Artificial intelligence and machine learning adoption in European enterprise
How companies in Europe are preparing for and adopting AI and ML technologies.
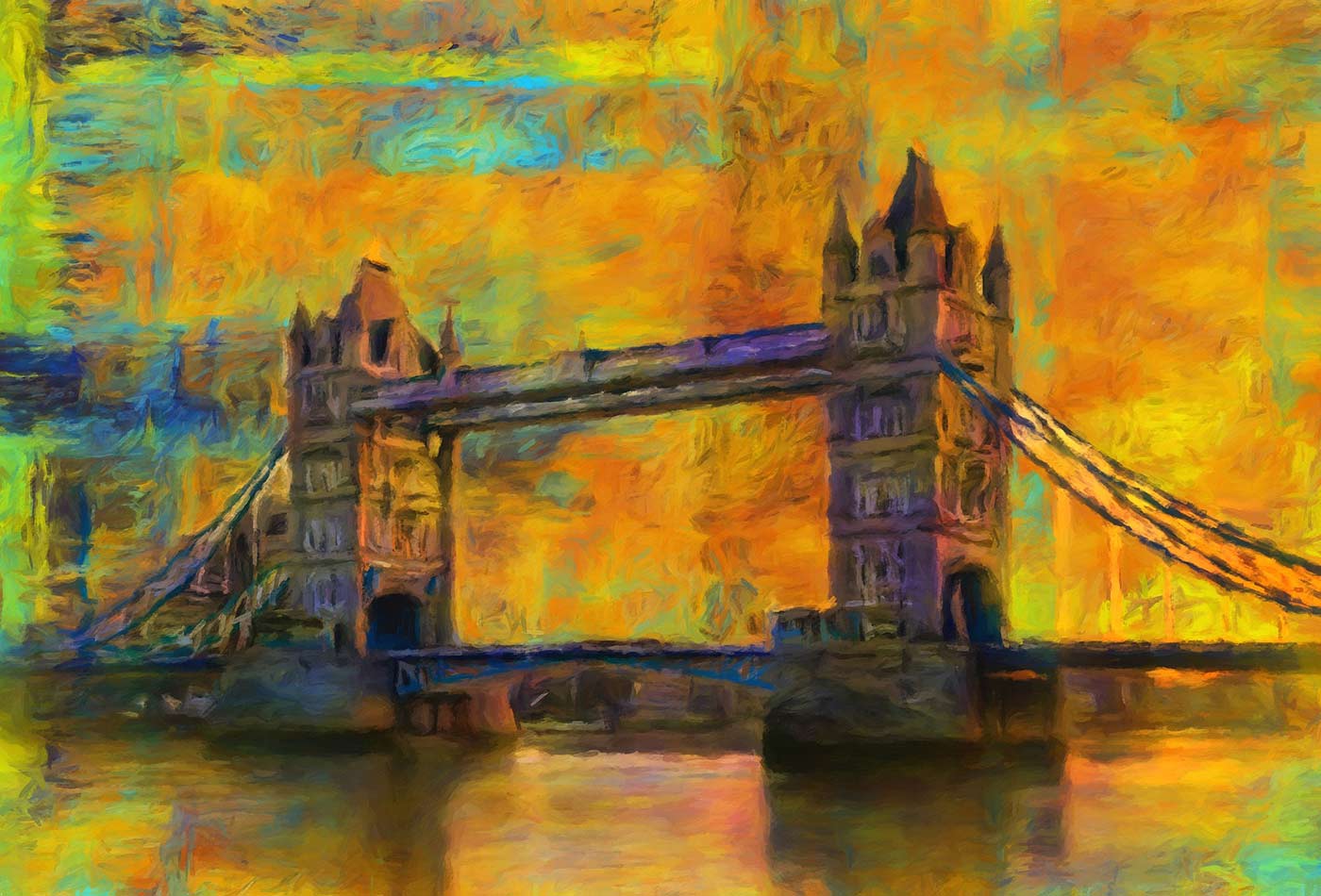
In a recent survey, we explored how companies were adjusting to the growing importance of machine learning and analytics, while also preparing for the explosion in the number of data sources. In practice this means developing a coherent strategy for integrating artificial intelligence (AI), big data, and cloud components, and specifically investing in foundational technologies needed to sustain the sensible use of data, analytics, and machine learning. (You can find full results from the survey in the free report “Evolving Data Infrastructure”.)
This survey drew from more than 3,200 respondents, including more than 1,000 respondents from Western and Eastern Europe. In this post, I’ll describe some of the key areas of interest and concern highlighted by respondents from Europe, while describing how some of these topics will be covered at the upcoming Strata Data conference in London (April 29 – May 2, 2019).
As interest in machine learning (ML) and AI grow, organizations are realizing that model building is but one aspect they need to plan for. Given the end-to-end nature of many data products and applications, sustaining ML and AI requires a host of tools and processes, ranging from collecting, cleaning, and harmonizing data, understanding what data is available and who has access to it, being able to trace changes made to data as it travels across a pipeline, and many other components. Our survey showed that companies are beginning to build some of the foundational pieces needed to sustain ML and AI within their organizations:
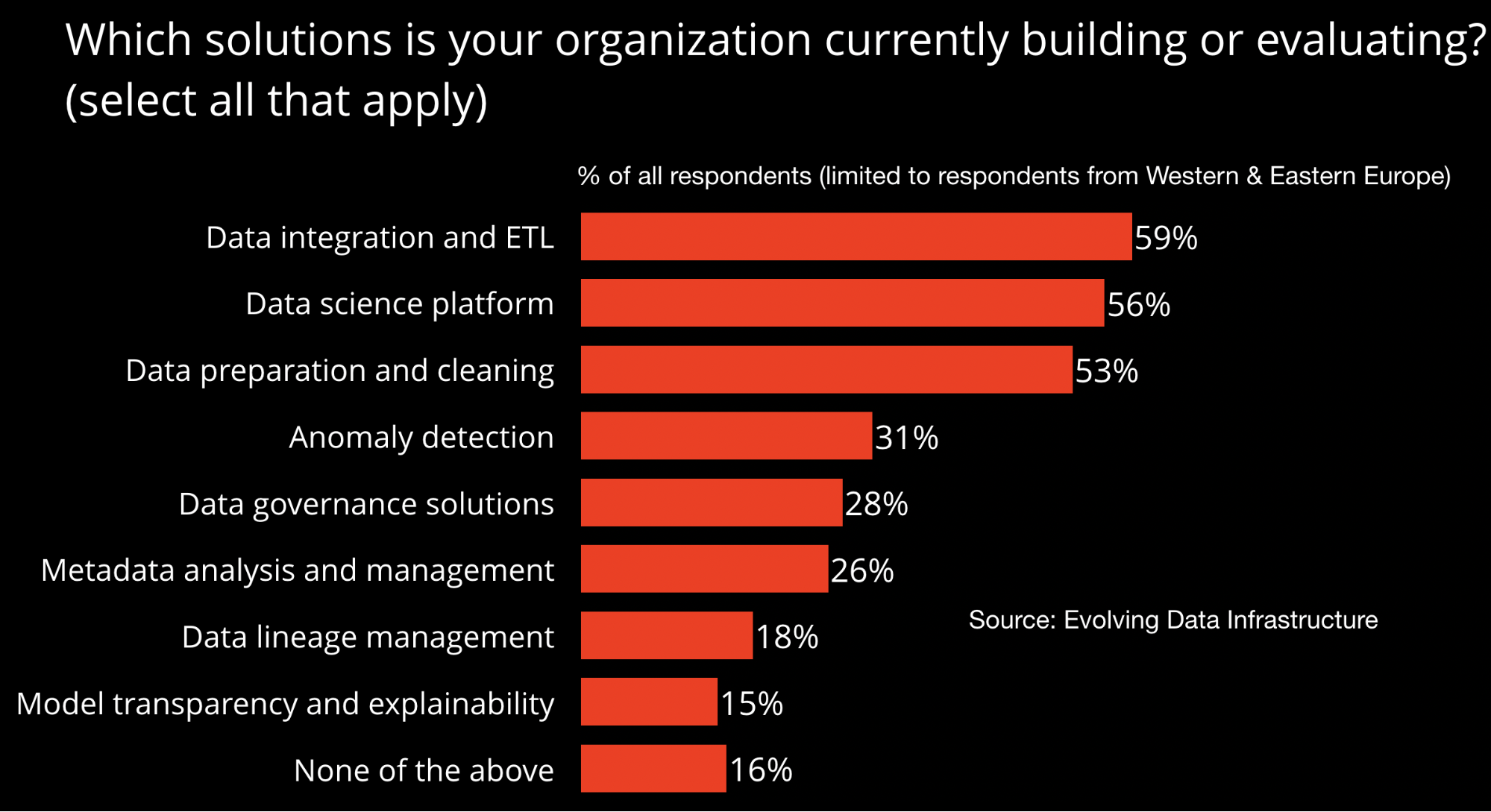
Solutions, including those for data governance, data lineage management, data integration and ETL, need to integrate with existing big data technologies used within companies. To that end, we also asked respondents what technologies (open source, managed services) they use for things like data storage, data management, and data processing. For example, the chart below lists popular (batch and streaming) data processing tools used by respondents based in Europe:
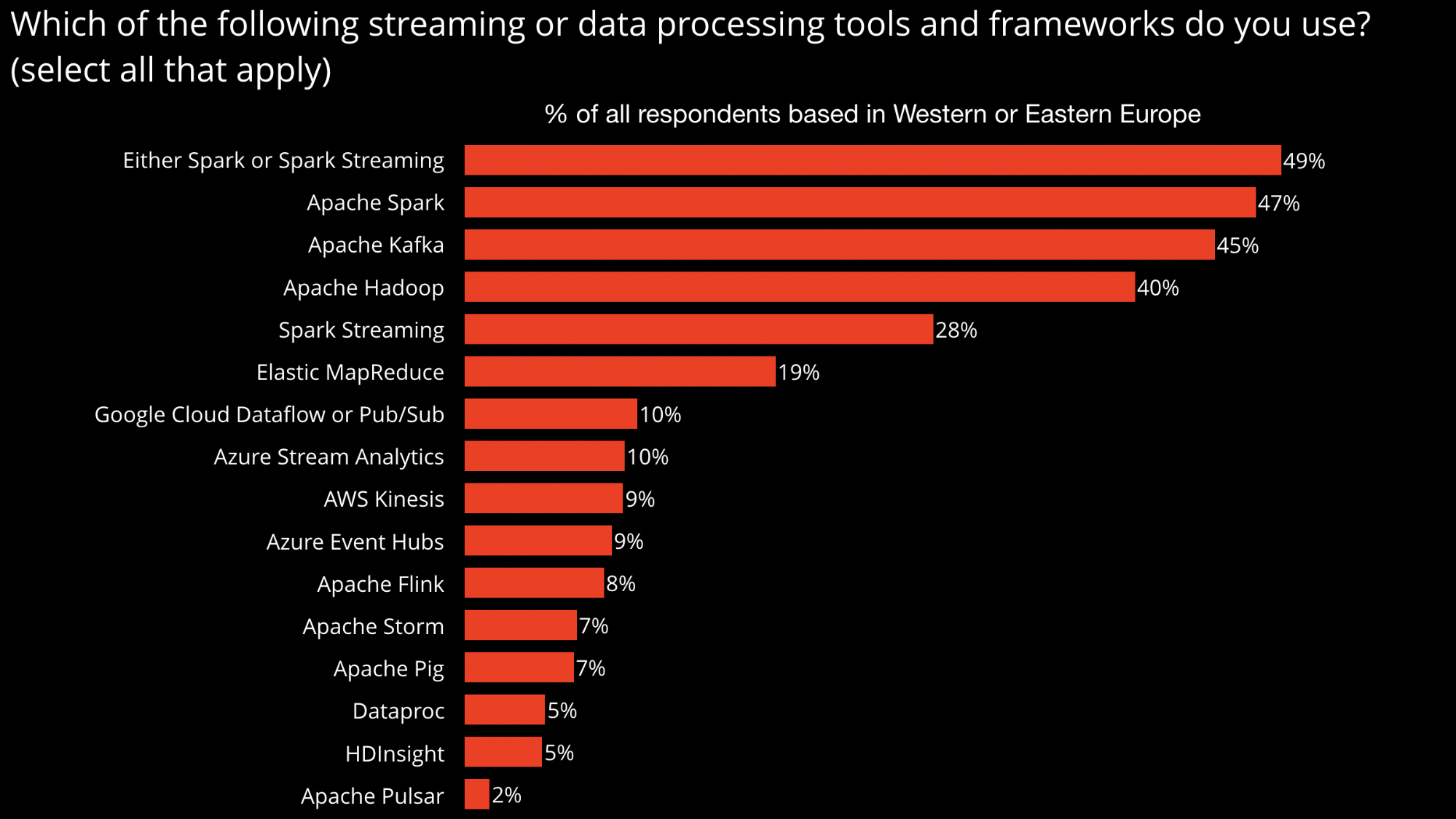
Many of the systems listed in the previous chart—Apache Spark, Kafka, Hadoop, etc.,—have been in use at enterprises across the globe for several years. One of the newer systems is Apache Pulsar, a promising new messaging system that unifies queuing and streaming. Pulsar will be covered in a popular new tutorial at Strata Data London, “Architecture and Algorithms for End-to-End Streaming Data Processing”. More importantly, there will be many sessions on the foundational technologies needed for machine learning and AI:
- AI and Data technologies in the cloud
- Data Platforms
- Data Integration and Data Pipelines
- Data preparation, data governance, and data lineage
- Machine Learning model lifecycle management
Our survey also aligned with recent articles describing the strong demand for data scientists. As noted above, ML and AI involves more than model building. Just as one needs a suite of technologies to sustain success in ML and AI, one also needs a team with a broad range of skills that go beyond model building. Not only is ML quite different from traditional software engineering, as noted in a previous post, ML is changing the nature of software development itself. The chart below lists demand for data-related skills in Europe:

The data science and machine learning program for Strata Data London will cover tools and methodologies, case studies and best practices, deep dives into familiar data types (text, temporal data, graphs), and new automation tools for data and machine learning professionals:
- AI and machine learning in the enterprise
- Deep Learning
- Text and Language processing and analysis
- Temporal data and time-series
- Automation in data science and big data
- Graph technologies and analytics
At the 2018 Strata Data London, data privacy and GDPR were big topics. In fact, our 2018 conference happened the same week GDPR came online. A year later, companies are still navigating through GDPR while also preparing for a new set of regulations (including the California Consumer Privacy Act). At this year’s conference, we will continue to have tutorials and sessions on data privacy and data security, but we will also have sessions on techniques and tools for privacy-preserving analytics—the very tools needed to build analytic and AI products that respect user privacy:
We are beginning to see interesting industrial IoT applications and systems. There’s good reason to expect that streaming and real-time applications will explode in the years to come. Tools and infrastructure for collecting streaming data have improved and continue to get easier to use. 5G mobile services are just around the corner and will pave the way for many new machine-to-machine applications. Since 5G increases the network bandwidth to mobile devices, it potentially will make it much more attractive to put machine learning at the edge of the network. Coincidentally, we are also beginning to see specialized hardware for intelligence on edge devices.
The good news is that companies are beginning to build foundational technologies (described in Figure 1) that will be essential in a world where the number of machine learning models and AI applications explode. The program at the Strata Data Conference in London will cover all these areas and more:
In an upcoming survey on the use of AI technologies (report forthcoming), we found that companies consider their inability to maintain a portfolio of use cases to be a major obstacle to AI adoption. At this year’s conference, we have presentations from leading companies detailing how they have successfully deployed data and machine learning technologies in real-world settings: