Chapter 4. Manual Versus Automation
If you are still manually optimizing campaigns the same way it was done half a decade ago, you may be a quickly disappearing breed in the user acquisition space.
Let’s remember that machine learning is a type of artificial intelligence developed around allowing computer system to progressively improve performance on a task by “learning” through statistical approaches. Put another way, machine learning is the development of algorithms that allow for more and more accurate prediction with incremental collection of data. That is why Facebook, Google, and all the major media platforms are perfectly ripe for automation—the bigger your paid customer acquisition budget, the more data you can deliver into these machines to enable them to train and learn faster to help you hit your desired success goals.
The key question to ask is: why are you looking to automate something? Let’s remember that the two biggest challenges for startups are hiring people and acquiring new customers. The best way to tackle these challenges is to figure out how to run a Lean growth team without compromising on driving results.
Intelligent Machine Thinking in the World of Digital Marketing
Digital marketing has revolutionized advertising in the last three decades. From its humble beginnings in the early 1990s—fueled by hypertext, open source web servers, and crude browsers—digital media has grown into one of the top investment channels for marketers globally. The first commercial banner hit the web in 1994; by 1996 the industry had grown to $267 million in global media investment and showed no signs of slowing down. By 2017, global digital ad spend had reached more than $88 billion and grown at a 21% clip from the prior year.
Today, marketers can take advantage of search, display, video, and mobile advertising through advertising networks and related solution providers. Investment in these channels is fueled by increasing amounts of consumer data, sophistication, compute power, and more all aimed at improving results for advertisers and, ideally, creating a more personalized and relevant ad experiences in the consumer’s digital life.
The amount of digital media investment options available to marketers is endless, but more options and more data have required marketers to adopt increasingly sophisticated approaches to the space. As digital spend grew, larger companies hired digital media agencies to handle media planning and buying and worked those efforts into their “offline” media spending; agencies and smaller advertisers alike adopted business intelligence tools—referred to as dashboards—to help them analyze and optimize their client’s media spend across this growing swath of channels.
Advances in cloud computing, artificial intelligence, and machine learning present a new opportunity to digital marketers. They can now move past dashboards, reporting, and manual optimizations to improve the efficiency and effectiveness of their digital dollars, gain new insights based on behavioral patterns, and even generate new, personalized ads dynamically based on those evolving insights.
None of this is trivial, mind you. Major platform players like Google and Facebook have been working for years refining their own targeting algorithms, data-driven targeting capabilities, machine learning, and more to make their solutions more effective for advertisers. That’s great for individual platforms, but not necessarily so great for marketers or agencies, who are left to perform manual analysis and reporting to optimize spend between major platforms to meet their various objectives.
By 2015, a wave of innovation around neuro-linguistic programming (NLP),1 neural networks,2 and machine learning advances coincided with cheap, robust cloud computing infrastructure available on demand from vendors like Amazon (Amazon Web Services or AWS), Google (Google Cloud), and Microsoft (Azure). Meanwhile, digital media buying evolved from a transactional business into a highly scalable programmatic business designed to be interacted with through APIs.
The stage had now been set for the next transformation: to move digital media and marketing beyond the purely tactical into a world that’s more intuitive, highly automated, and more strategic than ever before.
Given all the promise and possibilities, what can automation and artificial intelligence really accomplish when applied to digital marketing efforts? There are several areas ripe for innovation.
Automated Media Buying
Machine learning is particularly well suited to making predictions when given a large amount of data. Major marketing platform providers like Google and Facebook use machine learning to deliver more relevant ad experiences to consumers and improve the performance of their offerings in an effort to get advertisers to spend more.
Advances in machine learning have given rise to independent software providers building out packaged solutions to save time for media buyers. They help advertisers take advantage of machine learning algorithms to improve their media buying efficiency without the overhead of building and maintaining custom-developed software on their own (an expensive proposition). These systems can adjust bid strategies to shift budgets around to better performing creatives and different customer segments to make ongoing performance improvements without manual intervention.
These systems are classified as Level 3 in our Marketing AI Autonomy scale (Table 2-2)—a step up from manual budget optimization and rules-based automation. These systems can save you time on everyday gruntwork and free you up to work on strategy, creatives, segmentation, and more.
Cross-Channel Marketing Orchestration
The next step up from automated media buying involves more complex systems that can work across multiple digital marketing platforms. Each major marketing platform (Google, Facebook, Twitter, Snapchat, etc.) offers different capabilities, APIs, and relative strengths and weaknesses given your specific marketing objectives.
Orchestration is a key concept in this class of autonomous marketing solutions. It goes far beyond automated bid management to take into account your marketing funnel, customer journey, or life cycle. Certain marketing platforms, for example, may be better at driving awareness among prospective customers or users. Others may be better at driving app installs or generating revenue. Systems that orchestrate marketing efforts with a more robust view of the customer journey encompass a host of benefits driven by AI and automation, particularly in the following areas:
-
Predicting behaviors across various channels with a unified view of the customer journey
-
Fine-tuning and perfecting cross-sell and upsell opportunities
-
Identifying the right channel to drive engagement based on reach and frequency modeling
Cross-channel marketing orchestration capable of Level 3 and Level 4 Marketing AI Automation are emerging classes of software, but they’re also increasingly within reach. Aspects of Level 3 and Level 4 Marketing AI Automation within a single channel are generally available and often offered as a feature of different major marketing platform providers.
Virtual Marketing Assistants
Voice-based interfaces to intelligent assistants like Amazon Alexa represent an area of growth and exploration in the marketing world. While most “assistants” are designed for consumer use or customer service applications, these intuitive voice interfaces hold promise in helping marketers better understand what’s happening in their digital media efforts, uncover trends, make adjustments, and take advantage of opportunities in new ways that would have been too laborious or complex using traditional user interfaces (UIs) or reporting dashboards. These emerging capabilities are on the leading edge of Level 4 and Level 5 Marketing AI Automation on our scale.
Content Curation
The amount of digital content available today is absolutely staggering. On Instagram alone, consumers publish 95 million images per day according to statistics from the company in February 2019. More than 72,000 GB of global internet traffic is moving around every second.
So what kind of content do your customers like? What resonates with your prospective customers? Artificial intelligence can help marketers sift through huge amounts of content to help them find out what their customers are spending their time consuming or engaging with. This can lead to ideas around what types of media outlets might be fruitful places to advertise. These insights can also fuel your content development, content marketing, and advertising efforts from a creative perspective.
Customer Support and Service
Today, chatbots serve as a first line of contact for routine customer support requests. According to Gartner research, 85% of all customer interactions will be handled without a human agent. The increased adoption of chat-based interfaces for customer service, marketing, shopping, and more serves both business and consumer interests. The volume, structure, and repetitive nature of routine service requests make automation highly approachable with off-the-shelf solutions that plug into common chat interfaces ranging from text messaging, Facebook Messenger, and beyond.
There are many great benefits of AI-powered customer service for businesses and consumers alike. For consumers, chat-based interfaces are accessible, feel familiar, and provide immediate responses to most common queries. It saves consumers time compared to wading through support lines, phone trees, and support queues, or waiting for customer support emails to get addressed and answered by a support agent.
Similarly, offloading the lion’s share of support requests to an AI-based agent can help improve the customer experience—and the company’s bottom line. IBM estimates that companies spend $1.3 trillion annually handling 265 billion customer support requests. Chatbots can help businesses save on customer service costs by speeding up response times, freeing up agents for more challenging work, and answering up to 80% of routine questions autonomously.
Businesses can further improve the customer experience by integrating chat-based customer service and support channels with their CRM systems and data management platforms. This type of integration allows a company to escalate your most valuable customers to the top of the service queue, for example to present retention-based offers to customers on the verge of lapsing or poised for an upgrade or new purchase based on their behavioral patterns.
Chat-based service and support interfaces are great sources of customer data for the Lean AI marketing automation framework.
Segmentation Development and Management
The amount of data coursing through the global internet at any given moment is nearly unfathomable. The big four alone—Amazon, Microsoft, Google, and Facebook—store upwards of 1.2 petabytes of data between them. That’s 1.2 million terabytes (a terabyte is 1,000 gigabytes). Trillions upon trillions of customer data points exist within this primordial data soup, ready to be accessed and pumped into the modern digital customer experience—personalized ads, offers, content, services, and more based on the newfound ability to anticipate customers’ needs and desires.
Marketers can tap their vast data stores to create unlimited customer segmentation models powered by artificial intelligence. Companies can already tap third-party data sources to enhance their customer records with tens of thousands of attributes, like household income, zip code, behaviors, and more. AI allows companies to take this to the next level by combining these conventional data attributes to a live stream of customer interactions, transaction data, product usage data, support and service data, and beyond.
Segmentation vendors are using AI to generate and update ever-evolving dynamic customer segments to feed into their execution systems to run precisely targeted campaigns across the customer’s user journey.
Insight Generation
Artificial intelligence can look through a mountain of behavioral data on a hyper-granular level to predict with great accuracy what a consumer will do next, based on their past behaviors and actions.
This is how ad platforms create lookalike audiences. Leveraging vast amounts of data and machine learning, these systems can easily cluster people based on behavioral attributes (or other factors) to anticipate their next move, motivations, and desires. If everyone in Cluster 1 takes actions A, B, and C then we can predict that customers who take actions A and B will likely follow that with action C.
By looking at audience behavior, AI systems find out the interests, context, and hedonistic activities around users and products. And the system automatically adapts with evolving consumer behaviors and interests. This can lead to new insights that can inform your strategy, creative approaches, offers, and much more. You can then take action on these insights and add them to your intelligent machine.
Creative Generation
Perhaps one of the most fascinating aspects of artificial intelligence today intersects with marketing technology in some potentially problematic (even dangerous) ways. Natural language generation—coding computers to write or generate written or spoken words in a way that can pass as human—has been a field of academic study for decades. Advances in recent years, particularly around recurring neural networks and their offshoots, have led to rapid improvements in natural language generation.
As it relates to marketing, applications around natural language generation could be used to analyze your marketing copy and create variations using your brand’s “voice.” This requires some training, but is well within the realm of possibility these days.
Natural language generation models have become so powerful that Open AI, a research company working on artificial general intelligence or AGI, refused to release the code related to its large-scale language model known as GPT2. The company cited concerns over misuse and abuse related to the “fake news” problem.
Which brings us to “deepfakes”—AI-generated video content that is nearly indistinguishable from the original content. The technique uses human image synthesis to combine and superimpose existing images and video onto source images or videos using machine learning. Marketing applications for the technology revolve around creative generation, personalization, and more, but fears of misuse related to fake news and even “revenge porn” make deepfake technology concerning in its ability to be weaponized by rogue actors and nation states.
Given the breadth of applications for artificial intelligence in growth marketing, it’s important to assess the ability for each to impact your most important outcomes. For example, training a neural network to generate copy variations based on a catalog of ads and customer service interactions might be interesting, but the cost, complexity, and time involved may outweigh any lift you might achieve with some fancy new AI-generated ad creatives.
Given the breadth of applications for artificial intelligence in growth marketing, it’s important to assess the ability for each to impact your most important outcomes.
When it comes to immediate impact with the AI-powered marketing technology just outlined, the following are your best bet:
-
Segmentation development and management
-
Automated media buying
-
Cross-channel marketing orchestration
-
Insight generation
These four interrelated disciplines all play a role in how you approach optimization today—with or without AI. They also fit nicely into a broader framework of a customer life cycle, which we will discuss next. And for any of the four disciplines just listed, AI offers a high enough “risk to reward” ratio to make the potential benefits of time and resources involved worth the cost and distraction factor.
Table Stakes: Customer Life Cycle Management
Many companies ignore customer life cycle management—much to their peril. They limit their focus on acquiring new customers, rather than retaining and “upselling” them over time. This approach to growth marketing is costly and unsustainable for a number of reasons:
-
Acquiring a new customer costs anywhere between 5 and 25 times more than retaining an existing customer (Harvard Business Review).
-
According to Bain & Company’s research, your existing customers spend 67% more than new customers.
-
You’re much more likely to get an existing customer to make a purchase (60% to 70% chance) compared to a new customer (5% to 20% chance of converting to a sale), according to reporting by ClickZ.
While a life cycle marketing approach can take a bit more thinking, the justification for orienting your growth team this way is clear. Not only is it more efficient, but your focus shifts from acquisition efforts to a more holistic view of your customer and their journey along the customer life cycle with your company. It’s a better experience for the customer and a much more rewarding relationship for your company.
Let’s think about a typical customer life cycle and how it lines up against the classic purchase funnel in Figure 4-1.
The purchase funnel is a great tool for developing a framework around the different types of content you’re going to need to develop to inspire a prospective customer to take the plunge and “convert” into becoming a new customer. We’ll dig deeper into the upper funnel later in Chapter 10 and Chapter 11, but for now, an overview in Figure 4-2 is helpful to set the stage for a life cycle marketing approach.
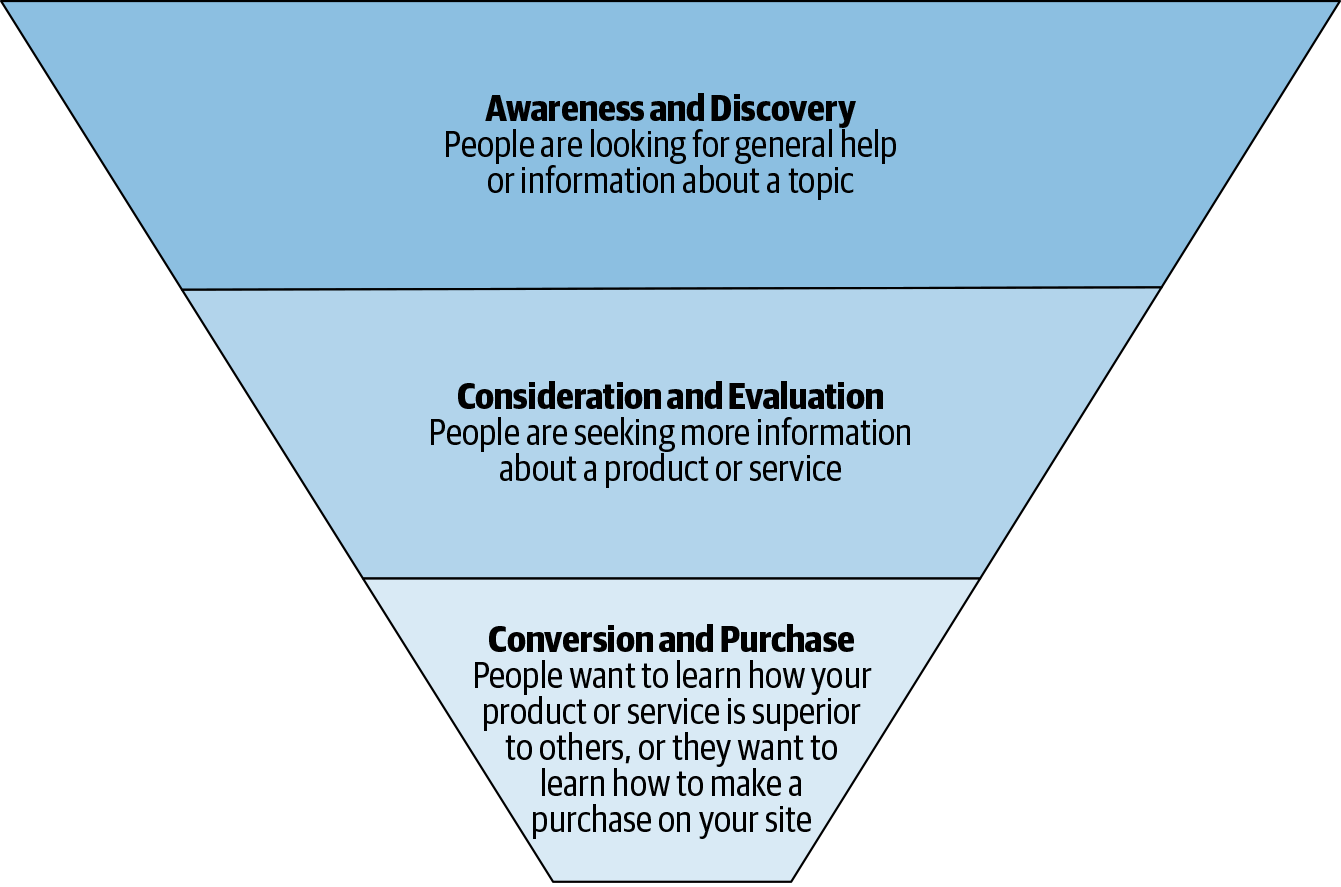
Figure 4-1. Classic customer purchase funnel
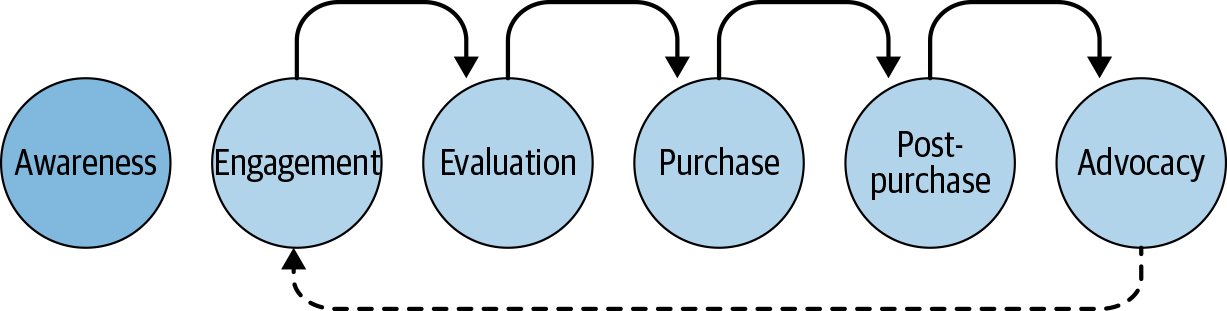
Figure 4-2. The different stages for a life cycle marketing approach funnel
Awareness
Awareness is the very top of the customer funnel: people can’t buy something they don’t know exists. Companies use mass media techniques like television, billboards, or social media to drive awareness. Editorial content, social posts, podcasts, and other types of engaging content work well for driving awareness and educating consumers on why they should care about what you’re offering.
The trick here is not to focus on awareness to the exclusion of the rest of the customer journey, a risk that leaves potentially willing prospects to find their own way through the rest of the funnel—this leads to significant drop-off from awareness to conversion, higher marketing costs, and alienating otherwise promising prospective customers.
While it’s clearly an important part of the marketing funnel, it’s important to remember that it’s just the beginning. You’ll need to figure out ways to engage the audience you’ve developed in your awareness phase, to begin walking them down the conversion funnel to becoming customers.
Engagement
Once you’ve built an audience of potential customers aware of your offering, you’ll need to keep them engaged. At this stage, the customer has a sense of what you’re offering and how it might fit into their daily lives.
In the engagement phase, your job is to get more specific with the customer on a few key points. Approach your content and creative development to ensure that it gives your prospects a reason to dig deeper into consideration. What are your top features? How do they compare with alternative offerings out there in the market that a prospect may be evaluating? Why should someone buy your product or service right now? Offers and discounts can be a good tactic to drive engagement, but don’t become over-reliant on them. It’s more likely at this stage that an offer won’t sway them one way or another, so the focus should be on educating prospects on the benefits you offer.
Evaluation
The evaluation stage sits at the bottom of your marketing funnel. It’s where your prospective customer is comparing your product offering to other options available. They’re reading reviews and comments to confirm that the choice they’re making is the right one. It’s important that your marketing content dives into great detail to ensure your prospects feel comfortable making a purchase commitment. Be sure to detail how your offering stacks up to competitive alternatives.
An offer or guarantee can help get someone over the final hurdle to making a purchase commitment. Test your calls to action and observe how they impact your customer lifetime value down the road.
Purchase
The purchase stage is self-evident. Making it easy to conduct a transaction is one critical point of optimization at this stage; the other is answering the question, “Why should I buy now?”
It’s at the purchase phase that a customer enters the next and often trickier stage of their life cycle marketing journey.
Post-Purchase
The post-purchase phase of marketing can be a creative challenge. Determining what types of content your new customers will find useful once they’ve made a purchase isn’t easy, particularly for certain types of products or services that have a limited life cycle or utility. Newsletters are the most common form of post-purchase marketing used today.
But AI holds tremendous potential in the post-purchase phase, thanks to the amount of transactional data that can be fed into our marketing engine. A few years back, triggered emails were the height of post-purchase CRM efforts. But today, companies aren’t limited to email for digital customer relationship management. Advanced segmentation techniques can feed targeting information to media partners for retention and activation marketing efforts in apps, on social, around the web, and beyond.
Advocacy
Activating your best customers and turning them into advocates can be a powerful technique for both deepening your customer relationships and improving your product or service offering. Brands that engage in customer advocacy marketing look for vocal customers who hold (and share) strong opinions about their products or services. Successful brands find ways to empower these advocates to make them feel connected to the company through loyalty programs, rewards for customer feedback or participating in focus groups, and other opportunities.
IMVU’s Strategy for Automating on the Growth Team
At IMVU, our growth team comprised several user acquisition managers, agencies, and consultants to help us manage our significant budget across different channels including mobile, paid search, SEO, mobile, display, affiliates, CRM, and retargeting. The biggest risk to our business was that our growth team was heavily dependent on humans and felt the impact of this especially with employee churn on the team. Most of the churn was related to the highly competitive job market in the San Francisco Bay Area where people working in user growth could easily job hop for more money.
A key insight that came from hiring and training new members on my team was that a lot of user acquisition tasks around managing and optimizing user acquisition campaigns were boring, repetitive tasks like changing bids, budgets, creative, running A/B testing, data analysis, and reporting. A lot of these tasks have logic and data as the central element, and as such machines could be trained to outperform humans in taking them on. That’s when the big “a-ha moment” hit me to solve this challenge by trying to figure out automation, so we could run a Lean team while applying technology to accomplish a lot of the tasks that are better managed by technology to scale up growth more efficiently.
Our approach to figure out what to consider for automation was as follows:
-
Identify all the human repetitive tasks being done to optimize our biggest paid channels
-
Calculate how much time was allocated in hours dedicated to each task
-
Order the tasks by time spent, budget, and impact on performance
-
Add a rank to each task that takes into consideration the time, complexity, and impact
-
Evaluate the ordered list to identify opportunities for automation and machine learning
When applying this approach to the growth team at IMVU, we initially decided to focus our automation efforts on our top two biggest paid channels—Facebook and Google—as those would have the biggest impact on the ROI of scaling up our user growth. The good news is that both of these partners are investing to provide more AI solutions and automation into their offerings for growth teams to make it easier to manage with less manual work.
Remember that machine learning is a type of artificial intelligence developed around allowing computer systems to progressively improve performance on a task by “learning” through statistical approaches. Put another way, machine learning is the development of algorithms that allow for more and more accurate prediction with incremental collection of data. That is why all these major media platforms are perfectly ripe for automation in user acquisition campaigns because the bigger your paid acquisition budget the more data you can deliver into these machines to enable them to train and learn faster to help you hit your desired success goals.
In 2018, both Facebook and Google Universal App Campaigns introduced ML algorithms that significantly improved the ability for advertisers to manage and scale up their campaigns based on success goals, with downstream optimizations becoming more easily managed using their native tools. The benefit is that now advertisers only have to focus on managing a few key levers like choosing the right optimization goals (CPI, CAC, ROAS), budgets, bids, and creative, leaving the time-consuming complex campaign optimization tasks in the hands of the powerful automated ML capabilities of Facebook and Google Universal App Campaigns.
I would envision Facebook and Google Universal App Campaigns algorithms getting even smarter with more accurate predictions for hitting KPIs (CPI, CAC, ROAS, and lifetime value [LTV]) and serving better personalized creative formats from incremental collection of data. This virtuous cycle will result in shifting even more budget toward the duopoly, which would impact the competitor’s ability to continue to invest significant R&D budget into further improving its own ML capabilities. What is still unknown is how much first-party data advertisers will need to continue to share with Facebook and Google Universal App Campaigns as these algorithms get better at predicting user intent with all the data signals they collect about users.
Building a Business Case for Automation
Most startups are resource-constrained and therefore need to develop a business case to determine which projects to prioritize based on their cost/benefit analysis compared to the success goals of the business. The challenge with new technology is always dealing with uncertainties to find the right data and costs to present in the business case. It’s important to clearly articulate the problems that leveraging AI/ML to automation would help solve. For example at IMVU the problem was to figure out how to better optimize our paid user acquisition budget across our key paid channels by accelerating our A/B testing to test new audience segments, creative, bids, and budgets at scale with real-time optimization that wasn’t possible with a Lean team. In our case at IMVU, we were able to project out the ROI of how much money we would save based on lowering the cost to acquire new users and hiring fewer user acquisition managers to manage these campaigns.
Most startups are resource-constrained and therefore need to develop a business case to determine which projects to prioritize.
In growth teams, you can determine your costs/benefits ROI formula using the following variables in your business case. The costs include:
-
In-house resources to support the project (engineering, data science, infrastructure, user acquisition managers, agencies, consultants, etc.). An example would be $100,000 per year on in-house resources to support the project.
-
Third-party AI/ML technology platforms (attribution, media buying, CRM, etc.). An example would be $200,000 per year for leveraging a third-party tool to support the project.
And the benefits include:
-
Efficiency improvement in CAC or ROAS. An example of projecting a 20% improvement in CAC on $20 would result in $4 savings per new payer, so if you project getting 100,000 new paying customers per year this would result in $400,000 savings in your acquisition budget.
-
Cost saving in hiring less people like user acquisition managers, consultants, or agencies to manage all the different paid channels that would be automated to enable a Lean growth team to scale up better with AI/ML automation. An example would be cutting costs of around $70,000 per year (which is the median salary for a user acquisition manager in the United States according to LinkedIn in April 2019).
To calculate ROI, the benefit (or return) of an investment is divided by the cost of the investment. The result is expressed as a percentage or a ratio.
The return on investment formula is:
ROI = (Current value of investment – Cost of investment) / Cost of investment
In this formula, “Current value of investment” refers to the proceeds obtained from the benefits of the investment. Because ROI is measured as a percentage, it can be easily compared with the returns from other investments, allowing one to measure a variety of types of investments against one another. In the example we’ve been looking at, our ROI would be:
ROI = ($470,000 - $300,000) / $300,000 = 57%
It is highly recommended to determine longer-term ROI projections over a three- to five-year timeframe. The goal should be achieving an ROI that remains positive over time and compounds that value by continuing to enrich growth team efficiencies and results. Because AI and ML automation systems need to be continually recalibrated and trained, contingencies or risks should always be factored into the ROI formula. As a rule of thumb, add a 15% cushion to your projected costs as a margin for the unexpected.
Now that we’ve mapped out the basics as they relate to customer life cycle marketing and automation, let’s start building out a framework for an autonomous marketing intelligent machine in the next chapter.
1 NLP is a psychological approach that involves analyzing strategies used by successful individuals and applying them to reach a personal goal. It relates thoughts, language, and patterns of behavior learned through experience to specific outcomes.
2 A neural network is a series of algorithms that endeavors to recognize underlying relationships in a data set through a process that mimics the way the human brain operates.
Get Lean AI now with the O’Reilly learning platform.
O’Reilly members experience books, live events, courses curated by job role, and more from O’Reilly and nearly 200 top publishers.