The evolution of data science, data engineering, and AI
The O’Reilly Data Show Podcast: A special episode to mark the 100th episode.
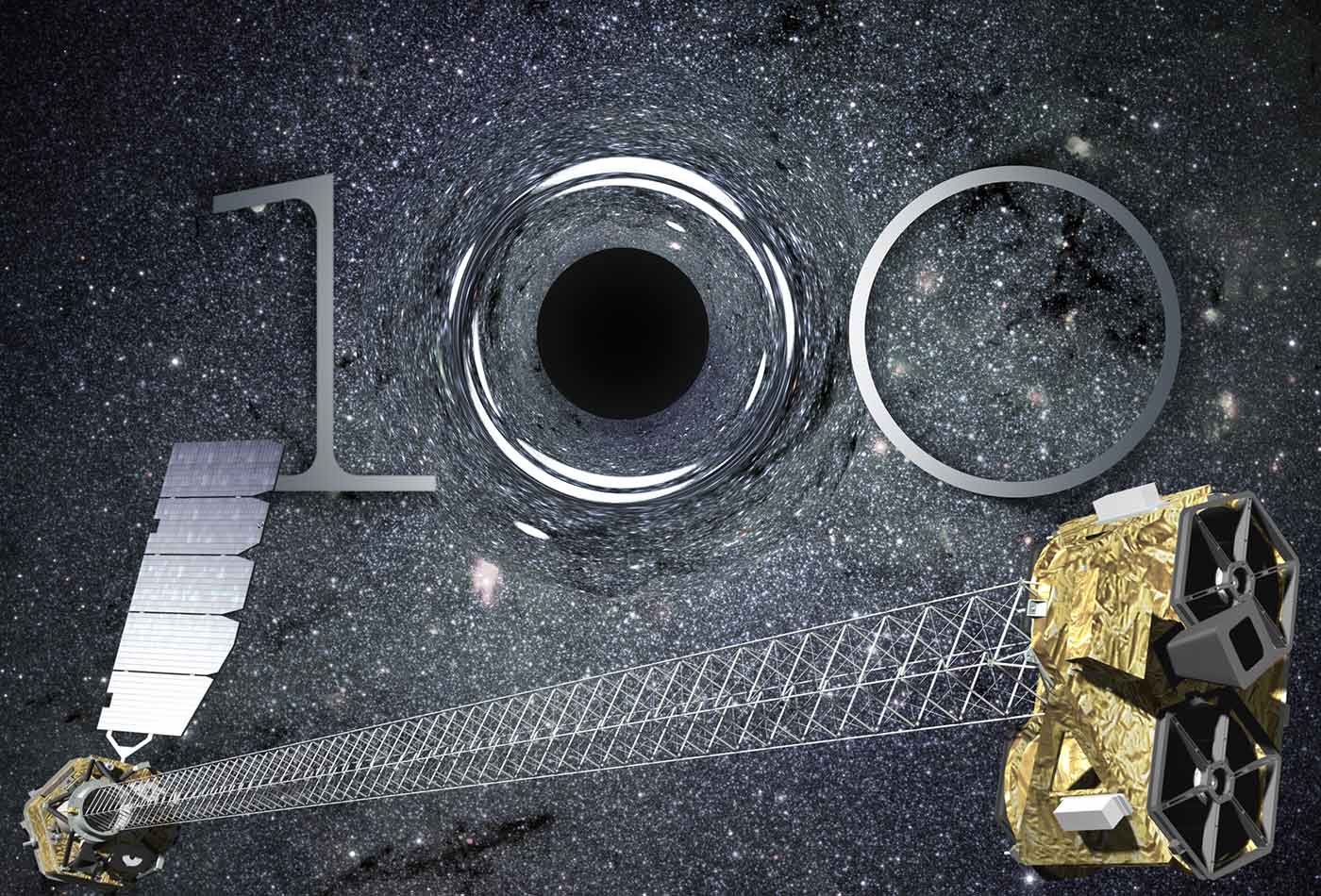
This episode of the Data Show marks our 100th episode. This podcast stemmed out of video interviews conducted at O’Reilly’s 2014 Foo Camp. We had a collection of friends who were key members of the data science and big data communities on hand and we decided to record short conversations with them. We originally conceived of using those initial conversations to be the basis of a regular series of video interviews. The logistics of studio interviews proved too complicated, but those Foo Camp conversations got us thinking about starting a podcast, and the Data Show was born.
To mark this milestone, my colleague Paco Nathan, co-chair of Jupytercon, turned the tables on me and asked me questions about previous Data Show episodes. In particular, we examined the evolution of key topics covered in this podcast: data science and machine learning, data engineering and architecture, AI, and the impact of each of these areas on businesses and companies. I’m proud of how this show has reached so many people across the world, and I’m looking forward to sharing more conversations in the future.
Here are some highlights from our conversation:
AI is more than machine learning
I think for many people machine learning is AI. I’m trying to, in the AI Conference series, convince people that a true AI system will involve many components, machine learning being one. Many of the guests I have seem to agree with that.
Evolving infrastructure for big data
In the early days of the podcast, many of the people I interacted with had Hadoop as one of the essential things in their infrastructure. I think while that might still be the case, there are more alternatives these days. I think a lot of people are going to object stores in the cloud. Another examples is that before, people maintained specialized systems. There’s still that, but people are trying to see if they can combine some of these systems, or come up with systems that can do more than one workload. For example, this whole notion in Spark of having a unified system that is able to do batch in streaming caught on during the span of this podcast.
Related resources: