Chapter 1. Exploring the Landscape of Artificial Intelligence
Following are the words from Dr. May Carson’s (Figure 1-1) seminal paper on the changing role of artificial intelligence (AI) in human life in the twenty-first century:
Artificial Intelligence has often been termed as the electricity of the 21st century. Today, artificial intelligent programs will have the power to drive all forms of industry (including health), design medical devices and build new types of products and services, including robots and automobiles. As AI is advancing, organizations are already working to ensure those artificial intelligence programs can do their job and, importantly, avoid mistakes or dangerous accidents. Organizations need AI, but they also recognize that not everything they can do with AI is a good idea.
We have had extensive studies of what it takes to operate artificial intelligence using these techniques and policies. The main conclusion is that the amount of money spent on AI programs per person, per year versus the amount used to research, build and produce them is roughly equal. That seems obvious, but it’s not entirely true.
First, AI systems need support and maintenance to help with their functions. In order to be truly reliable, they need people to have the skills to run them and to help them perform some of their tasks. It’s essential that AI organizations provide workers to do the complex tasks needed by those services. It’s also important to understand the people who are doing those jobs, especially once AI is more complex than humans. For example, people will most often work in jobs requiring advanced knowledge but are not necessarily skilled in working with systems that need to be built and maintained.
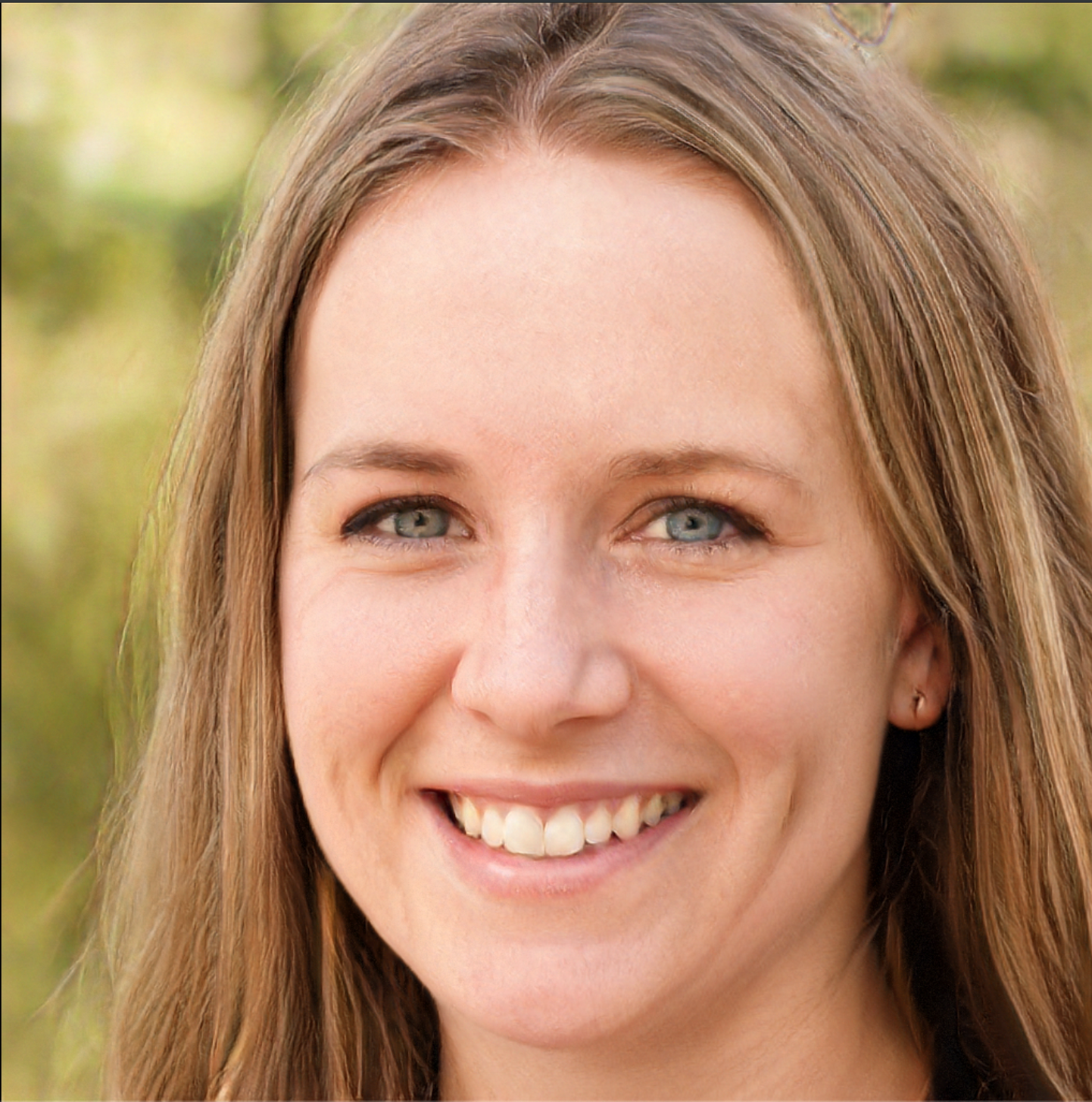
Figure 1-1. Dr. May Carson
An Apology
We now have to come clean and admit that everything in this chapter up to now was entirely fake. Literally everything! All of the text (other than the first italicized sentence, which was written by us as a seed) was generated using the GPT-2 model (built by Adam King) on the website TalkToTransformer.com. The name of the author was generated using the “Nado Name Generator” on the website Onitools.moe. At least the picture of the author must be real, right? Nope, the picture was generated from the website ThisPersonDoesNotExist.com which shows us new pictures of nonexistent people each time we reload the page using the magic of Generative Adversarial Networks (GANs).
Although we feel ambivalent, to say the least, about starting this entire book on a dishonest note, we thought it was important to showcase the state-of-the-art of AI when you, our reader, least expected it. It is, frankly, mind-boggling and amazing and terrifying at the same time to see what AI is already capable of. The fact that it can create sentences out of thin air that are more intelligent and eloquent than some world leaders is...let’s just say big league.
That being said, one thing AI can’t appropriate from us just yet is the ability to be fun. We’re hoping that those first three fake paragraphs will be the driest in this entire book. After all, we don’t want to be known as “the authors more boring than a machine.”
The Real Introduction
Recall that time you saw a magic show during which a trick dazzled you enough to think, “How the heck did they do that?!” Have you ever wondered the same about an AI application that made the news? In this book, we want to equip you with the knowledge and tools to not only deconstruct but also build a similar one.
Through accessible, step-by-step explanations, we dissect real-world applications that use AI and showcase how you would go about creating them on a wide variety of platforms—from the cloud to the browser to smartphones to edge AI devices, and finally landing on the ultimate challenge currently in AI: autonomous cars.
In most chapters, we begin with a motivating problem and then build an end-to-end solution one step at a time. In the earlier portions of the book, we develop the necessary skills to build the brains of the AI. But that’s only half the battle. The true value of building AI is in creating usable applications. And we’re not talking about toy prototypes here. We want you to construct software that can be used in the real world by real people for improving their lives. Hence, the word “Practical” in the book title. To that effect, we discuss various options that are available to us and choose the appropriate options based on performance, energy consumption, scalability, reliability, and privacy trade-offs.
In this first chapter, we take a step back to appreciate this moment in AI history. We explore the meaning of AI, specifically in the context of deep learning and the sequence of events that led to deep learning becoming one of the most groundbreaking areas of technological progress in the early twenty-first century. We also examine the core components underlying a complete deep learning solution, to set us up for the subsequent chapters in which we actually get our hands dirty.
So our journey begins here, with a very fundamental question.
What Is AI?
Throughout this book, we use the terms “artificial intelligence,” “machine learning,” and “deep learning” frequently, sometimes interchangeably. But in the strictest technical terms, they mean different things. Here’s a synopsis of each (see also Figure 1-2):
- AI
-
This gives machines the capabilities to mimic human behavior. IBM’s Deep Blue is a recognizable example of AI.
- Machine learning
-
This is the branch of AI in which machines use statistical techniques to learn from previous information and experiences. The goal is for the machine to take action in the future based on learning observations from the past. If you watched IBM’s Watson take on Ken Jennings and Brad Rutter on Jeopardy!, you saw machine learning in action. More relatably, the next time a spam email doesn’t reach your inbox, you can thank machine learning.
- Deep learning
-
This is a subfield of machine learning in which deep, multilayered neural networks are used to make predictions, especially excelling in computer vision, speech recognition, natural language understanding, and so on.
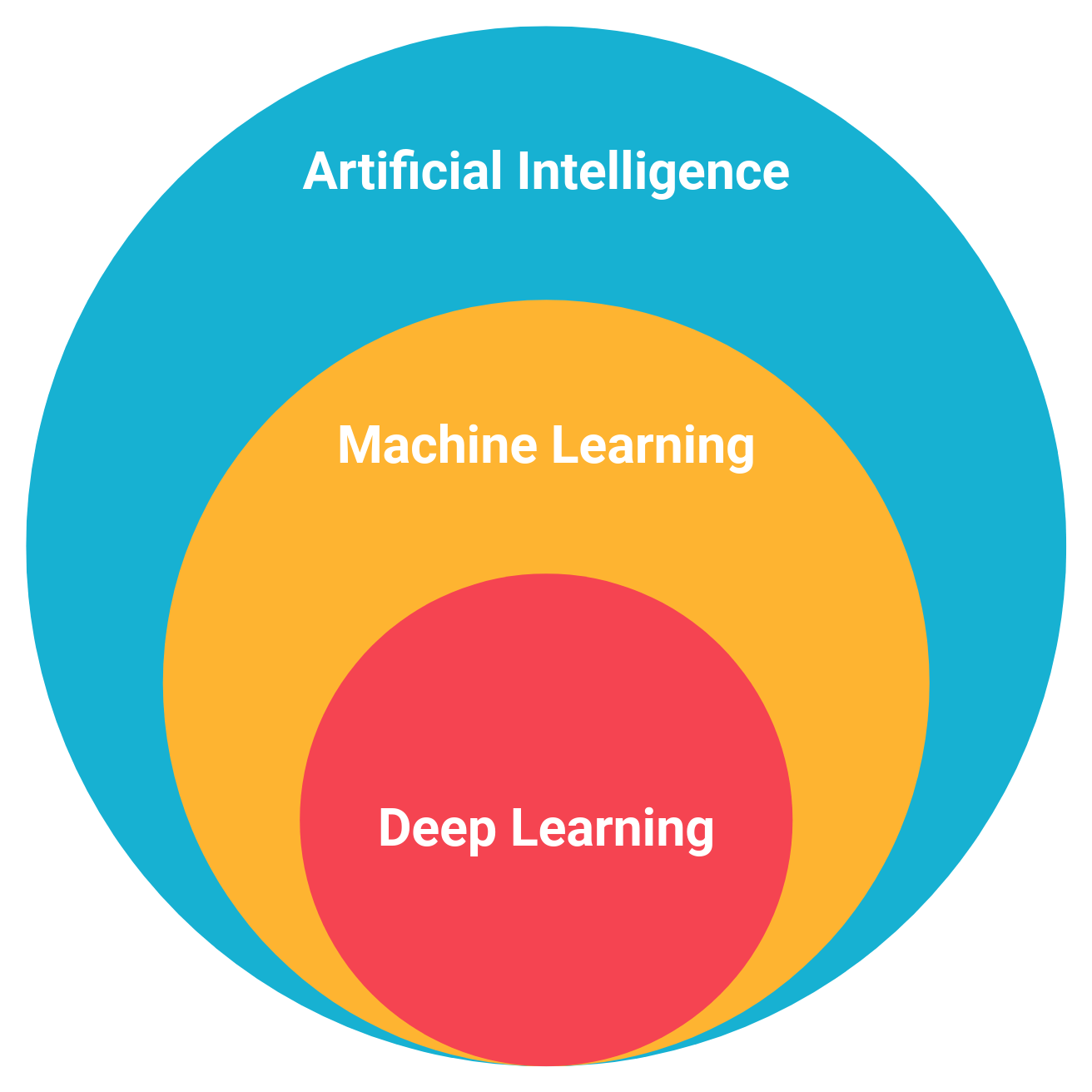
Figure 1-2. The relationship between AI, machine learning, and deep learning
Throughout this book, we primarily focus on deep learning.
Motivating Examples
Let’s cut to the chase. What compelled us to write this book? Why did you spend your hard-earned money1 buying this book? Our motivation was simple: to get more people involved in the world of AI. The fact that you’re reading this book means that our job is already halfway done.
However, to really pique your interest, let’s take a look at some stellar examples that demonstrate what AI is already capable of doing:
-
“DeepMind’s AI agents conquer human pros at StarCraft II”: The Verge, 2019
-
“AI-Generated Art Sells for Nearly Half a Million Dollars at Christie’s”: AdWeek, 2018
-
“AI Beats Radiologists in Detecting Lung Cancer”: American Journal of Managed Care, 2019
-
“Boston Dynamics Atlas Robot Can Do Parkour”: ExtremeTech, 2018
-
“Facebook, Carnegie Mellon build first AI that beats pros in 6-player poker”: ai.facebook.com, 2019
-
“Blind users can now explore photos by touch with Microsoft’s Seeing AI”: TechCrunch, 2019
-
“IBM’s Watson supercomputer defeats humans in final Jeopardy match”: VentureBeat, 2011
-
“Google’s ML-Jam challenges musicians to improvise and collaborate with AI”: VentureBeat, 2019
-
“Mastering the Game of Go without Human Knowledge”: Nature, 2017
-
“Chinese AI Beats Doctors in Diagnosing Brain Tumors”: Popular Mechanics, 2018
-
“Two new planets discovered using artificial intelligence”: Phys.org, 2019
-
“Nvidia’s latest AI software turns rough doodles into realistic landscapes”: The Verge, 2019
These applications of AI serve as our North Star. The level of these achievements is the equivalent of a gold-medal-winning Olympic performance. However, applications solving a host of problems in the real world is the equivalent of completing a 5K race. Developing these applications doesn’t require years of training, yet doing so provides the developer immense satisfaction when crossing the finish line. We are here to coach you through that 5K.
Throughout this book, we intentionally prioritize breadth. The field of AI is changing so quickly that we can only hope to equip you with the proper mindset and array of tools. In addition to tackling individual problems, we will look at how different, seemingly unrelated problems have fundamental overlaps that we can use to our advantage. As an example, sound recognition uses Convolutional Neural Networks (CNNs), which are also the basis for modern computer vision. We touch upon practical aspects of multiple areas so you will be able to go from 0 to 80 quickly to tackle real-world problems. If we’ve generated enough interest that you decide you then want to go from 80 to 95, we’d consider our goal achieved. As the oft-used phrase goes, we want to “democratize AI.”
It’s important to note that much of the progress in AI happened in just the past few years—it’s difficult to overstate that. To illustrate how far we’ve come along, take this example: five years ago, you needed a Ph.D. just to get your foot in the door of the industry. Five years later, you don’t even need a Ph.D. to write an entire book on the subject. (Seriously, check our profiles!)
Although modern applications of deep learning seem pretty amazing, they did not get there all on their own. They stood on the shoulders of many giants of the industry who have been pushing the limits for decades. Indeed, we can’t fully appreciate the significance of this time without looking at the entire history.
A Brief History of AI
Let’s go back in time a little bit: our whole universe was in a hot dense state. Then nearly 14 billion years ago expansion started, wait...okay, we don’t have to go back that far (but now we have the song stuck in your head for the rest of the day, right?). It was really just 70 years ago when the first seeds of AI were planted. Alan Turing, in his 1950 paper, “Computing Machinery and Intelligence,” first asked the question “Can machines think?” This really gets into a larger philosophical debate of sentience and what it means to be human. Does it mean to possess the ability to compose a concerto and know that you’ve composed it? Turing found that framework rather restrictive and instead proposed a test: if a human cannot distinguish a machine from another human, does it really matter? An AI that can mimic a human is, in essence, human.
Exciting Beginnings
The term “artificial intelligence” was coined by John McCarthy in 1956 at the Dartmouth Summer Research Project. Physical computers weren’t even really a thing back then, so it’s remarkable that they were able to discuss futuristic areas such as language simulation, self-improving learning machines, abstractions on sensory data, and more. Much of it was theoretical, of course. This was the first time that AI became a field of research rather than a single project.
The paper “Perceptron: A Perceiving and Recognizing Automaton” in 1957 by Frank Rosenblatt laid the foundation for deep neural networks. He postulated that it should be feasible to construct an electronic or electromechanical system that will learn to recognize similarities between patterns of optical, electrical, or tonal information. This system would function similar to the human brain. Rather than using a rule-based model (which was standard for the algorithms at the time), he proposed using statistical models to make predictions.
Throughout this book, we repeat the phrase neural network. What is a neural network? It is a simplified model of the human brain. Much like the brain, it has neurons that activate when encountering something familiar. The different neurons are connected via connections (corresponding to synapses in our brain) that help information flow from one neuron to another.
In Figure 1-3, we can see an example of the simplest neural network: a perceptron. Mathematically, the perceptron can be expressed as follows:
output = f(x1, x2, x3) = x1 w1 + x2 w2 + x3 w3 + b
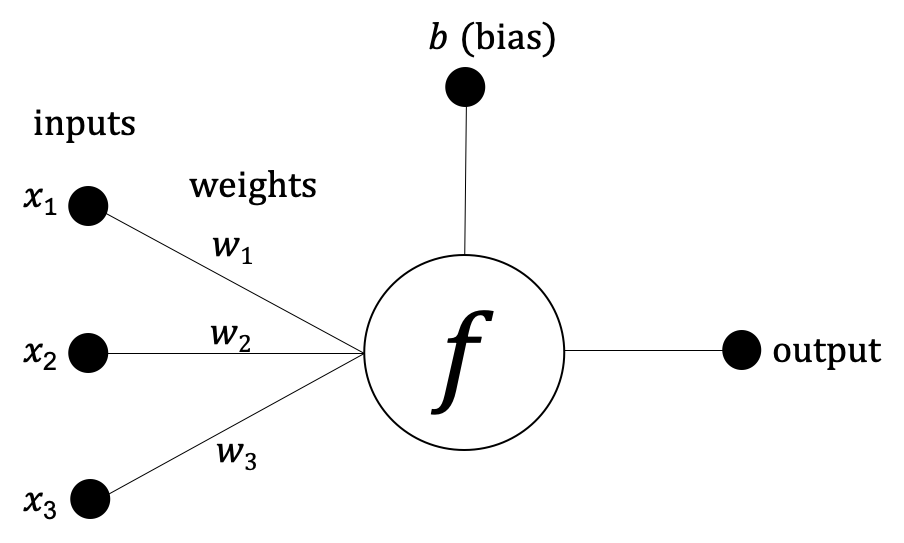
Figure 1-3. An example of a perceptron
In 1965, Ivakhnenko and Lapa published the first working neural network in their paper “Group Method of Data Handling—A Rival Method of Stochastic Approximation.” There is some controversy in this area, but Ivakhnenko is regarded by some as the father of deep learning.
Around this time, bold predictions were made about what machines would be capable of doing. Machine translation, speech recognition, and more would be performed better than humans. Governments around the world were excited and began opening up their wallets to fund these projects. This gold rush started in the late 1950s and was alive and well into the mid-1970s.
The Cold and Dark Days
With millions of dollars invested, the first systems were put into practice. It turned out that a lot of the original prophecies were unrealistic. Speech recognition worked only if it was spoken in a certain way, and even then, only for a limited set of words. Language translation turned out to be heavily erroneous and much more expensive than what it would cost a human to do. Perceptrons (essentially single-layer neural networks) quickly hit a cap for making reliable predictions. This limited their usefulness for most problems in the real world. This is because they are linear functions, whereas problems in the real world often require a nonlinear classifier for accurate predictions. Imagine trying to fit a line to a curve!
So what happens when you over-promise and under-deliver? You lose funding. The Defense Advanced Research Project Agency, commonly known as DARPA (yeah, those people; the ones who built the ARPANET, which later became the internet), funded a lot of the original projects in the United States. However, the lack of results over nearly two decades increasingly frustrated the agency. It was easier to land a man on the moon than to get a usable speech recognizer!
Similarly, across the pond, the Lighthill Report was published in 1974, which said, “The general-purpose robot is a mirage.” Imagine being a Brit in 1974 watching the bigwigs in computer science debating on the BBC as to whether AI is a waste of resources. As a consequence, AI research was decimated in the United Kingdom and subsequently across the world, destroying many careers in the process. This phase of lost faith in AI lasted about two decades and came to be known as the “AI Winter.” If only Ned Stark had been around back then to warn them.
A Glimmer of Hope
Even during those freezing days, there was some groundbreaking work done in this field. Sure, perceptrons—being linear functions—had limited capabilities. How could one fix that? By chaining them in a network, such that the output of one (or more) perceptron is the input to one (or more) perceptron. In other words, a multilayer neural network, as illustrated in Figure 1-4. The higher the number of layers, the more the nonlinearity it would learn, resulting in better predictions. There is just one issue: how does one train it? Enter Geoffrey Hinton and friends. They published a technique called backpropagation in 1986 in the paper “Learning representations by back-propagating errors.” How does it work? Make a prediction, see how far off the prediction is from reality, and propagate back the magnitude of the error into the network so it can learn to fix it. You repeat this process until the error becomes insignificant. A simple yet powerful concept. We use the term backpropagation repeatedly throughout this book.
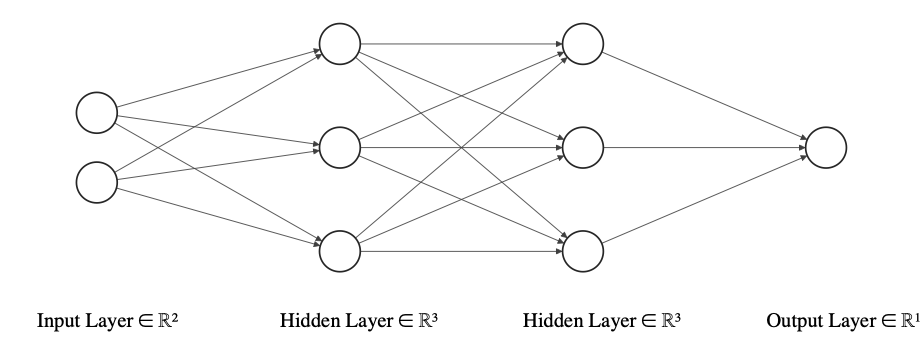
Figure 1-4. An example multilayer neural network (image source)
In 1989, George Cybenko provided the first proof of the Universal Approximation Theorem, which states that a neural network with a single hidden layer is theoretically capable of modeling any problem. This was remarkable because it meant that neural networks could (at least in theory) outdo any machine learning approach. Heck, it could even mimic the human brain. But all of this was only on paper. The size of this network would quickly pose limitations in the real world. This could be overcome somewhat by using multiple hidden layers and training the network with…wait for it…backpropagation!
On the more practical side of things, a team at Carnegie Mellon University built the first-ever autonomous vehicle, NavLab 1, in 1986 (Figure 1-5). It initially used a single-layer neural network to control the angle of the steering wheel. This eventually led to NavLab 5 in 1995. During a demonstration, a car drove all but 50 of the 2,850-mile journey from Pittsburgh to San Diego on its own. NavLab got its driver’s license before many Tesla engineers were even born!
Another standout example from the 1980s was at the United States Postal Service (USPS). The service needed to sort postal mail automatically according to the postal codes (ZIP codes) they were addressed to. Because a lot of the mail has always been handwritten, optical character recognition (OCR) could not be used. To solve this problem, Yann LeCun et al. used handwritten data from the National Institute of Standards and Technology (NIST) to demonstrate that neural networks were capable of recognizing these handwritten digits in their paper “Backpropagation Applied to Handwritten Zip Code Recognition.” The agency’s network, LeNet, became what the USPS used for decades to automatically scan and sort the mail. This was remarkable because it was the first convolutional neural network that really worked in the wild. Eventually, in the 1990s, banks would use an evolved version of the model called LeNet-5 to read handwritten numbers on checks. This laid the foundation for modern computer vision.
Those of you who have read about the MNIST dataset might have already noticed a connection to the NIST mention we just made. That is because the MNIST dataset essentially consists of a subset of images from the original NIST dataset that had some modifications (the “M” in “MNIST”) applied to them to ease the train and test process for the neural network. Modifications, some of which you can see in Figure 1-6, included resizing them to 28 x 28 pixels, centering the digit in that area, antialiasing, and so on.
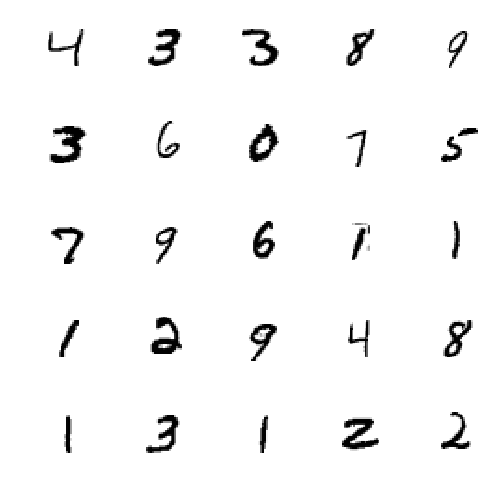
Figure 1-6. A sample of handwritten digits from the MNIST dataset
A few others kept their research going, including Jürgen Schmidhuber, who proposed networks like the Long Short-Term Memory (LSTM) with promising applications for text and speech.
At that point, even though the theories were becoming sufficiently advanced, results could not be demonstrated in practice. The main reason was that it was too computationally expensive for the hardware back then and scaling them for larger tasks was a challenge. Even if by some miracle the hardware was available, the data to realize its full potential was certainly not easy to come by. After all, the internet was still in its dial-up phase. Support Vector Machines (SVMs), a machine learning technique introduced for classification problems in 1995, were faster and provided reasonably good results on smaller amounts of data, and thus had become the norm.
As a result, AI and deep learning’s reputation was poor. Graduate students were warned against doing deep learning research because this is the field “where smart scientists would see their careers end.” People and companies working in the field would use alternative words like informatics, cognitive systems, intelligent agents, machine learning, and others to dissociate themselves from the AI name. It’s a bit like when the U.S. Department of War was rebranded as the Department of Defense to be more palatable to the people.
How Deep Learning Became a Thing
Luckily for us, the 2000s brought high-speed internet, smartphone cameras, video games, and photo-sharing sites like Flickr and Creative Commons (bringing the ability to legally reuse other people’s photos). People in massive numbers were able to quickly take photos with a device in their pockets and then instantly upload. The data lake was filling up, and gradually there were ample opportunities to take a dip. The 14 million-image ImageNet dataset was born from this happy confluence and some tremendous work by (then Princeton’s) Fei-Fei Li and company.
During the same decade, PC and console gaming became really serious. Gamers demanded better and better graphics from their video games. This, in turn, pushed Graphics Processing Unit (GPU) manufacturers such as NVIDIA to keep improving their hardware. The key thing to remember here is that GPUs are damn good at matrix operations. Why is that the case? Because the math demands it! In computer graphics, common tasks such as moving objects, rotating them, changing their shape, adjusting their lighting, and so on all use matrix operations. And GPUs specialize in doing them. And you know what else needs a lot of matrix calculations? Neural networks. It’s one big happy coincidence.
With ImageNet ready, the annual ImageNet Large Scale Visual Recognition Challenge (ILSVRC) was set up in 2010 to openly challenge researchers to come up with better techniques for classifying this data. A subset of 1,000 categories consisting of approximately 1.2 million images was available to push the boundaries of research. The state-of-the-art computer-vision techniques like Scale-Invariant Feature Transform (SIFT) + SVM yielded a 28% (in 2010) and a 25% (2011) top-5 error rate (i.e., if one of the top five guesses ranked by probability matches, it’s considered accurate).
And then came 2012, with an entry on the leaderboard that nearly halved the error rate down to 16%. Alex Krizhevsky, Ilya Sutskever (who eventually founded OpenAI), and Geoffrey Hinton from the University of Toronto submitted that entry. Aptly called AlexNet, it was a CNN that was inspired by LeNet-5. Even at just eight layers, AlexNet had a massive 60 million parameters and 650,000 neurons, resulting in a 240 MB model. It was trained over one week using two NVIDIA GPUs. This single event took everyone by surprise, proving the potential of CNNs that snowballed into the modern deep learning era.
Figure 1-7 quantifies the progress that CNNs have made in the past decade. We saw a 40% year-on-year decrease in classification error rate among ImageNet LSVRC–winning entries since the arrival of deep learning in 2012. As CNNs grew deeper, the error continued to decrease.
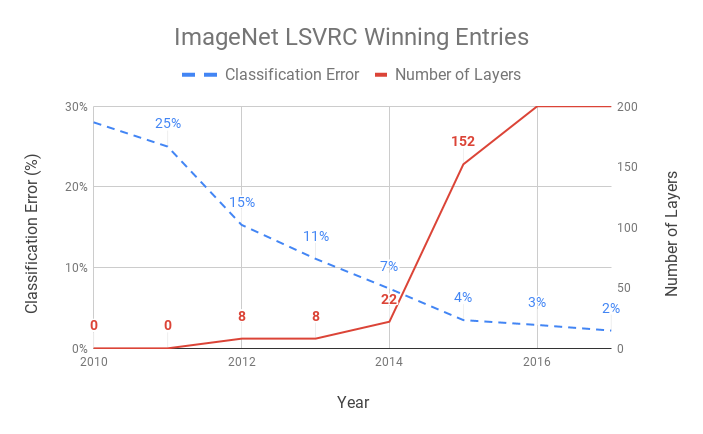
Figure 1-7. Evolution of winning entries at ImageNet LSVRC
Keep in mind we are vastly simplifying the history of AI, and we are surely glossing over some of the details. Essentially, it was a confluence of data, GPUs, and better techniques that led to this modern era of deep learning. And the progress kept expanding further into newer territories. As Table 1-1 highlights, what was in the realm of science fiction is already a reality.
2012 | Neural network from Google Brain team starts recognizing cats after watching YouTube videos |
2013 |
|
2014 |
|
2015 |
|
2016 |
|
2017 |
|
2018 |
|
2019 |
|
Hopefully, you now have a historical context of AI and deep learning and have an understanding of why this moment in time is significant. It’s important to recognize the rapid rate at which progress is happening in this area. But as we have seen so far, this was not always the case.
The original estimate for achieving real-world computer vision was “one summer” back in the 1960s, according to two of the field’s pioneers. They were off by only half a century! It’s not easy being a futurist. A study by Alexander Wissner-Gross observed that it took 18 years on average between when an algorithm was proposed and the time it led to a breakthrough. On the other hand, that gap was a mere three years on average between when a dataset was made available and the breakthrough it helped achieve! Look at any of the breakthroughs in the past decade. The dataset that enabled that breakthrough was very likely made available just a few years prior.
Data was clearly the limiting factor. This shows the crucial role that a good dataset can play for deep learning. However, data is not the only factor. Let’s look at the other pillars that make up the foundation of the perfect deep learning solution.
Recipe for the Perfect Deep Learning Solution
Before Gordon Ramsay starts cooking, he ensures he has all of the ingredients ready to go. The same goes for solving a problem using deep learning (Figure 1-8).
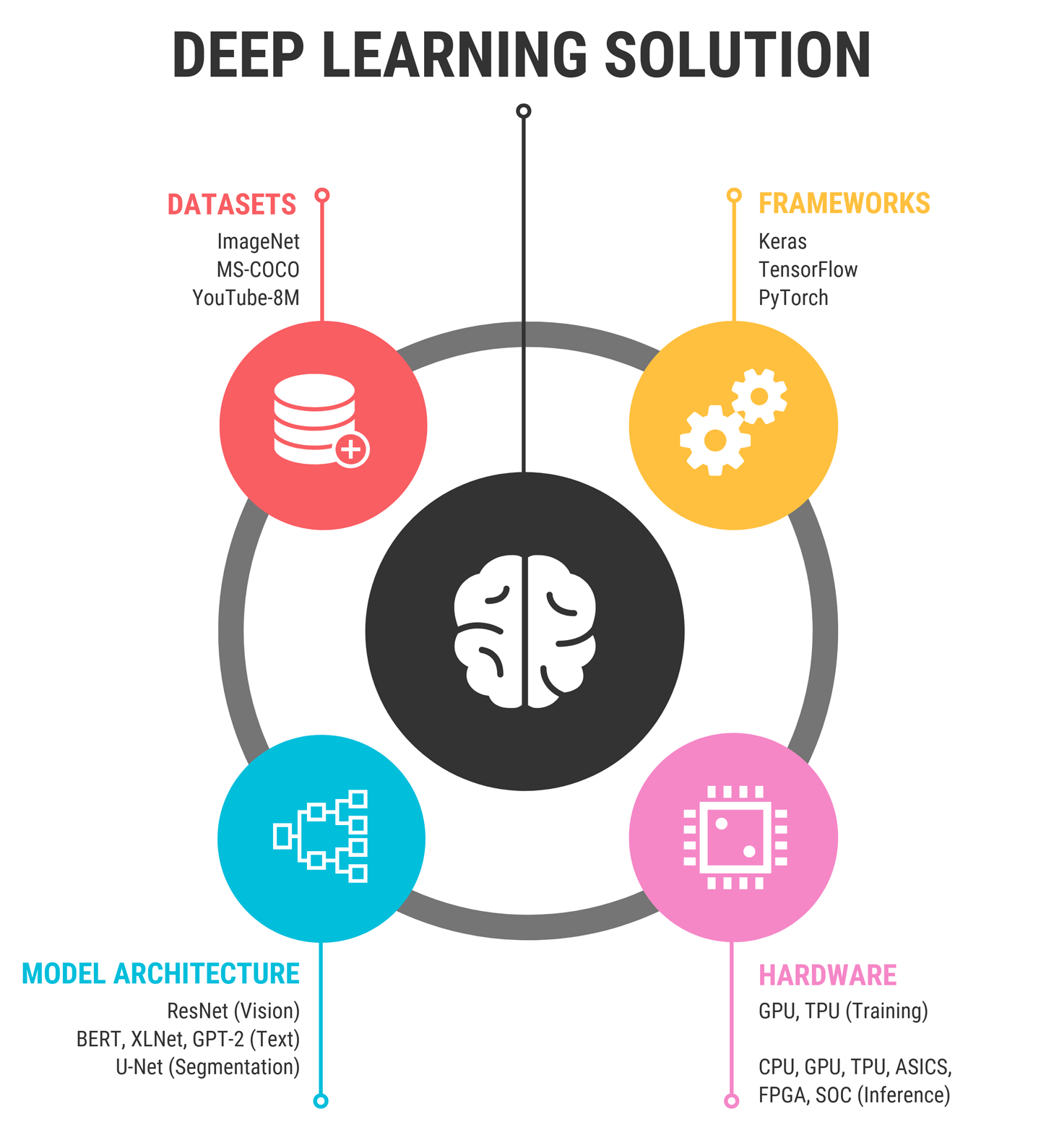
Figure 1-8. Ingredients for the perfect deep learning solution
And here’s your deep learning mise en place!
Dataset + Model + Framework + Hardware = Deep Learning Solution
Let’s look into each of these in a little more detail.
Datasets
Just like Pac-Man is hungry for dots, deep learning is hungry for data—lots and lots of data. It needs this amount of data to spot meaningful patterns that can help make robust predictions. Traditional machine learning was the norm in the 1980s and 1990s because it would function even with few hundreds to thousands of examples. In contrast, Deep Neural Networks (DNNs), when built from scratch, would need orders more data for typical prediction tasks. The upside here is far better predictions.
In this century, we are having a data explosion with quintillions of bytes of data being created every single day—images, text, videos, sensor data, and more. But to make effective use of this data, we need labels. To build a sentiment classifier to know whether an Amazon review is positive or negative, we need thousands of sentences and an assigned emotion for each. To train a face segmentation system for a Snapchat lens, we need the precise location of eyes, lips, nose, and so forth on thousands of images. To train a self-driving car, we need video segments labeled with the human driver’s reactions on controls such as the brakes, accelerator, steering wheel, and so forth. These labels act as teachers to our AI and are far more valuable than unlabeled data alone.
Getting labels can be pricey. It’s no wonder that there is an entire industry around crowdsourcing labeling tasks among thousands of workers. Each label might cost from a few cents to dollars, depending on the time spent by the workers to assign it. For example, during the development of the Microsoft COCO (Common Objects in Context) dataset, it took roughly three seconds to label the name of each object in an image, approximately 30 seconds to place a bounding box around each object, and 79 seconds to draw the outlines for each object. Repeat that hundreds of thousands of times and you can begin to fathom the costs around some of the larger datasets. Some labeling companies like Appen and Scale AI are already valued at more than a billion dollars each.
We might not have a million dollars in our bank account. But luckily for us, two good things happened in this deep learning revolution:
-
Gigantic labeled datasets have been generously made public by major companies and universities.
-
A technique called transfer learning, which allows us to tune our models to datasets with even hundreds of examples—as long as our model was originally trained on a larger dataset similar to our current set. We use this repeatedly in the book, including in Chapter 5 where we experiment and prove even a few tens of examples can get us decent performance with this technique. Transfer learning busts the myth that big data is necessary for training a good model. Welcome to the world of tiny data!
Table 1-2 showcases some of the popular datasets out there today for a variety of deep learning tasks.
Data type | Name | Details |
---|---|---|
Image |
Open Images V4 (from Google) |
|
Microsoft COCO |
|
|
Video | YouTube-8M |
|
Video, images |
BDD100K (from UC Berkeley) |
|
Waymo Open Dataset | 3,000 driving scenes totaling 16.7 hours of video data, 600,000 frames, approximately 25 million 3D bounding boxes, and 22 million 2D bounding boxes | |
Text | SQuAD | 150,000 Question and Answer snippets from Wikipedia |
Yelp Reviews | Five million Yelp reviews | |
Satellite data | Landsat Data | Several million satellite images (100 nautical mile width and height), along with eight spectral bands (15- to 60-meter spatial resolution) |
Audio |
Google AudioSet | 2,084,320 10-second sound clips from YouTube with 632 categories |
LibriSpeech | 1,000 hours of read English speech |
Model Architecture
At a high level, a model is just a function. It takes in one or more inputs and gives an output. The input might be in the form of text, images, audio, video, and more. The output is a prediction. A good model is one whose predictions reliably match the expected reality. The model’s accuracy on a dataset is a major determining factor as to whether it’s suitable for use in a real-world application. For many people, this is all they really need to know about deep learning models. But it’s when we peek into the inner workings of a model that it becomes really interesting (Figure 1-9).

Figure 1-9. A black box view of a deep learning model
Inside the model is a graph that consists of nodes and edges. Nodes represent mathematical operations, whereas edges represent how the data flows from one node to another. In other words, if the output of one node can become the input to one or more nodes, the connections between those nodes are represented by edges. The structure of this graph determines the potential for accuracy, its speed, how much resources it consumes (memory, compute, and energy), and the type of input it’s capable of processing.
The layout of the nodes and edges is known as the architecture of the model. Essentially, it’s a blueprint. Now, the blueprint is only half the picture. We still need the actual building. Training is the process that utilizes this blueprint to construct that building. We train a model by repeatedly 1) feeding it input data, 2) getting outputs from it, 3) monitoring how far these predictions are from the expected reality (i.e., the labels associated with the data), and then, 4) propagating the magnitude of error back to the model so that it can progressively learn to correct itself. This training process is performed iteratively until we are satisfied with the accuracy of the predictions.
The result from this training is a set of numbers (also known as weights) that is assigned to each of the nodes. These weights are necessary parameters for the nodes in the graph to operate on the input given to them. Before the training begins, we usually assign random numbers as weights. The goal of the training process is essentially to gradually tune the values of each set of these weights until they, in conjunction with their corresponding nodes, produce satisfactory predictions.
To understand weights a little better, let’s examine the following dataset with two inputs and one output:
input1 | input2 | output |
---|---|---|
1 | 6 | 20 |
2 | 5 | 19 |
3 | 4 | 18 |
4 | 3 | 17 |
5 | 2 | 16 |
6 | 1 | 15 |
Using linear algebra (or guesswork in our minds), we can deduce that the equation governing this dataset is:
output = f(input1, input2) = 2 x input1 + 3 x input2
In this case, the weights for this mathematical operation are 2 and 3. A deep neural network has millions of such weight parameters.
Depending on the types of nodes used, different themes of model architectures will be better suited for different kinds of input data. For example, CNNs are used for image and audio, whereas Recurrent Neural Networks (RNNs) and LSTM are often used in text processing.
In general, training one of these models from scratch can take a pretty significant amount of time, potentially weeks. Luckily for us, many researchers have already done the difficult work of training them on a generic dataset (like ImageNet) and have made them available for everyone to use. What’s even better is that we can take these available models and tune them to our specific dataset. This process is called transfer learning and accounts for the vast majority of needs by practitioners.
Compared to training from scratch, transfer learning provides a two-fold advantage: significantly reduced training time a (few minutes to hours instead of weeks), and it can work with a substantially smaller dataset (hundreds to thousands of data samples instead of millions). Table 1-4 shows some famous examples of model architectures.
Task | Example model architectures |
---|---|
Image classification | ResNet-152 (2015), MobileNet (2017) |
Text classification | BERT (2018), XLNet (2019) |
Image segmentation | U-Net (2015), DeepLabV3 (2018) |
Image translation | Pix2Pix (2017) |
Object detection | YOLO9000 (2016), Mask R-CNN (2017) |
Speech generation | WaveNet (2016) |
Each one of the models from Table 1-4 has a published accuracy metric on reference datasets (e.g., ImageNet for classification, MS COCO for detection). Additionally, these architectures have their own characteristic resource requirements (model size in megabytes, computation requirements in floating-point operations, or FLOPS).
We explore transfer learning in-depth in the upcoming chapters. Now, let’s look at the kinds of deep learning frameworks and services that are available to us.
When Kaiming He et al. came up with the 152-layer ResNet architecture in 2015—a feat of its day considering the previous largest GoogLeNet model consisted of 22 layers—there was just one question on everyone’s mind: “Why not 153 layers?” The reason, as it turns out, was that Kaiming ran out of GPU memory!
Frameworks
There are several deep learning libraries out there that help us train our models. Additionally, there are frameworks that specialize in using those trained models to make predictions (or inference), optimizing for where the application resides.
Historically, as is the case with software generally, many libraries have come and gone—Torch (2002), Theano (2007), Caffe (2013), Microsoft Cognitive Toolkit (2015), Caffe2 (2017)—and the landscape has been evolving rapidly. Learnings from each have made the other libraries easier to pick up, driven interest, and improved productivity for beginners and experts alike. Table 1-5 looks at some of the popular ones.
Framework | Best suited for | Typical target platform |
---|---|---|
TensorFlow (including Keras) | Training | Desktops, servers |
PyTorch | Training | Desktops, servers |
MXNet | Training | Desktops, servers |
TensorFlow Serving | Inference | Servers |
TensorFlow Lite | Inference | Mobile and embedded devices |
TensorFlow.js | Inference | Browsers |
ml5.js | Inference | Browsers |
Core ML | Inference | Apple devices |
Xnor AI2GO | Inference | Embedded devices |
TensorFlow
In 2011, Google Brain developed the DNN library DistBelief for internal research and engineering. It helped train Inception (2014’s winning entry to the ImageNet Large Scale Visual Recognition Challenge) as well as helped improve the quality of speech recognition within Google products. Heavily tied into Google’s infrastructure, it was not easy to configure and to share code with external machine learning enthusiasts. Realizing the limitations, Google began working on a second-generation distributed machine learning framework, which promised to be general-purpose, scalable, highly performant, and portable to many hardware platforms. And the best part, it was open source. Google called it TensorFlow and announced its release on November 2015.
TensorFlow delivered on a lot of these aforementioned promises, developing an end-to-end ecosystem from development to deployment, and it gained a massive following in the process. With more than 100,000 stars on GitHub, it shows no signs of stopping. However, as adoption gained, users of the library rightly criticized it for not being easy enough to use. As the joke went, TensorFlow was a library by Google engineers, of Google engineers, for Google engineers, and if you were smart enough to use TensorFlow, you were smart enough to get hired there.
But Google was not alone here. Let’s be honest. Even as late as 2015, it was a given that working with deep learning libraries would inevitably be an unpleasant experience. Forget even working on these; installing some of these frameworks made people want to pull their hair out. (Caffe users out there—does this ring a bell?)
Keras
As an answer to the hardships faced by deep learning practitioners, François Chollet released the open source framework Keras in March 2015, and the world hasn’t been the same since. This solution suddenly made deep learning accessible to beginners. Keras provided an intuitive and easy-to-use interface for coding, which would then use other deep learning libraries as the backend computational framework. Starting with Theano as its first backend, Keras encouraged rapid prototyping and reduced the number of lines of code. Eventually, this abstraction expanded to other frameworks including Cognitive Toolkit, MXNet, PlaidML, and, yes, TensorFlow.
PyTorch
In parallel, PyTorch started at Facebook early in 2016, where engineers had the benefit of observing TensorFlow’s limitations. PyTorch supported native Python constructs and Python debugging right off the bat, making it flexible and easier to use, quickly becoming a favorite among AI researchers. It is the second-largest end-to-end deep learning system. Facebook additionally built Caffe2 to take PyTorch models and deploy them to production to serve more than a billion users. Whereas PyTorch drove research, Caffe2 was primarily used in production. In 2018, Caffe2 was absorbed into PyTorch to make a full framework.
A continuously evolving landscape
Had this story ended with the ease of Keras and PyTorch, this book would not have the word “TensorFlow” in the subtitle. The TensorFlow team recognized that if it truly wanted to broaden the tool’s reach and democratize AI, it needed to make the tool easier. So it was welcome news when Keras was officially included as part of TensorFlow, offering the best of both worlds. This allowed developers to use Keras for defining the model and training it, and core TensorFlow for its high-performance data pipeline, including distributed training and ecosystem to deploy. It was a match made in heaven! And to top it all, TensorFlow 2.0 (released in 2019) included support for native Python constructs and eager execution, as we saw in PyTorch.
With so many competing frameworks available, the question of portability inevitability arises. Imagine a new research paper published with the state-of-the-art model being made public in PyTorch. If we didn’t work in PyTorch, we would be locked out of the research and would have to reimplement and train it. Developers like to be able to share models freely and not be restricted to a specific ecosystem. Organically, many developers wrote libraries to convert model formats from one library to another. It was a simple solution, except that it led to a combinatorial explosion of conversion tools that lacked official support and sufficient quality due to the sheer number of them. To address this issue, the Open Neural Network Exchange (ONNX) was championed by Microsoft and Facebook, along with major players in the industry. ONNX provided a specification for a common model format that was readable and writable by a number of popular libraries officially. Additionally, it provided converters for libraries that did not natively support this format. This allowed developers to train in one framework and do inferences in a different framework.
Apart from these frameworks, there are several Graphical User Interface (GUI) systems that make code-free training possible. Using transfer learning, they generate trained models quickly in several formats useful for inference. With point-and-click interfaces, even your grandma can now train a neural network!
Service | Platform |
---|---|
Microsoft CustomVision.AI | Web-based |
Google AutoML | Web-based |
Clarifai | Web-based |
IBM Visual Recognition | Web-based |
Apple Create ML | macOS |
NVIDIA DIGITS | Desktop |
Runway ML | Desktop |
So why did we choose TensorFlow and Keras as the primary frameworks for this book? Considering the sheer amount of material available, including documentation, Stack Overflow answers, online courses, the vast community of contributors, platform and device support, industry adoption, and, yes, open jobs available (approximately three times as many TensorFlow-related roles compared to PyTorch in the United States), TensorFlow and Keras currently dominate the landscape when it comes to frameworks. It made sense for us to select this combination. That said, the techniques discussed in the book are generalizable to other libraries, as well. Picking up a new framework shouldn’t take you too long. So, if you really want to move to a company that uses PyTorch exclusively, don’t hesitate to apply there.
Hardware
In 1848, when James W. Marshall discovered gold in California, the news spread like wildfire across the United States. Hundreds of thousands of people stormed to the state to begin mining for riches. This was known as the California Gold Rush. Early movers were able to extract a decent chunk, but the latecomers were not nearly as lucky. But the rush did not stop for many years. Can you guess who made the most money throughout this period? The shovel makers!
Cloud and hardware companies are the shovel makers of the twenty-first century. Don’t believe us? Look at the stock performance of Microsoft and NVIDIA in the past decade. The only difference between 1849 and now is the mind-bogglingly large amount of shovel choices available to us.
Given the variety of hardware available, it is important to make the correct choices for the constraints imposed by resource, latency, budget, privacy, and legal requirements of the application.
Depending on how your application interacts with the user, the inference phase usually has a user waiting at the other end for a response. This imposes restrictions on the type of hardware that can be used as well as the location of the hardware. For example, a Snapchat lens cannot run on the cloud due to the network latency issues. Additionally, it needs to run in close to real time to provide a good user experience (UX), thus setting a minimum requirement on the number of frames processed per second (typically >15 fps). On the other hand, a photo uploaded to an image library such as Google Photos does not need immediate image categorization done on it. A few seconds or few minutes of latency is acceptable.
Going to the other extreme, training takes a lot more time; anywhere between minutes to hours to days. Depending on our training scenario, the real value of better hardware is enabling faster experimentation and more iterations. For anything more serious than basic neural networks, better hardware can make a mountain of difference. Typically, GPUs would speed things up by 10 to 15 times compared to CPUs, and at a much higher performance per watt, reducing the wait time for our experiment to finish from a week to a few hours. This can be the difference in watching a documentary about the Grand Canyon (two hours) versus actually making the trip to visit the Grand Canyon (four days).
Following are a few fundamental hardware categories to choose from and how they are typically characterized (see also Figure 1-10):
- Central Processing Unit (CPU)
-
Cheap, flexible, slow. For example, Intel Core i9-9900K.
- GPU
-
High throughput, great for batching to utilize parallel processing, expensive. For example, NVIDIA GeForce RTX 2080 Ti.
- Field-Programmable Gate Array (FPGA)
-
Fast, low power, reprogrammable for custom solutions, expensive. Known companies include Xilinx, Lattice Semiconductor, Altera (Intel). Because of the ability to run in seconds and configurability to any AI model, Microsoft Bing runs the majority of its AI on FPGAs.
- Application-Specific Integrated Circuit (ASIC)
-
Custom-made chip. Extremely expensive to design, but inexpensive when built for scale. Just like in the pharmaceutical industry, the first item costs the most due to the R&D effort that goes into designing and making it. Producing massive quantities is rather inexpensive. Specific examples include the following:
- Tensor Processing Unit (TPU)
-
ASIC specializing in operations for neural networks, available on Google Cloud only.
- Edge TPU
-
Smaller than a US penny, accelerates inference on the edge.
- Neural Processing Unit (NPU)
-
Often used by smartphone manufacturers, this is a dedicated chip for accelerating neural network inference.
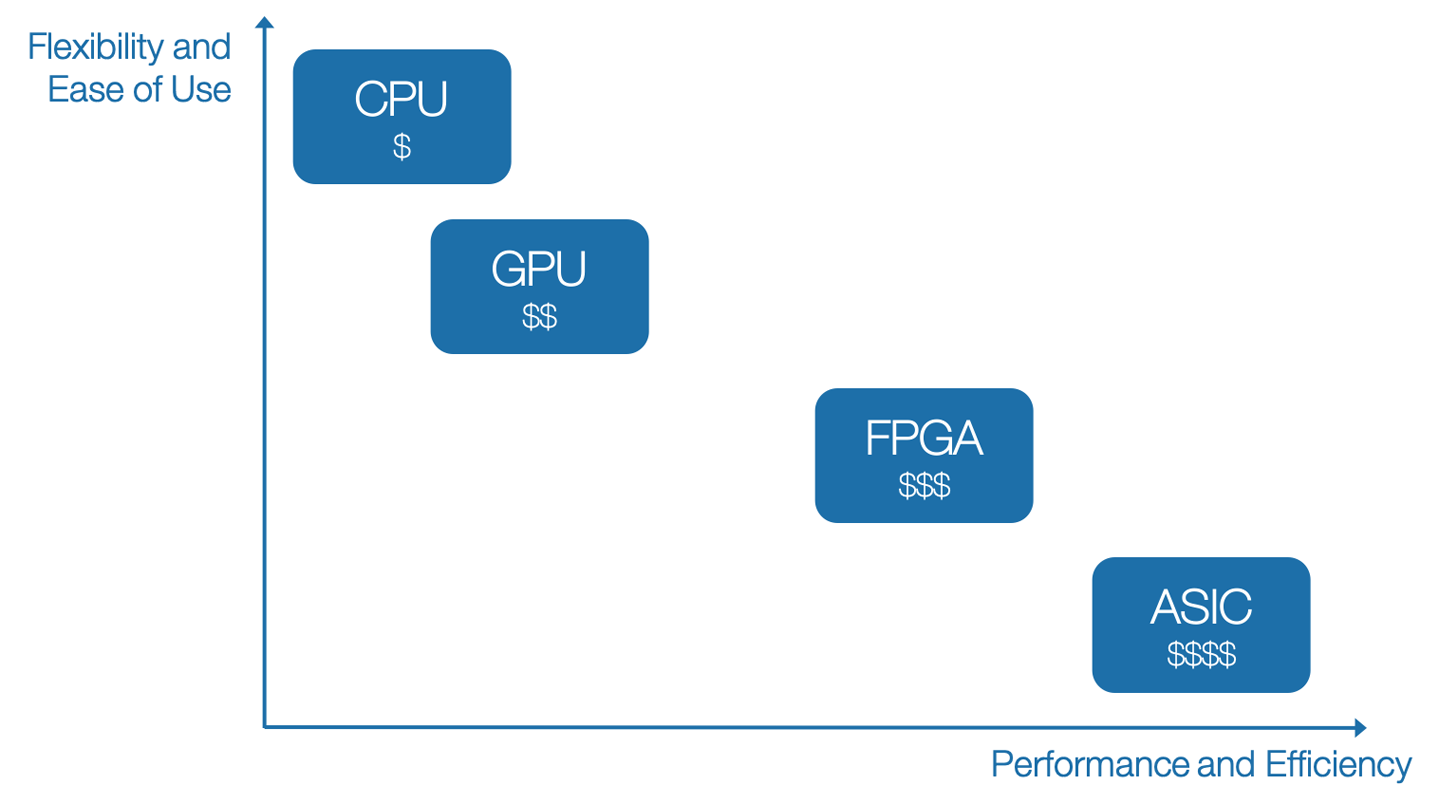
Figure 1-10. Comparison of different types of hardware relative to flexibility, performance, and cost
Let’s look at a few scenarios for which each one would be used:
-
Getting started with training → CPU
-
Training large networks → GPUs and TPUs
-
Inference on smartphones → Mobile CPU, GPU, Digital Signal Processor (DSP), NPU
-
Wearables (e.g., smart glasses, smartwatches) → Edge TPU, NPUs
-
Embedded AI projects (e.g., flood surveying drone, autonomous wheelchair) → Accelerators like Google Coral, Intel Movidius with Raspberry Pi, or GPUs like NVIDIA Jetson Nano, all the way down to $15 microcontrollers (MCUs) for wake word detection in smart speakers
As we go through the book, we will closely explore many of these.
Responsible AI
So far, we have explored the power and the potential of AI. It shows great promise to enhance our abilities, to make us more productive, to give us superpowers.
But with great power comes great responsibility.
As much as AI can help humanity, it also has equal potential to harm us when not designed with thought and care (either intentionally or unintentionally). The AI is not to blame; rather, it’s the AI’s designers.
Consider some real incidents that made the news in the past few years.
-
“____ can allegedly determine whether you’re a terrorist just by analyzing your face” (Figure 1-11): Computer World, 2016
-
“AI is sending people to jail—and getting it wrong”: MIT Tech Review, 2019
-
“____ supercomputer recommended ‘unsafe and incorrect’ cancer treatments, internal documents show”: STAT News, 2018
-
“____ built an AI tool to hire people but had to shut it down because it was discriminating against women”: Business Insider, 2018
-
“____ AI study: Major object recognition systems favor people with more money”: VentureBeat, 2019
-
“____ labeled black people ‘gorillas’” USA Today, 2015. “Two years later, ____ solves ‘racist algorithm’ problem by purging ‘gorilla’ label from image classifier”: Boing Boing, 2018
-
“____ silences its new A.I. bot Tay, after Twitter users teach it racism”: TechCrunch, 2016
-
“AI Mistakes Bus-Side Ad for Famous CEO, Charges Her With Jaywalking”: Caixin Global, 2018
-
“____ to drop Pentagon AI contract after employee objections to the ‘business of war’”: Washington Post, 2018
-
“Self-driving ____ death car ‘spotted pedestrian six seconds before mowing down and killing her’”: The Sun, 2018
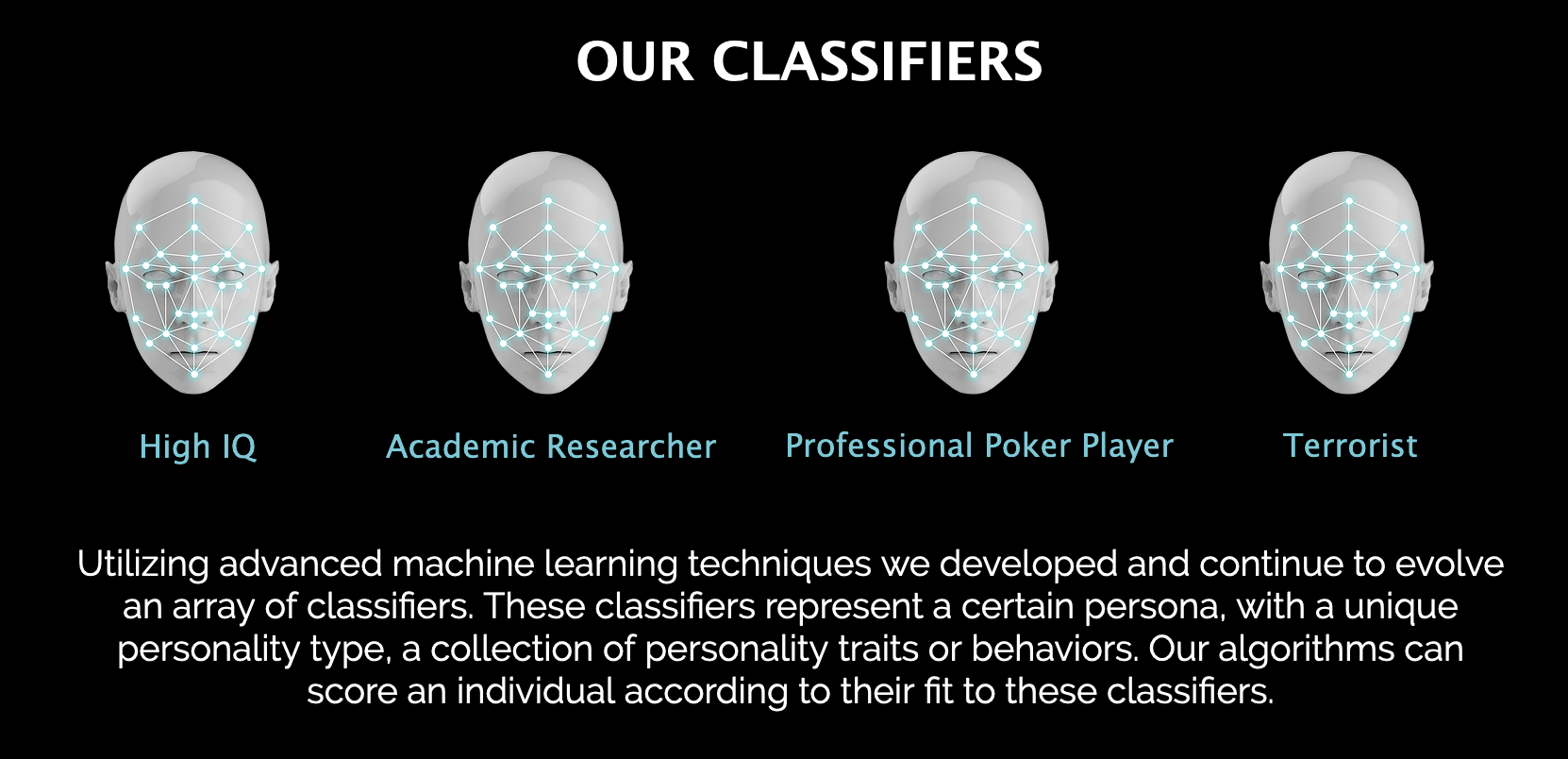
Figure 1-11. Startup claiming to classify people based on their facial structure
Can you fill in the blanks here? We’ll give you some options—Amazon, Microsoft, Google, IBM, and Uber. Go ahead and fill them out. We’ll wait.
There’s a reason we kept them blank. It’s to recognize that it’s not a problem belonging to a specific individual or a company. This is everyone’s problem. And although these things happened in the past, and might not reflect the current state, we can learn from them and try not to make the same mistakes. The silver lining here is that everyone learned from these mistakes.
We, as developers, designers, architects, and leaders of AI, have the responsibility to think beyond just the technical problem at face value. Following are just a handful of topics that are relevant to any problem we solve (AI or otherwise). They must not take a backseat.
Bias
Often in our everyday work, we bring in our own biases, knowingly or unknowingly. This is the result of a multitude of factors including our environment, upbringing, cultural norms, and even our inherent nature. After all, AI and the datasets that power them were not created in a vacuum—they were created by human beings with their own biases. Computers don’t magically create bias on their own, they reflect and amplify existing ones.
Take the example from the early days of the YouTube app when the developers noticed that roughly 10% of uploaded videos were upside-down. Maybe if that number had been lower, say 1%, it could have been brushed off as user error. But 10% was too high a number to be ignored. Do you know who happens to make up 10% of the population? Left-handed people! These users were holding their phones in the opposite orientation as their right-handed peers. But the engineers at YouTube had not accounted for that case during the development and testing of their mobile app, so YouTube uploaded videos to its server in the same orientation for both left-handed and right-handed users.
This problem could have been caught much earlier if the developers had even a single left-handed person on the team. This simple example demonstrates the importance of diversity. Handedness is just one small attribute that defines an individual. Numerous other factors, often outside their control, often come into play. Factors such as gender, skin tone, economic status, disability, country of origin, speech patterns, or even something as trivial as hair length can determine life-changing outcomes for someone, including how an algorithm treats them.
Google’s machine learning glossary lists several forms of bias that can affect a machine learning pipeline. The following are just some of them:
- Selection bias
-
The dataset is not representative of the distribution of the real-world problem and is skewed toward a subset of categories. For example, in many virtual assistants and smart home speakers, some spoken accents are overrepresented, whereas other accents have no data at all in the training dataset, resulting in a poor UX for large chunks of the world’s population.
Selection bias can also happen because of co-occurrence of concepts. For example, Google Translate, when used to translate the sentences “She is a doctor. He is a nurse” into a gender-neutral language such as Turkish and then back, switches the genders, as demonstrated in Figure 1-12. This is likely because the dataset contains a large sample of co-occurrences of male pronouns and the word “doctor,” and female pronouns and the word “nurse.”
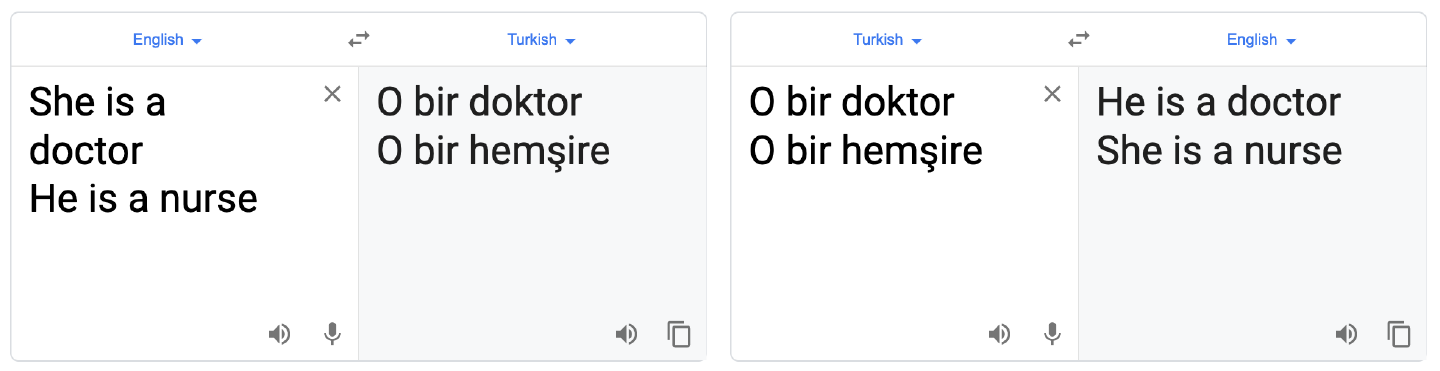
Figure 1-12. Google Translate reflecting the underlying bias in data (as of September 2019)
- Implicit bias
-
This type of bias creeps in because of implicit assumptions that we all make when we see something. Consider the highlighted portion in Figure 1-13. Anyone shown it might assume with a high amount of certainty that those stripes belong to a zebra. In fact, given how much ImageNet-trained networks are biased toward textures,2 most of them will classify the full image as a zebra. Except that we know that the image is of a sofa upholstered in a zebra-like fabric.
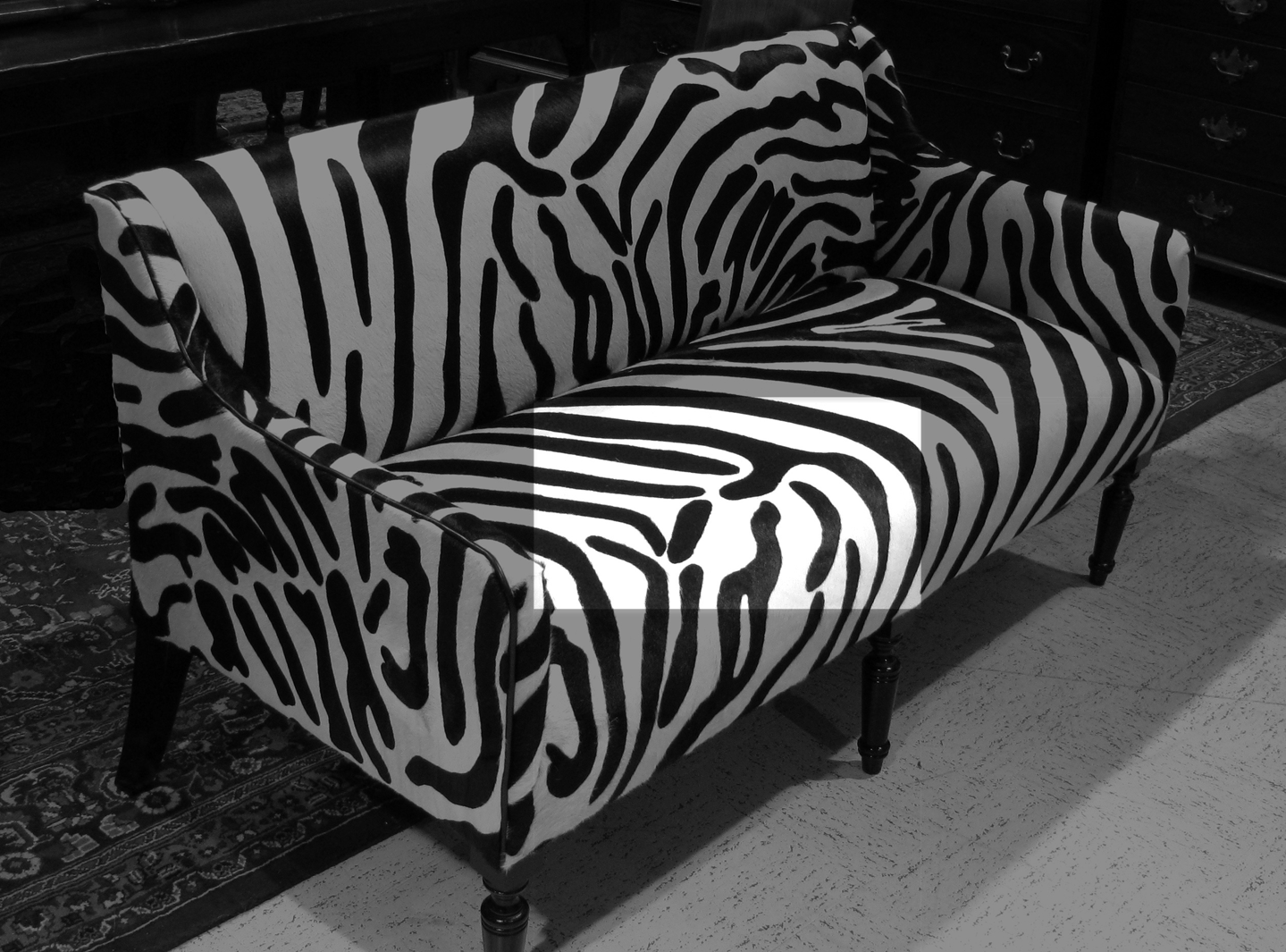
Figure 1-13. Zebra sofa by Glen Edelson (image source)
- Reporting bias
-
Sometimes the loudest voices in the room are the most extreme ones and dominate the conversation. One good look at Twitter might make it seem as if the world is ending, whereas most people are busy leading mundane lives. Unfortunately, boring does not sell.
- In-group/out-group bias
-
An annotator from East Asia might look at a picture of the Statue of Liberty and give it tags like “America” or “United States,” whereas someone from the US might look at the same picture and assign more granular tags such as “New York” or “Liberty Island.” It’s human nature to see one’s own groups with nuance while seeing other groups as more homogenous, and that reflects in our datasets, as well.
Accountability and Explainability
Imagine, in the late 1800s, Mr. Karl Benz told you that he invented this four-wheeled device that could transport you quicker than anything else in existence. Except he had no idea how it worked. All he knew was that it consumed a highly flammable liquid that exploded several times inside it to propel it forward. What caused it to move? What caused it to stop? What stopped it from burning the person sitting inside it? He had no answers. If this was the origin story of the car, you’d probably not want to get into that contraption.
This is precisely what is happening with AI right now. Previously, with traditional machine learning, data scientists had to manually pick features (predictive variables) from data, from which a machine learning model then would learn. This manual selection process, although cumbersome and restrictive, gave them more control and insight into how the prediction came about. However, with deep learning, these features are automatically selected. Data scientists are able to build models by providing lots of data, and these models somehow end up making predictions reliably—most of the time. But the data scientist doesn’t know exactly how the model works, what features it learned, under what circumstances the model works, and, more importantly, the circumstances under which it doesn’t work. This approach might be acceptable when Netflix is recommending TV shows to us based on what we’ve already watched (although we’re fairly certain they have the line recommendations.append("Stranger Things")
in their code somewhere). But AI does a lot more than just recommend movies these days. Police and judicial systems are beginning to rely on algorithms to decide whether someone poses a risk to society and whether they should be detained before their trial. The lives and freedoms of many people are at stake. We simply must not outsource important decision making to an unaccountable black box. Thankfully, there’s momentum to change that with investments in Explainable AI, wherein the model would be able to not just provide predictions but also account for the factors that caused it to make a certain prediction, and reveal areas of limitations.
Additionally, cities (such as New York) are beginning to make their algorithms accountable to the public by recognizing that the public has a right to know what algorithms they use for vital decision making and how they work, allowing reviews and audits by experts, improving expertise in government agencies to better evaluate each system they add, and by providing mechanisms to dispute a decision made by an algorithm.
Reproducibility
Research performed in the scientific field gains wide acceptance by the community only when it’s reproducible; that is, anyone studying the research should be able to replicate the conditions of the test and obtain the same results. Unless we can reproduce a model’s past results, we cannot hold it accountable when using it in the future. In the absence of reproducibility, research is vulnerable to p-hacking—tweaking the parameters of an experiment until the desired results are obtained. It’s vital for researchers to extensively document their experimental conditions, including the dataset(s), benchmarks, and algorithms, and declare the hypothesis they will be testing prior to performing an experiment. Trust in institutions is at an all-time low and research that is not grounded in reality, yet sensationalized by the media, can erode that trust even more. Traditionally, replicating a research paper was considered a dark art because many implementation details are left out. The uplifting news is that researchers are now gradually beginning to use publicly available benchmarks (as opposed to their privately constructed datasets) and open sourcing the code they used for their research. Members of the community can piggyback on this code, prove it works, and make it better, thereby leading to newer innovations rapidly.
Robustness
There’s an entire area of research on one-pixel attacks on CNNs. Essentially, the objective is to find and modify a single pixel in an image to make a CNN predict something entirely different. For example, changing a single pixel in a picture of an apple might result in a CNN classifying it as a dog. A lot of other factors can influence predictions, such as noise, lighting conditions, camera angle, and more that would not have affected a human’s ability to make a similar call. This is particularly relevant for self-driving cars, where it would be possible for a bad actor on a street to modify the input the car sees in order to manipulate it into doing bad things. In fact, Tencent’s Keen Security Lab was able to exploit a vulnerability in Tesla’s AutoPilot by strategically placing small stickers on the road, which led it to change lanes and drive into the oncoming lane. Robust AI that is capable of withstanding noise, slight deviations, and intentional manipulation is necessary if we are to be able to trust it.
Privacy
In the pursuit of building better and better AI, businesses need to collect lots of data. Unfortunately, sometimes they overstep their bounds and collect information overzealously beyond what is necessary for the task at hand. A business might believe that it is using the data it collects only for good. But what if it is acquired by a company that does not have the same ethical boundaries for data use? The consumer’s information could be used for purposes beyond the originally intended goals. Additionally, all that data collected in one place makes it an attractive target for hackers, who steal personal information and sell it on the black market to criminal enterprises. Moreover, governments are already overreaching in an attempt to track each and every individual.
All of this is at odds to the universally recognized human right of privacy. What consumers desire is having transparency into what data is being collected about them, who has access to it, how it’s being used, and mechanisms to opt out of the data collection process, as well to delete data that was already collected on them.
As developers, we want to be aware of all the data we are collecting, and ask ourselves whether a piece of data is even necessary to be collected in the first place. To minimize the data we collect, we could implement privacy-aware machine learning techniques such as Federated Learning (used in Google Keyboard) that allow us to train networks on the users’ devices without having to send any of the Personally Identifiable Information (PII) to a server.
It turns out that in many of the aforementioned headlines at the beginning of this section, it was the bad PR fallout that brought mainstream awareness of these topics, introduced accountability, and caused an industry-wide shift in mindset to prevent repeats in the future. We must continue to hold ourselves, academics, industry leaders, and politicians accountable at every misstep and act swiftly to fix the wrongs. Every decision we make and every action we take has the potential to set a precedent for decades to come. As AI becomes ubiquitous, we need to come together to ask the tough questions and find answers for them if we want to minimize the potential harm while reaping the maximum benefits.
Summary
This chapter explored the landscape of the exciting world of AI and deep learning. We traced the timeline of AI from its humble origins, periods of great promise, through the dark AI winters, and up to its present-day resurgence. Along the way, we answered the question of why it’s different this time. We then looked at the necessary ingredients to build a deep learning solution, including datasets, model architectures, frameworks, and hardware. This sets us up for further exploration in the upcoming chapters. We hope you enjoy the rest of the book. It’s time to dig in!
Frequently Asked Questions
-
I’m just getting started. Do I need to spend a lot of money on buying powerful hardware?
Luckily for you, you can get started even with your web browser. All of our scripts are available online, and can be run on free GPUs courtesy of the kind people at Google Colab (Figure 1-14), who are generously making powerful GPUs available for free (up to 12 hours at a time). This should get you started. As you become better at it by performing more experiments (especially in a professional capacity or on large datasets), you might want to get a GPU either by renting one on the cloud (Microsoft Azure, Amazon Web Services (AWS), Google Cloud Platform (GCP), and others) or purchasing the hardware. Watch out for those electricity bills, though!
Figure 1-14. Screenshot of a notebook on GitHub running on Colab inside Chrome
-
Colab is great, but I already have a powerful computer that I purchased for playing <insert name of video game>. How should I set up my environment?
The ideal setup involves Linux, but Windows and macOS work, too. For most chapters, you need the following:
-
Python 3 and PIP
-
tensorflow
ortensorflow-gpu
PIP package (version 2 or greater) -
Pillow
We like keeping things clean and self-contained, so we recommend using Python virtual environments. You should use the virtual environment whenever you install a package or run a script or a notebook.
If you do not have a GPU, you are done with the setup.
If you have an NVIDIA GPU, you would want to install the appropriate drivers, then CUDA, then cuDNN, then
tensorflow-gpu
package. If you’re using Ubuntu, there’s an easier solution than installing these packages manually, which can be tedious and error prone even for the best of us: simply install the entire environment with just one line using Lambda Stack.Alternatively, you could install all of your packages using Anaconda Distribution, which works equally well for Windows, Mac, and Linux.
-
-
Where will I find the code used in this book?
You’ll find ready-to-run examples at http://PracticalDeepLearning.ai.
-
What are the minimal prerequisites to be able to read this book?
A Ph.D. in areas including Calculus, Statistical Analysis, Variational Autoencoders, Operations Research, and so on...are definitely not necessary to be able to read this book (had you a little nervous, didn’t we?). Some basic coding skills, familiarity with Python, a healthy amount of curiosity, and a sense of humor should go a long way in the process of absorbing the material. Although a beginner-level understanding of mobile development (with Swift and/or Kotlin) will help, we’ve designed the examples to be self-sufficient and easy enough to be deployed by someone who has never written a mobile app previously.
-
What frameworks will we be using?
Keras + TensorFlow for training. And chapter by chapter, we explore different inference frameworks.
-
Will I be an expert when I finish this book?
If you follow along, you’ll have the know-how on a wide variety of topics all the way from training to inference, to maximizing performance. Even though this book primarily focuses on computer vision, you can bring the same know-how to other areas such as text, audio, and so on and get up to speed very quickly.
-
Who is the cat from earlier in the chapter?
That is Meher’s cat, Vader. He will be making multiple cameos throughout this book. And don’t worry, he has already signed a model release form.
-
Can I contact you?
Sure. Drop us an email at PracticalDLBook@gmail.com with any questions, corrections, or whatever, or tweet to us @PracticalDLBook.
1 If you’re reading a pirated copy, consider us disappointed in you.
Get Practical Deep Learning for Cloud, Mobile, and Edge now with the O’Reilly learning platform.
O’Reilly members experience books, live events, courses curated by job role, and more from O’Reilly and nearly 200 top publishers.