Chapter 4. Sensemaking
In March 2011, David Moore published a sensemaking manual to help central intelligence agencies reduce mindlessness, a mode of fixation and relaxation where analysts became âso preoccupied with a few central signals that they largely ignored things in their periphery.â1
Moore observed that analysts had developed an automatic response to the signals they were seeing, which prevented them from uncovering new insights. To keep up with the wealth of incoming data, they began to focus on specific signals, leaving themselves vulnerable to new threats they hadnât considered.
As you begin to conduct interviews, surveys, concept value tests, and other experiments, your team will have a flood of data points. It can be hard to gain broad insights from all of these signals and, just like intelligence analysts, your team will be susceptible to focusing on just a few signals, missing out on larger opportunities for your products.
Sensemaking is a process that ensures we organize the data we collect, identify patterns and meaningful insights, and refine them into a story that compels others to action.
The Sensemaking Loop
Sensemaking is a perpetual cycle of collecting data, making sense out of it, and sharing knowledge throughout our teams and organizations. Pirolli and Card refer to this as the âsensemaking loop.â2 It essentially breaks down into five procedural components (see Figure 4-1):
Data sources
Shoebox
Evidence file
Schema
Stories
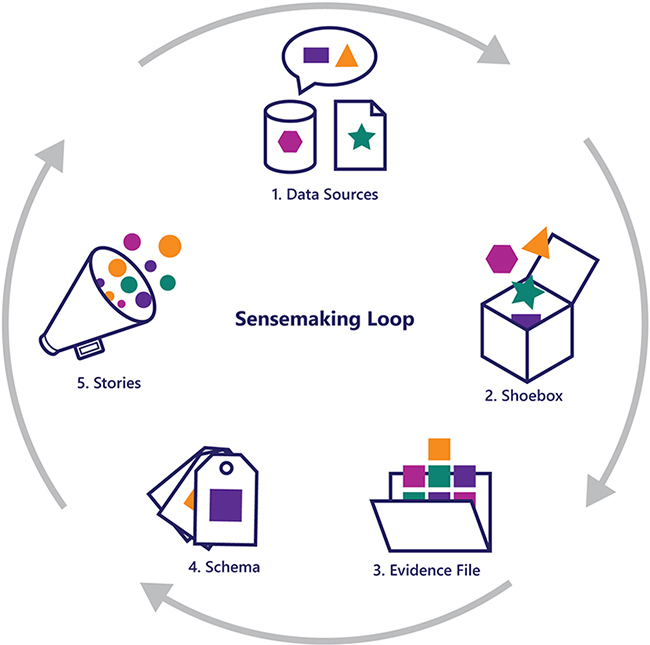
Figure 4-1. The sensemaking loop
These components come together to help you transition raw customer data into meaningful, âstickyâ insights. Youâll find that, in our playbooks, we provide activities that help you navigate this loop by taking your data, curating an evidence file, creating schemas, and crafting a compelling story to share throughout your organization.
You can think of the sensemaking loop as the components youâll need to make an effective case for your productâs strategy. The hypotheses you create with the HPF will be the backbone of this loop.
Sensemaking is a continuous cycle that we use at every stage of the HPF. Each stage will manifest its own insights. While it isnât close to an exhaustive list, Table 4-1 gives some examples.
As you engage in sensemaking, youâll find that itâs not a linear path, where you move from collecting data to sharing your knowledge. Instead, youâll move back and forth within the loopâcollecting data, identifying patterns, sharing stories, and returning to collect more data.
Put another way, sensemaking isnât a destination, where you try to reach the end of the loop; itâs a continual process that ensures consistent learning and a fully developed understanding of your customers.
Letâs look at each component of the loop more closely.
Data Sources
Weâve talked about the various methods you can employ to validate your hypotheses. While most of the types of experiments weâve discussed have talking to customers at their heart, your customer and product development strategy should pull from multiple data sources.
Usage statistics, discussion forums, support tickets, and customer relationship management (CRM) systems are all great sources for data. You can also forage through market trend analysis reports, conduct a competitive analysis, or go on a customer visit. The important thing here is that while youâre making sense of the data youâre collecting, youâre also tracking the sources of that data.
Shoeboxes
Before the advent of cloud storage and digital photography, the shoebox was the de facto storage method for photos. Shoeboxes were great because they required little to no organization (you could just wrap a rubber band around the photos from your summer vacation), and they kept all your photos secure and in the same place.
As you begin to cull your data sources, youâll collect notes, articles, and other assets that comprise your area of study. There are many online collaborative âshoeboxesâ to store these types of things. Microsoft SharePoint, OneNote, Evernote, Basecamp, Google Drive, and Dropbox are all tools that allow teams to collect the information theyâre gathering. You shouldnât spend too much time curating or organizing your shoebox. This should be a loose inventory of any data youâve collectedâimportant or unimportant.
Evidence Files
When youâre on a customer or product development journey, youâre running an investigation. Your evidence file is like a case file: it contains the meaningful bits of data that comprise your point of view, vision, or strategy for your product.
An evidence file could include pictures of a customerâs environment, a direct customer quote, or any other type of signal that points to why you validated or invalidated your hypotheses. For example, you could begin to capture direct quotes from your customer interviews that highlight a particular motivation or problem you hypothesized might exist.
These evidence files should be constantly culled, organized, and reflected upon, as they are the foundation for the case youâre trying to make on behalf of your customer. They can help the team stay organized and up-to-date on the latest findings.
As you begin to curate your evidence files, youâll find yourself adding pieces of evidence that your gut tells you are meaningful but youâre not yet sure why. Your ability to clearly articulate the underlying meaning will evolve as the evidence file takes shape. Youâll find yourself continually moving things back and forth between your shoebox and evidence file until youâve refined your collection to its most impactful bits of data.
When starting your project, you may find that all the data youâve collected feels meaningful, and thatâs okay. As your project matures, youâll need a way to reduce your data signals and separate the âwheat from the chaff.â What you believe to be most meaningful will evolve over time, and so should your evidence file.
Schemas
Schematizing data is the process of applying categories and patterns to your data. We often refer to this as âtagging your data,â giving it meaning and defining it.
For example, you may mark a quote with a âproblemâ tag when a customer expresses a frustration. This will help you look at all your interviews and identify each time a frustration was articulated.
These tags will help you see patterns in your data and begin to draw conclusions.
Again, the parameters within the HPF automatically get you started. If your hypotheses and Discussion Guides are formulated to capture parameters like job-to-be-done, problem, or motivation, it can be much easier to begin tagging your data using those parameters.
Weâve repeatedly seen teams create spreadsheets to connect the data theyâve collected to their hypotheses. Some spreadsheets are quite simple, tracking the status of hypotheses. Others are more elaborate, containing dashboard-like interfaces with counters and formatting that change the status of a hypothesis from green to red based on the number of times it has been validated or invalidated.
Weâve also seen teams create hypotheses backlogs that help them track the various hypotheses the team might be exploring (and remember others they stopped exploring).
Stories
There are two things you need to get others onboard with your vision:
A compelling story
A way to share it
The most important thing about sensemaking is that it helps you share meaning, not data. Data is important, but emotion and empathy are what compel others to action.
As you begin to identify patterns, youâll need ways to express your data so that people can easily understand it. Visual elements like charts, graphs, and models can be a powerful way to help others understand what youâve learned.
You can certainly take the quantitative data youâve collected and transfer it into pie charts or line graphs, but you should look for more illustrative models as well. For example, you may have identified that there is a tension between customers wanting quality service providers and saving money. Perhaps this tension changes, depending on the type of service the customer is looking for. A customer might be looking to save money when researching lawn care service, but willing to pay much more for quality childcare.
Illustrating these nuances in graphical models can help others easily understand relationships and connections in your data.
Analogies and metaphors are also powerful tools to help convey complex ideas to others. Look for opportunities where your findings parallel other situations that might be more familiar and accessible to them. For example, one of our teams identified parallels between people trying to learn a new programming language and people learning to swim. This comparison helped the rest of the organization empathize with how difficult the challenges were without requiring knowledge about the programming language.
Once you have a compelling story, you need a way to share it with others in your organization. Youâll want to create a continuous communication channel (or multiple channels) thatâs easy to use and accessible to everyone. Weâve found the less formal and lightweight the communication channel is, the more likely people are to use it. Leverage existing channels like email or chat clients so you donât have to encourage your organization to use another channel they can easily ignore.
Throughout the stages in the HPF, you should fall into a pattern of continual sensemaking; these activities should be happening, in parallel, with your customer and product development. You can do this by splitting the teamsâ efforts or scheduling a day each week, during development, to stop and make sense of the data youâve been collecting.
Over time, the continual pattern of the sensemaking loop will increase your understanding and the overall empathy of your organization toward its customers.
At this point, weâve covered the three phases of the Customer-Driven Cadence: Formulating, Experimenting, and Sensemaking. This cadence happens in each stage of the HPF. You formulate your assumptions into hypotheses, you run experiments to collect data, and you make sense of that data to gain insights.
Now, weâre going to dive into each stage of the HPF and examine the hypotheses and parameters we use to drive us toward better understanding our customers, their problems, and what they find valuable and useful.
Key Points
Sensemaking is the process you go through to take your raw customer data and convert it into meaningful results that can be shared with others.
The sensemaking loop is a series of components that make up the sensemaking processes. These components include external data sources, shoeboxes, evidence files, schemas, and stories.
External data sources are an inventory of the sources of the data youâre collecting. While our playbooks focus primarily on direct customer interactions, you may also have supporting data coming from market reports, competitive analysis, discussion forums, support tickets, or customer relationship management systems.
The shoebox is the location where you store all your interview notes, articles, pictures, or any other assets that have been generated throughout your customer and product development.
The evidence file is your curated and organized location for the findings that validate or invalidate your hypotheses. Much like investigators, you will need an evidence file to help your team make their case. Some teams use spreadsheet software, formulas, and special formatting to connect their data points to a validated or invalidated hypothesis.
Storytelling is an essential component of the customer-driven processes. By using charts, graphs, models, and illustrations, you can share your findings with a broader audience. Metaphors and analogies can be used to draw parallels between a complex learning and something that is more accessible or understandable to your audience.
To surface your learnings, youâll need to support multiple channels for sharing information. Some teams use daily or weekly standups, monthly newsletters, or email aliases where team members can share their customer interview notes.
Get The Customer-Driven Playbook now with the O’Reilly learning platform.
O’Reilly members experience books, live events, courses curated by job role, and more from O’Reilly and nearly 200 top publishers.