Chapter 4. Data Engineering
Introduction
In earlier chapters, you were introduced to abstract concepts. Now, we’ll move forward from that technical introduction to discuss implementation details and more subjective choices. I’ll show you how we work with the art of training data in practice as we walk through scaling to larger projects and optimizing performance.
Data ingestion is the first and one of the most important steps. And the first step to ingestion is setting up and using a training data system of record (SoR). An example of an SoR is a training data database.
Why is data ingestion hard? Many reasons. For example, training data is a relatively new concept, there are a variety of formatting and communication challenges. The volume, variety, and velocity of data vary, and there is a lack of well-established norms, leading to many ways to do it.
Also, there are many concepts, like using a training data database, and who wants to access what when; that may not be obvious, even to experienced engineers. Ingestion decisions ultimately determine query, access, and export considerations.
This chapter is organized into:
Who wants to use the data and when they want to use it
Why the data formats and communication methods matter; think “game of telephone”
An introduction to a training data database as your system of record
The technical basics of getting started
Storage, media-specific needs, and versioning
Commercial concerns of formatting and mapping data
Data access, security, and pre-labeled data
To achieve a data-driven or data-centric approach, tooling, iteration, and data are needed. The more iteration and the more data, the more need there is for great organization to handle it.
You may ingest data, explore it, and annotate it in that order. Or perhaps you may go straight from ingesting to debugging a model. After streaming to training, you may ingest new predictions, then debug those, then use annotation workflow. The more you can lean on your database to do the heavy lifting, the less you have to do yourself.
Who Wants the Data?
Before we dive into the challenges and the technical specifics, let’s set the table about goals and the humans involved here and discuss how data engineering services those end users and systems. After, I’ll cover the conceptual reasons for wanting a training data database. I’ll frame the need for this by showing what the default case looks like without it, and then what it looks like with it.
For ease of discussion, we can divide this into groups:
Annotators
Data scientists
ML programs (machine to machine)
Application engineers
Other stakeholders
Annotators
Annotators need to be served the right data at the right time with the right permissions. Often, this is done at a single-file level, and is driven by very specifically scoped requests. There is an emphasis on permissions and authorization. In addition, the data needs to be delivered at the proper time—but what is the “right time”? Well, in general it means on-demand or online access. This is where the file is identified by a software process, such as a task system, and served with fast response times.
Data scientists
Data science most often looks at data at the set level. More emphasis is placed on query capabilities, the ability to handle large volumes of data, and formatting. Ideally, there is also the ability to drill down into specific samples and compare the results of different approaches both quantitatively and qualitatively.
ML programs
ML programs follow a path similar to that of data science. Differences include permissions schemes (usually programs have more access than individual data scientists), and clarity on what gets surfaced and when (usually more integration and process oriented versus on-demand analysis). Often, ML programs can have a software-defined integration or automation.
Application engineers
Application engineers are concerned with getting data from the application to the training data database and how to embed the annotation and supervision to end users. Queries per second (throughput) and volume of data are often top concerns. There is sometimes a faulty assumption that there is a linear flow of data from an “ingestion” team, or the application, to the data scientists.
Other stakeholders
Other stakeholders with an interest in the training data might be security personnel, DevMLOps professionals, backup systems engineers, etc. These groups often have cross-domain concerns and crosscut other users’ and systems’ needs. For example, security cares about end-user permissions already mentioned. Security also cares about a single data scientist not being a single point of critical failure, e.g., having the entire dataset on their machine or overly broad access to remote sets.
Now that you have an overview of the groups involved, how do they talk with each other? How do they work together?
A Game of Telephone
Telephone is “a game where you come up with a phrase and then you whisper it into the ear of the person sitting next to you. Next, this person has to whisper what he or she heard in the next person’s ear. This continues in the circle until the last person has heard the phrase. Errors typically accumulate in the retellings, so the statement announced by the last player differs significantly from that of the first player, usually with amusing or humorous effect.”1
As an analogy, you can think of suboptimal data engineering as a game of telephone as shown in Figure 4-1. At each stage, accumulated data errors increase. And it’s actually even worse than that graphic shows. This is because sensors, humans, models, data, and ML systems all interact with each other in nonlinear ways.
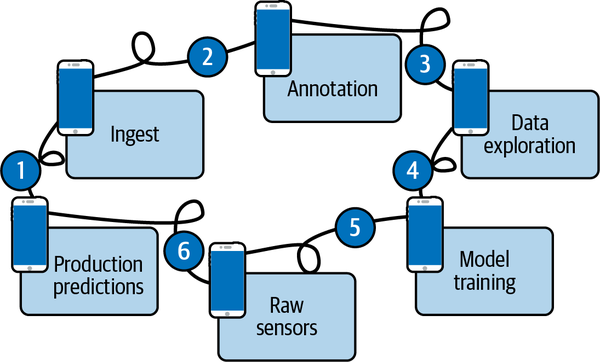
Figure 4-1. Without a system of record, data errors accumulate like a game of telephone
Unlike the game, with training data, these errors are not humorous. Data errors cause poor performance, system degradation, and failures, resulting in real-world physical and financial harm. At each stage, things like different formats, poor or missing data definitions, and assumptions will cause the data to get malformed and garbled. While one tool may know about “xyz” properties, the next tool might not. And it repeats. The next tool won’t export all of the properties, or will modify them randomly, etc. No matter how trivial these issues seem on the whiteboard, they will always be a problem in the real world.
Problems are especially prevalent in larger, multiteam contexts and when the holistic needs of all major groups are not taken into account. As a new area with emerging standards, even seemingly simple things are poorly defined. In other words, data engineering is especially important for training data. If you have a greenfield (new) project, then now is the perfect time to plan your data engineering.
How do you know when a system of record is needed? Let’s explore that next.
When a system of record is needed
From planning a new project to rethinking existing projects, signals it’s time for a training data system of record include the following:
There is data loss between teams, e.g., because each team owns their own copy of the data.
Teams aggregate other teams’ data but only use a small slice of it, e.g., when a query would be better.
There is abuse or overuse of unstructured or semi-structured formats like CSVs, strings, JSON, etc., e.g., dumping output into many CSV files in a bucket.
Cases emerge where the format is only known to the unique application that generated the data. For example, people might be loading unstructured or badly structured data after the fact, hoping for the best.
There are over assumptions that each system will run in a specific, predefined daisy-chained order instead of a more composable design.
The overall system performance is not meeting expectations or models are slow to ship or update.
Alternatively, you may simply have no true system of record anywhere. If you have a system, but it does not holistically represent the state of the training data (e.g., thinking of it as just labeling rather than as the center of gravity of the process) then its usefulness is likely low. This is because there will likely be an unreasonable level of communication required to make changes (e.g., changing the schema is not one quick API call or one UI interaction). It also means changes that should be done by end users must be discussed as an engineering-level change.
If each team owns its own copy of the data, there will be unnecessary communications and integration overhead, and likely data loss. This copying is often the “original sin,” since the moment there are multiple teams doing this, it will take an engineering-level change to update the overall system—meaning updates will not be fluid, leading to the overall system performance not meeting expectations.
The user expectations and data formats change frequently enough that the solution can’t be an overly rigid, automatic process. So don’t think about this in terms of automation, but rather in terms of “where is the center of gravity of training data?” It should be with the humans and a system of record, e.g., a training data database, in order to get the best results.
Planning a Great System
So how do you avoid the game of telephone? It starts with planning. Here are a couple of thought starters, and then I’ll walk through best practices for the actual setup.
The first is to establish a meaningful unit of work relevant to your business. For example, for a company doing analytics on medical videos, it could be a single medical procedure. Then, within each procedure, think about how many models are needed (don’t assume one!), how often they will be updated, how the data will flow, etc. We will cover this in more depth in “Overall system design”, but for now I just want to make sure it’s clear that ingestion is often not a “once and done” thing. It’s something that will need ongoing maintenance, and likely expansion over time.
Second, is to think about the data storage and access, setting up a system of record like a training data database. While it is possible to “roll your own,” it’s difficult to holistically consider the needs of all of the groups. The more a training data database is used, the easier it is to manage the complexity. The more independent storage is used, the more pressure is put on your team to “reinvent the wheel” of the database.
There are some specifics to building a great ingestion subsystem. Usually, the ideal is that these sensors feed directly into a training data system. Think about how much distance, or hops, are there between sensors, predictions, raw data, and your training data tools.
Production data often needs to be reviewed by humans, analyzed at a set level, and potentially further “mined” for improvements. The more predictions, the more opportunity for further system correction. You’ll need to consider questions like: How will production data get to the training data system in a useful way? How many times is data duplicated during the tooling processes?
What are our assumptions around the distinctions between various uses of the data? For example, querying the data within a training data tool scales better than expecting a data scientist to export all the data and then query it themselves after.
Naive and Training Data–Centric Approaches
There are two main approaches that people tend to take to working with training data. One I’ll refer to as “naive,” and the other is more centered on the importance of the data itself (data-centric).
Naive approaches tend to see training data as just one step to be bolted alongside a series of existing steps. Data-centric approaches see the human actions of supervising the data as the “center of gravity” of the system. Many of the approaches in this book align well or equate directly to being data-centric, in some ways making the training-data-first mindset synonymous with data-centric.
For example, a training data database has the definitions, and/or literal storage, of the raw data, annotations, schema, and mapping for machine-to-machine access.
There is naturally some overlap in approaches. In general, the greater the competency of the naive approach, the more it starts to look like a re-creation of a training data–centric one. While it’s possible to achieve desirable results using other approaches, it is much easier to consistently achieve desirable results with a training data–centric approach.
Let’s get started by looking at how naive approaches typically work.
Naive approaches
Typically, in a naive approach, sensors capture, store, and query the data independently of the training data tooling, as shown in Figure 4-2. This usually ends up looking like a linear process, with pre-established start and end conditions.
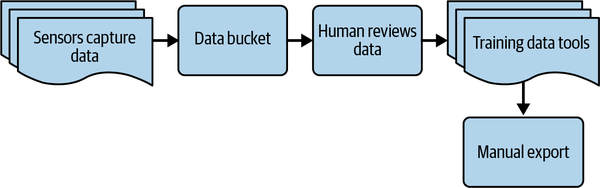
Figure 4-2. Naive data engineering process example
The most common reasons naive approaches get used:
The project started before training data–centric approaches had mature tooling support.
Engineers did not know about training data–centric approaches.
Testing and development of new systems.
Old, historical data, with no chance of new data coming in (rare).
Cases where it’s impractical to use a training data–centric approach (rare).
Naive approaches tend to look like the game of telephone mentioned earlier. Since each team has its own copy of the data, errors accumulate as that data gets passed around. Since there is either no system of record, or the system of record does not contain the complete state of training data, it is difficult to make changes at the user level. Overall, the harder it is to safely make changes, the slower it is to ship and iterate, and the worse the overall results are.
Additionally, naive approaches tend to be coupled to hidden or undefined human processes. For example, some engineer somewhere has a script on their local machine that does some critical part of the overall flow, but that script is not documented or not accessible to others in a reasonable way. This often occurs because of a lack of understanding of how to use a training data database, as opposed to being a purposeful action.
In naive approaches, there is a greater chance of data being unnecessarily duplicated. This increases hardware costs, such as storage and network bandwidth, in addition to the already-mentioned conceptual bottlenecks between teams. It also can introduce security issues since the various copies may maintain different security postures. For example, a team aggregating or correlating data might bypass security in a system earlier in the processing chain.
A major assumption in naive approaches is that a human administrator is manually reviewing the data (usually only at the set level), so that only the data that appears to be desired to be annotated is imported. In other words, only data pre-designated (usually through a relatively inconsistent means) for annotation is imported. This “random admin before import” assumption makes it hard to effectively supervise production data and use exploration methods, and generally bottlenecks the process because of the manual and undefined nature of the hidden curation process. Essentially this is relying on implicit admin-driven assumptions instead of explicitly defining processes with multiple stakeholders including SMEs. To be clear, it’s not the reviewing of the data that’s at issue—it’s the distinction between a process driven at a larger team level being better than a single admin person working in a more arbitrary manner.
Please think of this conceptually, not in terms of literal automation. A software-defined ingestion process is, by itself, little indication of a system’s overall health, since it does not speak to any of the real architectural concerns around the usage of a training data database.
Training data–centric (system of record)
Another option is to use a training data–centric approach. A training data database, as shown in Figure 4-3, is the heart of a training data–centric approach. It forms the system of record for your applications.
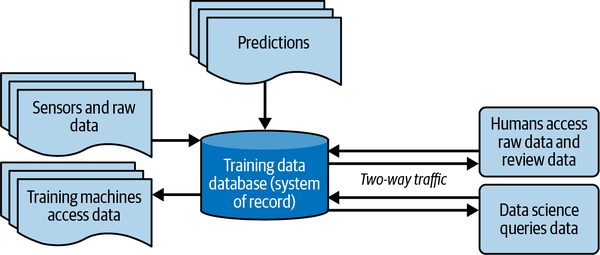
Figure 4-3. Training data database (system of record)
A training data database has the definitions, and/or literal storage, of the raw data, annotations, schema, and mapping for machine-to-machine access, and more. Ideally, it’s the complete definition of the system, meaning that given the training data database, you could reproduce the entire ML process with no additional work.
When you use a training data database as your system of record, you now have a central place for all teams to store and access training data. The greater the usage of the database, the better the overall results—similar to how the proper use of a database in a traditional application is well-known to be essential.
The most common reasons to use a training data database:
It supports a shift to data-centric machine learning. This means focusing on improving overall performance by improving the data instead of just improving the model algorithm.
It supports more easily aligning multiple ML programs by having the training data definitions all in one place.
It supports end users supervising (annotating) data and supports embedding of end-user supervision deeper in workflows and applications.
Data access is now query-based instead of requiring bulk duplication and extra aggregation steps.
Continuing the theme of the distinction between training data and data science, at the integration points, naturally, the line will be the most blurred. In practice, it is reasonable to invoke ML programs from a training data system.
Other reasons for using a training data database include the following:
It decouples visual UI requirements from data modeling (e.g., query mechanisms).
It enables faster access to new tools for data discovery, what to label, and more.
It enables user-defined file types, e.g., representing an “Interaction” as a set of images and text, which supports fluid iteration and end user–driven changes.
It avoids data duplication and stores external mapping definitions and relationships in one place.
It unblocks teams to work as fast as they can instead of waiting for discrete stages to be completed.
There are a few problems with a training data database approach:
Using one requires knowledge of its existence and conceptual understanding.
Staff need to have the time, ability, and resources to use a training data database.
Well-established data access patterns may require reworking to the new context.
Its reliability as a system of record does not have the same history as older systems have.
Ideally, instead of deciding what raw data to send (e.g., from an application to the database), all relevant data gets sent to the database first, before humans select samples to label. This helps to ensure it is the true system of record. For example, there may be a “what to label” program that uses all of the data, even if the humans only review a sample of it. Having all the data available to the “what to label” program through the training data database makes this easy. The easiest way to remember this is to think of the training data database as the center of gravity of the process.
The training data database takes on the role of managing references to, or even contains the literal byte storage of, raw media. This means sending the data directly to the training data tool. In actual implementation, there could be a series of processing steps, but the idea is that the final resting place of the data, the source of truth, is inside the training data database.
A training data database is the complete definition of the system, meaning that given the training data database, you can create, manage, and reproduce all end-user ML application needs, including ML processes, with no additional work. This goes beyond MLOps, which is an approach that often focuses more on automation, modeling, and reproducibility, purely on the ML side. You can think of MLOps as a sub-concern of a more strategic training data–centric approach.
A training data database considers multiple users from day one and plans accordingly. For example, an application designed to support data exploration can establish that indexes for data discovery are automatically created at ingest. When it saves annotations, it can also create indexes for discovery, streaming, security, etc., all at the same time.
A closely related theme is that of exporting the data to other tools. Perhaps you want to run some process to explore the data, and then need to send it to another tool for a security process, such as to blur personally identifiable info. Then you need to send it on to some other firm to annotate, then get the results back from them and into your models, etc. And let’s say that at each of these steps, there is a mapping (definitions) problem. Tool A outputs in a different format than Tool B inputs. This type of data transfer often requires orders of magnitude more computing resources than when using other common systems. In a sense, each transfer is more like a mini-database migration, because all the components of the data have to go through the conversion process. This is discussed in “Scale” as well.
Generally speaking, the tighter the connection between the sensors and the training data tools, the more potential for all of the end users and tools to be effective together. Every other step that’s added between sensors and the tools is virtually guaranteed to be a bottleneck. The data can still be backed up to some other service at the same time, but generally, this means organizing the data in the training data tooling from day one.
The first steps
Let’s say you are on board to use a training data–centric approach. How do you actually get started?
The first steps are to:
Set up training data database definitions
Set up data ingestion
Let’s consider definitions first. A training data database puts all the data in one place, including mapping definitions to other systems. This means that there is one single place for the system of record and the associated mapping definitions to modules running within the training data system and external to it. This reduces mapping errors, data transfer needs, and data duplication.
Before we start actually ingesting data, here are a few more terms that need to be covered first:
Raw data storage
Raw media BLOB-specific concerns
Formatting and mapping
Data access
Security concerns
Let’s start with raw data storage.
Raw Data Storage
The objective is to get the raw data, such as images, video, and text, into a usable form for training data work. Depending on the type of media, this may be easier or harder. With small amounts of text data, the task is relatively easy; with large amounts of video or even more specialized data like genomics, it becomes a central challenge.
It is common to store raw data in a bucket abstraction. This can be on the cloud or using software like MinIO. Some people like to think of these cloud buckets as “dump it and forget it,” but there are actually a lot of performance tuning options available. At the training data scale, raw storage choices matter. There are a few important considerations to keep in mind when identifying your storage solution:
- Storage class
- There are more major differences between storage tiers than it may first appear. The tradeoffs involve things like access time, redundancy, geo-availability, etc. There are orders-of-magnitude price differences between the tiers. The most key tool to be aware of is lifecycle rules, e.g., Amazon S3’s, wherein, usually with a few clicks, you can set policies to automatically move old files to cheaper storage options as they age. Examples of best practices in more granular detail can be found on Diffgram’s site.
- Geolocation (aka Zone, Region)
- Are you storing data on one side of the Atlantic Ocean and having annotators access it on the other? It’s worth considering where the actual annotation is expected to happen, and if there are options to store the data closer to it.
- Vendor support
- Not all annotation tools have the same degree of support for all major vendors. Keep in mind that you can typically manually integrate any of these offerings, but this requires more effort than tools that have native integration.
Support for accessing data from these storage providers is different from the tool running on that provider. Some tools may support access from all three, but as a service, the tool itself runs on a single cloud. If you have a system you install on your own cloud, usually the tool will support all three.
For example, you may choose to install the system on Azure. You may then pull data into the tool from Azure, which leads to better performance. However, that doesn’t prevent you from pulling data from Amazon and Google as needed.
By Reference or by Value
Storing by reference means storing only small pieces of information, such as strings or IDs, that allow you to identify and access the raw bytes on an existing source system. By value means copying the literal bytes. Once they are copied to the destination system, there is no dependency on the source system.
If you want to maintain your folder structure, some tools support referencing the files instead of actually transferring them. The benefit of this is less data transfer. A downside is that now it’s possible to have broken links. Also, separation of concerns could be an issue; for example, some other process may modify a file that the annotation tool expects to be able to access.
Even when you use a pass-by-reference approach for the raw data, it’s crucial that the system of truth is the training data database. For example, data may be organized into sets in the database that are not represented in the bucket organization. In addition, the bucket represents only the raw data, whereas the database will have the annotations and other associated definitions.
For simplicity, it’s best to think of the training data database as one abstraction, even if the raw data is stored outside the physical hardware of the database, as shown in Figure 4-4.
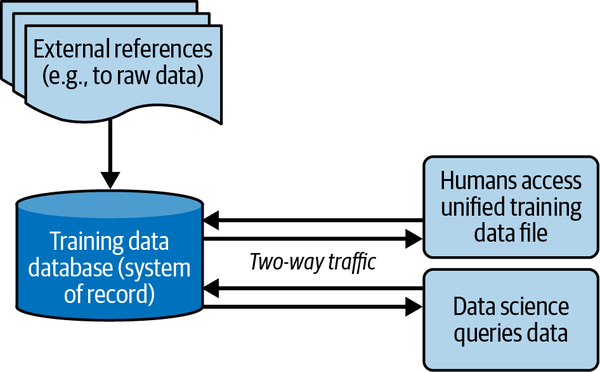
Figure 4-4. Training data database with references to raw data stored externally
Off-the-Shelf Dedicated Training Data Tooling on Your Own Hardware
Let’s assume that your tooling is trustworthy (perhaps you were able to inspect the source code). In this context, we trust the training data tool to manage the signed URL process, and to handle the identity and access management (IAM) concerns. With this established, the only real concern is what bucket it uses—and that generally becomes a one-time concern, because the tool manages the IAM. For advanced cases, the tool can still link up with a single sign-on (SSO) or more complex IAM scheme.
Note: The tooling doesn’t have to run on the hardware. The next level up is to trust the training data tooling, and also trust the service provider to host/process the data. Although this is an option, keep in mind that it provides the least level of control.
Data Storage: Where Does the Data Rest?
Generally speaking, any form of tooling will generate some form of data that is added to the “original” data. The BLOB data is usually stored by reference, or by physically moving the data.
This means if I have data in bucket A, and I use a tool to process it, there has to either be additional data in bucket A, or I will need a new bucket, B, to be used by the tool. This is true for Diffgram, SageMaker, and, as far as I’m aware, most major tools.
Depending on your cost and performance goals, this may be a critical issue or of little concern. On a practical level, for most use cases, you need to keep in mind only two simple concepts:
Expect that additional data will be generated.
Know where the data is being stored, but don’t overthink it.
In the same way, we don’t really question how much storage, say, a PostgreSQL Write-Ahead Log (WAL) generates. My personal opinion is that it’s best to trust the training data tool in this regard. If there’s an issue, address it within the training data tool’s realm of influence.
External Reference Connection
A useful abstraction to create is a connection from the training data tooling to an existing external reference, e.g., a cloud bucket. There are various opinions on how to do this, and the specifics vary by hardware and cloud provider.
For the non-technical user, this is essentially “logging in” to a bucket. In other words, I create a new bucket, A, and it gets a user ID and password (client ID/client secret) from the IAM system. I pass those credentials to the training data tool and it stores them securely. Then, when needed, the training data tool uses those credentials to interact with the bucket.
Raw Media (BLOB)–Type Specific
A BLOB is a Binary Large Object. It is the raw data form of media, and is also known as an “object.” The technology that stores raw-data BLOBs is called a “bucket” or an “object store.” Depending on your media type, there will be specific concerns. Each media type will have vastly more concerns than can be listed here. You must research each type for your needs. A training data database will help format BLOBs to be useful for multiple end users, such as annotators and data scientists. Here, I note some of the most common considerations to be aware of.
Video
It’s common to split video files into smaller clips for easier annotation and processing. For example, a 10-minute video might be split into 60-second clips.
Sampling frames is one approach to reduce processing overhead. This can be done by reducing the frame rate and extracting frames. For example, you can convert a file from 30 frames per second (FPS) to 5 or 10 per second. A drawback is that losing too many frames may make it more difficult to annotate (for example, a key moment in the video may be cut off) or you may lose the ability to use other relevant video features like interpolation or tracking (that rely on assumptions around having a certain frame rate). Usually, it’s best to keep the video as a playable video and also extract all the frames required. This improves the end-user annotation experience and maximizes annotation ability.
Event-focused analytics need the exact frame when something happens, which effectively becomes lost if many frames are removed. Further, with all the frames intact, the full data is available to be sampled by “find interesting highlights” algorithms. This leads annotators to see more “interesting” things happening, and thus, higher quality data. Object tracking and interpolation further drive this point home, as an annotator may only need to label a handful of frames and can often get many back “for free” through those algorithms. And while, in practice, nearby frames are generally similar, it often still helps to have the extra data.
An exception to this is that sometimes, a very high FPS video (e.g., 240–480+) may still need to be sampled down to 120 FPS or similar. Note that just because many frames are available to be annotated, we can still choose to train models only on completed videos, completed frames, etc. If you must downsample the frames, use the global reference frame to maintain the mapping of the downsampled frame to the original frame.
Text
You will need to select your desired tokenizer or confirm that the tokenizer used by the system meets your needs. The tokenizer divides up words, for example based on spaces or using more complex algorithms, into smaller components. There are many open source tokenizers and a more detailed description of this is beyond the scope of this book. You may also need a process to convert BLOB files (e.g., .txt) into strings or vice versa.
Formatting and Mapping
Raw media is one part of the puzzle. Annotations and predictions are another big part. Think in terms of setting up data definitions rather than a one-time import. The better your definitions, the more easily data can flow between ML applications, and the more end users can update the data without engineering.
User-Defined Types (Compound Files)
Real-world cases often involve more than one file. For example, a driver’s license has a front and a back. We can think of creating a new user-defined type of “driver’s license” and then having it support two child files, each being an image. Or we can consider a “rich text” conversation that has multiple text files, images, etc.
Defining DataMaps
A DataMap handles the loading and unloading of definitions between applications. For example, it can load data to a model training system or a “What to Label” analyzer. Having these definitions well defined allows smooth integrations by end users, and decouples the need for engineering-level changes. In other words, it decouples when an application is called from the data definition itself. Examples include declaring a mapping between spatial locations formatted as x_min, y_min, x_max, y_max
and top_left, bottom_right
, or mapping integer results from a model back to a schema.
Ingest Wizards
One of the biggest gaps in tooling is often around the question, “How hard is it to set up my data in the system and maintain it?” Then comes what type of media can it ingest? How quickly can it ingest it?
This is a problem that’s still not as well defined as in other forms of software. You know when you get a link to a document and you load it for the first time? Or some big document starts to load on your computer?
Recently, new technology like “Import Wizards”—step-by-step forms—have come up that help make some of the data import process easier. While I fully expect these processes to continue to become even easier over time, the more you know about the behind-the-scenes aspects, the more you understand how these new wonderful wizards are actually working. This started originally with file browsers for cloud-based systems, and has progressed into full-grown mapping engines, similar to smart switch apps for phones, like the one that allows you to move all your data from Android to iPhone, or vice versa.
At a high level how it works is that a mapping engine (e.g., part of the ingest wizard) steps you through the process of mapping each field from one data source to another. Mapping wizards offer tremendous value. They save having to do a more technical integration. They typically provide more validations and checks to ensure the data is what you expect (picture seeing an email preview in Gmail before committing to open the email). And best of all, once the mappings are set up, they can easily be swapped out from a list without any context switching!
The impact of this is hard to overstate. Before, you may have been hesitant to, say, try a new model architecture, commercial prediction service, etc., because of the nuances of getting the data to and from it. This dramatically relieves that pressure.
What are the limitations of wizards? Well, first, some tools don’t support them yet, so they simply may not be available. Another issue is that they may impose technical limitations that are not present in more pure API calls or SDK integrations.
Organizing Data and Useful Storage
One of the first challenges is often how to organize the data you have already captured (or will capture). One reason this is more challenging than it may at first appear is that often these raw datasets are stored remotely.
At the time of writing, cloud data storage browsers are generally less mature than local file browsers. So even the most simple operations, e.g., me sitting at a screen and dragging files, can provide a new challenge.
Some practical suggestions here:
Try to get the data into your annotation tool sooner in the process than later. For example, at the same time new data comes in, I can write the data reference to the annotation tool at a similar time at which I’m writing to a generic object store. This way, I can “automatically” organize it to a degree, and/or more smoothly enlist team members to help with organization-level tasks.
Consider using tools that help surface the “most interesting” data. This is an emerging area—but it’s already clear that these methods, while not without their challenges, have merit and appear to be getting better.
Use tags. As simple as it sounds, tagging datasets with business-level organizational information helps. For example, the dataset “Train Sensor 12” can be tagged “Client ABC.” Tags can crosscut data science concerns and allow both business control/organizational and data science-level objectives.
Remote Storage
Data is usually stored remotely relative to the end user. This is because of the size of the data, security requirements, and automation requirements (e.g., connecting from an integrated program, practicalities of running model inference, aggregating data from nodes/system). Regarding working in teams, the person who administers the training data may not be the person who collected the data (consider use cases in medical, military, on-site construction, etc.).
This is relevant even for solutions with no external internet connection, also commonly referred to as “air-gapped” secret-level solutions. In these scenarios, it’s still likely the physical system that houses the data will be in a different location than the end user, even if they are sitting two feet from each other.
The implication of the data being elsewhere is that we now need a way to access it. At the very least, whoever is annotating the data needs access, and most likely some kind of data prep process will also require it.
Versioning
Versioning is important for reproducibility. That said, sometimes versioning gets a little too much attention. In practice, for most use cases, being mindful of the high-level concepts, using snapshots, and having good overall system of record definitions will get you very far.
There are three primary levels of data versioning, per instance (annotation), per file, and export. Their relation to each other is shown in Figure 4-5.
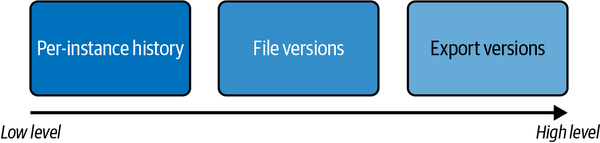
Figure 4-5. Versioning high-level comparison
Next, I’ll introduce each of these at a high level.
Per-instance history
By default, instances are not hard deleted. When an edit is made to an existing instance, Diffgram marks it as a soft delete and creates a new instance that succeeds it, as shown in Figure 4-6. You could, for example, use this for deep-dive annotation or model auditing. It is assumed that soft_deleted
instances are not returned when the default instance list is pulled for a file.
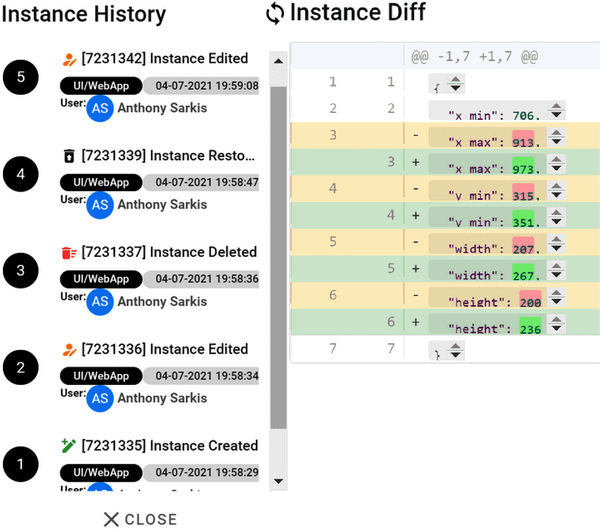
Figure 4-6. Left, per-instance history in UI; right, a single differential comparison between the same instance at different points in time
Per file and per set
Each set of tasks may be set to automatically create copies per file at each stage of the processing pipeline. This automatically maintains multiple file-level versions relevant to the task schema.
You may also programmatically and manually organize and copy data into sets on demand. You can filter data by tags, such as by a specific machine learning run. Then, compare across files and sets to see what’s changed.
Add files to multiple sets for cases where you want the files to always be on the latest version. That means you can construct multiple sets, with different criteria, and instantly have the latest version as annotation happens. Crucially, this is a living version, so it’s easy to always be on the “latest” one.
You can use these building blocks to flexibly manage versions across work in progress at the administrator level.
Per-export snapshots
With per-export snapshots, every export is automatically cached into a static file. This means you can take a snapshot at any moment, for any query, and have a repeatable way to access that exact set of data. This can be combined with webhooks, SDKs, or userscripts to automatically generate exports. These can be generated on demand, anytime. For example, you can use per-export snapshots to guarantee that a model is accessing the exact same data. The header of an export is shown as an example in Figure 4-7.

Figure 4-7. Export UI list view example
Next, we’ll cover exporting and accessing pattern trade-offs in more detail in Data Access.
Data Access
So far, we have covered overall architectural concepts, such as using a training data database to avoid a game of telephone. We covered the basics of getting started, media storage, mapping concepts, and BLOBs and other formats. Now we discuss the annotations themselves. A benefit of using a training data–centric approach is you get best practices, such as snapshots and streaming, built-in.
Streaming is a separate concept from querying, but it goes hand in hand in practical applications. For example, you may run a query that results in 1/100 of the data of a file-level export, and then stream that slice of the data directly in code.
There are some major concepts to be aware of:
File-based exports
Streaming
Querying data
Disambiguating Storage, Ingestion, Export, and Access
One way to think of this is that often, the way data is utilized in a database is different from how it’s stored at rest, and how it’s queried. Training data is similar. There are processes to ingest data, different processes to store it, and different ones again to query it:
Raw data storage refers to the literal storage of the BLOBs, and not the annotations, which are assumed to be kept in a separate database.
Ingestion is about the throughput, architecture, formats, and mapping of data. Often, this is between other applications and the training data system.
Export, in this context, usually refers to a one-time file-based export from the training data system.
Data access is about querying, viewing, and downloading BLOBs and annotations.
Modern training data systems store annotations in a database (not a JSON dump), and provide abstract query capabilities on those annotations.
File-Based Exports
As mentioned in versioning, a file-based export is a moment-in-time snapshot of the data. This is usually generated only on a very rough set of criteria, e.g., a dataset name. File-based exports are fairly straightforward, so I won’t spend much time on them. A comparison of trade-offs of file-based exporting and streaming is covered in the next section.
Streaming Data
Classically, annotations were always exported into a static file, such as a JSON file. Now, instead of generating each export as a one-off thing, you stream the data directly into memory. This brings up the question, “How to get my data out of the system?” All systems offer some form of export, but you’ll need to consider what kind. Is it a static, one-term export? Is it direct to TensorFlow or PyTorch memory?
Streaming benefits
You can load only what you need, which may be a small percent of a JSON file. At scale, it may be impractical to load all the data into a JSON file, so this may be a major benefit.
It works better for large teams. It avoids having to wait for static files; you can program and do work with the expected dataset before annotation starts, or even while annotation is happening.
It’s more memory efficient—because it’s streaming, you need not ever load the entire dataset into memory. This is especially applicable for distributed training, and when marshaling a JSON file would be impractical on a local machine.
It saves having “double mapping,” e.g., mapping to another format, which will itself then be mapped to tensors. In some cases too, parsing a JSON file can take even more effort than just updating a few tensors.
It provides more flexibility; the format can be defined and redefined by end users.
Streaming drawbacks
The specifications are defined in the code. If the dataset changes, reproducibility may be affected unless additional steps are taken.
It requires a network connection.
Some legacy training systems/AutoML providers may not support loading directly from memory and may require static files.
One thing to keep top of mind throughout this is that we don’t really want to statically select folders and files. We are really setting up a process in which we stream new data, in an event-driven way. To do this, we need to think of it more like assembling a pipeline, rather than focusing on the mechanics of getting a single existing known set.
Example: Fetch and stream
In this example, we will fetch a dataset and stream it using the Diffgram SDK:
pipinstall
diffgram
==
0
.15.0
from
diffgram
import
Project
project
=
Project
(
project_string_id
=
'your_project'
)
default_dataset
=
project
.
directory
.
get
(
name
=
'Default'
)
# Let's see how many images we got
(
'Number of items in dataset:
{}
'
.
format
(
len
(
default_dataset
)))
# Let's stream just the 8th element of the dataset
(
'8th element:
{}
'
.
format
(
default_dataset
[
7
]))
pytorch_ready_dataset
=
default_dataset
.
to_pytorch
()
Queries Introduction
Each application has its own query language. This language often has a special structure specific to the context of training data. It also may have support for abstract integrations with other query constructs.
To help frame this, let’s start with this easy example to get all files with more than 3 cars and at least 1 pedestrian (assuming those labels exist in your project):
dataset = project.dataset.get('my dataset') sliced_dataset = dataset.slice('labels.cars > 3 and labels.pedestrian >= 1')
Integrations with the Ecosystem
There are many applications available to perform model training and operation. At the time of writing, there are many hundreds of tools that fall in this category. As mentioned earlier, you can set up a mapping of definitions for formats, triggers, dataset names, and more in your training data tooling. We will cover these concepts in more depth in later chapters.
Security
The security of training data is of critical importance. Often, raw data is treated with greater scrutiny than data in other forms from a security viewpoint. For example, the raw data of critical infrastructure, driver’s licenses, military targets, etc. is very carefully stored and transferred.
Security is a broad topic that must be researched thoroughly and addressed separately from this book. However, we will address some issues that are crucial to understand when working with training data. I am calling attention specifically to the most common security items in the context of data engineering for training data:
Access control
Signed URLs
Personally identifiable information
Identity and Authorization
Production-level systems will often use OpenID Connect (OIDC). This can be coupled with role-based access control (RBAC) and attribute-based access control (ABAC).
Specifically with regard to training data, often the raw data is where there is the most tension around access. In that context, it can usually be addressed on either the per-file or per-set level. At the per-file level, access must be controlled by a policy engine that is aware of the triplicate of the {user, file, policy}
. This can be complex to administer at the per-file level. Usually, it is easier to achieve this at the per-set level. At the set (dataset) level, it is achieved with {user, set, policy}
.
Example of Setting Permissions
In this code sample, we will create a new dataset and a new security advisor role, and add the {view, dataset}
permission abstract object pair on that role:
restricted_ds1
=
project
.
directory
.
new
(
name
=
'Hidden Dataset 1'
,
access_type
=
'restricted'
)
advisor_role
=
project
.
roles
.
new
(
name
=
'security_advisor'
)
advisor_role
.
add_permission
(
perm
=
'dataset_view'
,
object_type
=
'WorkingDir'
)
We then assign the user (member) to the restricted dataset:
member_to_grant
=
project
.
get_member
(
=
'security_advisor_1@example.com'
)
advisor_role
.
assign_to_member_in_object
(
member_id
=
member
.
get
(
'member_id'
),
object_id
=
restricted_ds1
.
id
,
object_type
=
'WorkingDir'
)
Alternatively, this can be done with an external policy engine.
Signed URLs
Signed URLs are a technical mechanism to provide secure access to resources, most often raw media BLOBs. A signed URL is the output of a security process that involves identity and authorization steps. A signed URL is most concretely thought of as a one-time password to a resource, with the most commonly added caveat that it expires after a preset amount of time. This expiry time is sometimes as short as a few seconds, is routinely under one week, and rarely, the signed URL will be applicable “virtually indefinitely,” such as for multiple years. Signed URLs are not unique to training data, and you might benefit from further researching them, as they appear to be simple but contain many pitfalls. We touch on signed URLs here only in the context of training data.
One of the most critical things to be aware of is that because signed URLs are ephemeral, it is not a good idea to transmit signed URLs as a one-time thing. Doing so would effectively cripple the training data system when the URL expires. It is also less secure, since either the time will be too short to be useful, or too long to be secure. Instead, it is better to integrate them with your identity and authorization system. This way, signed URLs can be generated on demand by specific {User, Object/Resource}
pairs. That specific user can then get a short-expiring URL.
In other words, you can use a service outside the training data system to generate the signed URLs so long as the service is integrated directly with the training data system. Again, it’s important to move as much of the actual organizational logic and definitions inside the training data system as possible. Single sign-on (SSO) and identity and access–management integration commonly crosscut databases and applications, so that’s a separate consideration.
In addition to what we cover in this section, training data systems are currently offering new ways to secure data. This includes things like transmitting training data directly to ML programs, thus bypassing the need for a single person to have extremely open access. I encourage you to read the latest documentation from your training data system provider to stay up to date on the latest security best practices.
Cloud connections and signed URLs
Whoever is going to supervise the data needs to view it. This is the minimum level of access and is essentially unavoidable. The prep systems, such as the system that removes personally identifiable information (PII), generate thumbnails, pre-labels, etc., also need to see it. Also, for practical system-to-system communication, it’s often easier to transmit only a URL/file path and then have the system directly download the data. This is especially true because many end-user systems have much slower upload rates than download rates. For example, imagine saying “use the 48 GB video at this cloud path” (KBs of data) versus trying to transmit 48 GB from your home machine.
There are many ways to achieve this, but signed URLs—a per-resource password system—are currently the most commonly accepted method. They can be “behind the scenes,” but generally always end up being used in some form.
For both good and bad reasons, this can sometimes be an area of controversy. I’ll highlight some trade-offs here to help you decide what’s relevant for your system.
To sum up, training data presents some unusual data processing security concerns that must be kept in mind:
Humans see the “raw” data in ways that are uncommon in other systems.
Admins usually need fairly sweeping permissions to work with data, again in ways uncommon in classic systems.
Due to the proliferation of new media types, formats, and methods of data transmission, there are size and processing concerns with regard to training data. Although the concerns are similar to ones we’re familiar with from the classic systems, there are fewer established norms for what’s reasonable.
Personally Identifiable Information
Personally identifiable information must be handled with care when working with training data. Three of the most common ways to address PII concerns are to create a PII-compliant handling chain, to avoid it altogether, or to remove it.
PII-compliant data chain
Although PII introduces complications into our workflows, sometimes its presence is needed. Perhaps the PII is desired or useful for training. This requires having a PII-compliant data chain, PII training for staff, and appropriate tagging identifying the elements that contain PII. This also applies if the dataset contains PII and the PII will not be changed. The main factors to look at here are:
OAuth or similar Identify methods, like OIDC
On-premises or cloud-oriented installs
Passing by reference and not sending data
PII avoidance
It may be possible to avoid handling PII. Perhaps your customers or users can be the ones to actually look at their own PII. This may still require some level of PII compliance work, but less than if you or your team directly looks at the data.
PII removal
You may be able to strip, remove, or aggregate data to avoid PII. For example, the PII may be contained in metadata (such as DICOM). You may wish to either completely wipe this information, or retain only a single ID linking back to a separate database containing the required metadata. Or, the PII may be contained in the data itself, in which case other measures apply. For images, for example, this may involve blurring faces and identifying marks such as house numbers. This will vary dramatically based on your local laws and use case.
Pre-Labeling
Supervision of model predictions is common. It is used to measure system quality, improve the training data (annotation), and alert on errors. I’ll discuss the pros and cons of pre-labeling in later chapters; for now, I’ll provide a brief introduction to the technical specifics. The big idea with pre-labeling is that we take the output from an already-run model and surface it to other processes, such as human review.
Updating Data
It may seem strange to start with the update case, but since records will often be updated by ML programs, it’s good to have a plan for how updates will work prior to running models and ML programs.
If the data is already in the system, then you will need to refer to the file ID, or some other form of identification such as the filename, to match with the existing file. For large volumes of images, frequent updates, video, etc., it’s much faster to update an existing known record than to reimport and reprocess the raw data.
It’s best to have the definitions between ML programs and training data defined in the training data program.
If that is not possible, then at least include the training data file ID along with the data the model trains on. Doing this will allow you to more easily update the file later with the new results. This ID is more reliable than a filename because filenames are often only unique within a directory.
Pre-labeling gotchas
Some formats, for example video sequences, can be a little difficult to wrap one’s head around. This is especially true if you have a complex schema. For these, I suggest making sure the process works with an image, and/or the process works with a single default sequence, before trying true multiple sequences. SDK functions can help with pre-labeling efforts.
Some systems use relative coordinates, and some use absolute ones. It is easy to transform between these, as long as the height and width of the image are known. For example, a transformation from absolute to relative coordinates is defined as “x / image width” and “y / image height.” For example: A point x,y (120, 90) with an image width/height (1280, 720) would have a relative value of 120/1280 and 90/720 or (0.09375, 0.125).
If this is the first time you are importing the raw data, then it’s possible to attach the existing instances (annotations) at the same time as the raw data. If it’s not possible to attach the instances, then treat it as updating.
A common question is: “Should all machine predictions be sent to the training data database?” The answer is yes, as long as it is feasible. Noise is noise. There’s no point in sending known noisy predictions. Many prediction methods generate multiple predictions with some threshold for inclusion. Generally, whatever mechanism you have for filtering this data needs to be applied here too. For example, you might only take the highest “confidence” prediction. To this same end, in some cases, it can be very beneficial to include this “confidence” value or other “entropy” values to help better filter training data.
Pre-labeling data prep process
Now that we have covered some of the abstract concepts, let’s dive into some specific examples for selected media formats. We cannot cover all possible formats and types in this book, so you must research the docs for your specific training data system, media types, and needs.
Figure 4-8 shows an example pre-labeling process with three steps. It’s important to begin by mapping your data to the format of your system of record. Once your data has been processed, you’ll want to be sure to verify that everything is accurate.
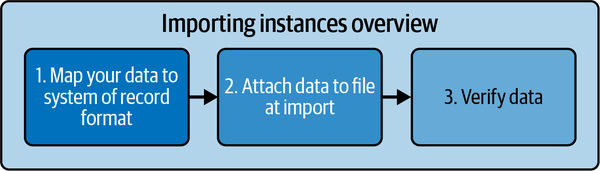
Figure 4-8. Block diagram example
Usually, there will be some high-level formatting information to note, such as saying that an image may have many instances associated with it, or that a video may have many frames, and each frame may have many instances, as shown in Figure 4-9.
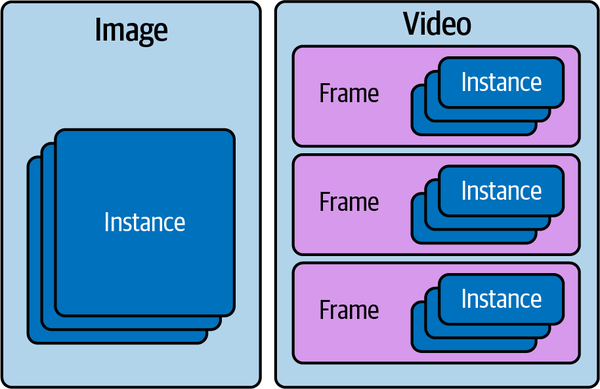
Figure 4-9. Visual overview of relationship between raw media and instances
Let’s put all of this together into a practical code example that will mock up data for an image bounding box.
Here is example Python code:
def
mock_box
(
sequence_number
:
int
=
None
,
name
:
str
=
None
):
return
{
"name"
:
name
,
"number"
:
sequence_number
,
"type"
:
"box"
,
"x_max"
:
random
.
randint
(
500
,
800
),
"x_min"
:
random
.
randint
(
400
,
499
),
"y_max"
:
random
.
randint
(
500
,
800
),
"y_min"
:
random
.
randint
(
400
,
499
)
}
This is one “instance.” So for example, running the function mock_box()
will yield the following:
instance
=
{
"name"
:
"Example"
,
"number"
:
0
,
"type"
:
"box"
,
"x_max"
:
500
,
"x_min"
:
400
,
"y_max"
:
500
,
"y_min"
:
400
}
We can combine instances into a list to represent multiple annotations on the same frame:
instance
=
{}
instance_list
=
[
instance
,
instance
,
instance
]
Summary
Great data engineering for training data requires a central system of record, raw data considerations, ingestion and query setups, defining access methods, securing data appropriately, and setting up pre-labeling (e.g., model prediction) integrations. There are some key points from this chapter that merit review:
A system of record is crucial to achieve great performance and avoid accumulating data errors like in a game of telephone.
A training data database is an example of a practical system of record.
Planning ahead for a training data system of record is ideal, but you can also add it to an existing system.
Raw data storage considerations include storage class, geolocation, cost, vendor support, and storing by reference versus by value.
Different raw data media types, like images, video, 3D, text, medical, and geospatial data have particular ingestion needs.
Queries and streaming provide more flexible data access than file exports.
Security aspects like access control, signed URLs, and PII must be considered.
Pre-labeling ideally loads model predictions into the system of record.
Mapping formats, handling updates, and checking accuracy are key parts of pre-labeling workflows.
Using the best practices in this chapter will help you on your path to improving your overall training data results and the machine learning model consumption of the data.
1 From Google Answers
Get Training Data for Machine Learning now with the O’Reilly learning platform.
O’Reilly members experience books, live events, courses curated by job role, and more from O’Reilly and nearly 200 top publishers.