89 63.2 25.8 665.64 10.5
C 91 65.2 25.8 665.64 10.2
D 37 62.8 25.8 665.64 10.5
Table 8.16 Data Table for Calculating a CHI-Square Test
H6352-Ch08.qxd 7/18/05 3:40 PM Page 165
Get Understanding Mobile Human-Computer Interaction now with the O’Reilly learning platform.
O’Reilly members experience books, live events, courses curated by job role, and more from O’Reilly and nearly 200 top publishers.