The Internet of Things has four big data problems
The IoT and big data are two sides of the same coin; building one without considering the other is a recipe for doom.
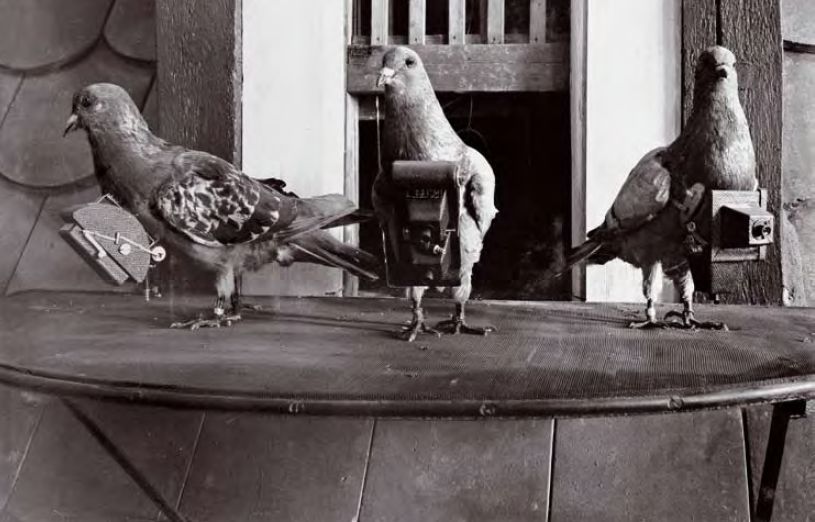
The Internet of Things (IoT) has a data problem. Well, four data problems. Walking the halls of CES in Las Vegas last week, it’s abundantly clear that the IoT is hot. Everyone is claiming to be the world’s smartest something. But that sprawl of devices, lacking context, with fragmented user groups, is a huge challenge for the burgeoning industry.
What the IoT needs is data. Big data and the IoT are two sides of the same coin. The IoT collects data from myriad sensors; that data is classified, organized, and used to make automated decisions; and the IoT, in turn, acts on it. It’s precisely this ever-accelerating feedback loop that makes the coin as a whole so compelling.
Nowhere are the IoT’s data problems more obvious than with that darling of the connected tomorrow known as the wearable. Yet, few people seem to want to discuss these problems:
Problem one: Nobody will wear 50 devices
If there’s one lesson today’s IoT start-ups have learned from their failed science project predecessors, it’s that things need to be simple and turnkey. As a result, devices are designed to do one thing really well. A corollary of this is that there’s far too much specialization happening — a device specifically, narrowly designed to measure sleep, or eating speed, or knee health.
Unfortunately, nobody’s going to charge, manage, and wear 50 devices, looking like a demented garage-sale cyborg. VentureBeat’s Harrison Weber managed to try on 56 different wearables at CES.
With this many competitors, the industry will crash. Wearables today are a digital quilt, a strange patchwork of point solutions trying to blanket a human life. To achieve simplicity, companies have over-focused on a single problem, or a single use case, deluding themselves that their beach-head is actually a sustainable market. The aisles of CES were littered with digital yoga mats, smart sun sensors, epilepsy detectors, and instrumented snowboard bindings.
Problem two: More inference, less sensing
Consider the aforementioned sun sensor. Do you really need a wristband that senses how much sunlight you’ve been exposed to? Or can your smartphone instead measure light levels periodically (which it does to determine screen brightness anyway), decide whether you’re outside, and check the UV index? The latter is inference, rather than sensing, and it’s probably good enough.
When the IoT sprawl finally triggers a mass extinction, only a few companies will survive. Many of the survivors will be the ones that can discover more information by inference, and that means teams that have a data science background.
Early versions of Jawbone’s wearable, for example, asked wearers to log their activity manually. More recent versions are smarter: the device notices a period of activity, guesses at what that activity was by comparing it to known patterns — were you playing basketball for a half hour? — and uses your response to either reinforce its guess, or to update its collective understanding of what basketball feels like.
Problem three: Datamandering
This sprawl of devices also means a sprawl of data. Unless you’re one of the big wearable players — Jawbone, Fitbit, Withings and a handful of others — you probably don’t have enough user data to make significant breakthrough discoveries about your users’ lives. This gives the big players a strong first-mover advantage.
When the wearables sector inevitably consolidates, all the data that failed companies collected will be lost. There’s little sharing of information across product lines, and export is seldom more than a comma-separated file.
Consider that one of the strongest reasons people don’t switch from Apple to Android is the familiarity of the user experience and the content in iTunes. Similarly, in the IoT world, interfaces and data discourage switching. Unfortunately, this means constant wars over data formats in a strange kind of digital jerrymandering — call it datamandering — as each vendor jockeys for position, trying to be the central hub of our health, parenting, home, or finances.
As Samsung CEO BK Yoon said in his CES keynote, “I’ve heard people say they want to create a single operating system for the Internet of Things, but these people only work with their own devices.”
Walking CES, you see hundreds of manufacturers from Shenzhen promoting the building blocks of the IoT. Technologies like fabric sensors — which only months ago were freshly released from secret university labs and lauded on tech blogs — can now be had at scale from China. Barriers to entry crumble fast. What remains for IoT companies are attention, adoption, and data.
When technical advances erode quickly, companies have little reason to cooperate on the data they collect. There’s no data lake in wearables, just myriad jealously guarded streams.
Problem four: Context is everything
If data doesn’t change your behavior, why bother collecting it? Perhaps the biggest data problem the IoT faces is correlating the data it collects with actions you can take. Consider V1bes, which calls itself a “mind app.” It measures stress levels and brain activity. Sociometric Solutions does the same thing by listening to the tone of my voice, and can predict my stress levels accurately.
That sounds useful: it’d be great to see how stressed I was at a particular time, or when my brain was most active. But unless I can see the person to whom I was talking, or hear the words I was thinking about, at that time, it’s hard to do anything about it. The data tells me I’m stressed; it doesn’t tell me who’s triggering my chronic depression or who makes my eyes light up.
There might be hope here. If I had a photo stream of every day, and with it a voice recorder, I might be able to see who I was with (and whom to avoid). Start-ups like Narrative Clip, which constantly logs my life by taking a photo every 30 seconds and using algorithms to decide which of those photos are interesting, might give me a clue about what triggered my stress. And portable recorders like Kapture can record conversations with time stamps; their transcripts, analyzed, could help me understand how I react to certain topics.
Ultimately, it’s clear that the Internet of Things is here to stay. We’re in the midst of an explosion of ideas, but many of them are stillborn, either too specific or too disconnected from the context of our lives to have true meaning. The Internet of Things and big data are two sides of the same coin, and building one without considering the other is a recipe for doom.
This post is part of our investigations into Big Data Sensor Networks and Distributed Computation, Big Data Design and Social Science, and Interaction Design and Connected Devices. For more on how sensors, fast networks, AI, and distributed computing are affecting the data landscape, download our free report: Data: Emerging Trends and Technologies, by Alistair Croll.