Chapter 1. Training Data Introduction
Data is all around us—videos, images, text, documents, as well as geospatial, multi-dimensional data, and more. Yet, in its raw form, this data is of little use to supervised machine learning (ML) and artificial intelligence (AI). How do we make use of this data? How do we record our intelligence so it can be reproduced through ML and AI? The answer is the art of training data—the discipline of making raw data useful.
In this book you will learn:
-
All-new training data (AI data) concepts
-
The day-to-day practice of training data
-
How to improve training data efficiency
-
How to transform your team to be more AI/ML-centric
-
Real-world case studies
Before we can cover some of these concepts, we first have to understand the foundations, which this chapter will unpack.
Training data is about molding, reforming, shaping, and digesting raw data into new forms: creating new meaning out of raw data to solve problems. These acts of creation and destruction sit at the intersection of subject matter expertise, business needs, and technical requirements. It’s a diverse set of activities that crosscut multiple domains.
At the heart of these activities is annotation. Annotation produces structured data that is ready to be consumed by a machine learning model. Without annotation, raw data is considered to be unstructured, usually less valuable, and often not usable for supervised learning. That’s why training data is required for modern machine learning use cases including computer vision, natural language processing, and speech recognition.
To cement this idea in an example, let’s consider annotation in detail. When we annotate data, we are capturing human knowledge. Typically, this process looks as follows: a piece of media such as an image, text, video, 3D design, or audio, is presented along with a set of predefined options (labels). A human reviews the media and determines the most appropriate answers, for example, declaring a region of an image to be “good” or “bad.” This label provides the context needed to apply machine learning concepts (Figure 1-1).
But how did we get there? How did we get to the point that the right media element, with the right predefined set of options, was shown to the right person at the right time? There are many concepts that lead up to and follow the moment where that annotation, or knowledge capture, actually happens. Collectively, all of these concepts are the art of training data.
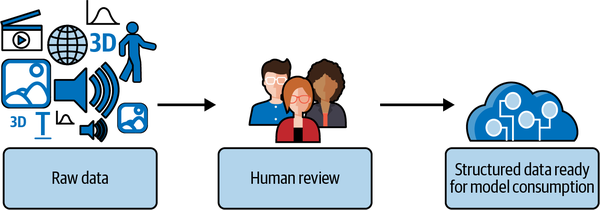
Figure 1-1. The training data process
In this chapter, we’ll introduce what training data is, why it matters, and dive into many key concepts that will form the base for the rest of the book.
Training Data Intents
The purpose of training data varies across different use cases, problems, and scenarios. Let’s explore some of the most common questions, like what can you do with training data? What is it most concerned with? What are people aiming to achieve with training data?
What Can You Do With Training Data?
Training data is the foundation of AI/ML systems—the underpinning that makes these systems work.
With training data, you can build and maintain modern ML systems, such as ones that create next-generation automations, improve existing products, and even create all-new products.
In order to be most useful, the raw data needs to be upgraded and structured in a way that is consumable by ML programs. With training data, you are creating and maintaining the required new data and structures, like annotations and schemas, to make the raw data useful. Through this creation and maintenance process, you will have great training data, and you will be on the path toward a great overall solution.
In practice, common use cases center around a few key needs:
-
Improving an existing product (e.g., performance), even if ML is not currently a part of it
-
Production of a new product, including systems that run in a limited or “one-off” fashion
-
Research and development
Training data transcends all parts of ML programs:
-
Training a model? It requires training data.
-
Want to improve performance? It requires higher-quality, different, or a higher volume of training data.
-
Made a prediction? That’s future training data that was just generated.
Training data comes up before you can run an ML program; it comes up during running in terms of output and results, and even later in analysis and maintenance. Further, training data concerns tend to be long-lived. For example, after getting a model up and running, maintaining the training data is an important part of maintaining a model. While, in research environments, a single training dataset may be unchanged (e.g., ImageNet), in industry, training data is extremely dynamic and changes often. This dynamic nature puts more and more significance on having a great understanding of training data.
The creation and maintenance of novel data is a primary concern of this book. A dataset, at a moment in time, is an output of the complex processes of training data. For example, a Train/Test/Val split is a derivative of an original, novel set. And that novel set itself is simply a snapshot, a single view into larger training data processes. Similarly to how a programmer may decide to print or log a variable, the variable printed is just the output; it doesn’t explain the complex set of functions that were required to get the desired value. A goal of this book is to explain the complex processes behind getting usable datasets.
Annotation, the act of humans directly annotating samples, is the “highest” part of training data. By highest, I mean that human annotation works on top of the collection of existing data (e.g., from BLOB storages, existing databases, metadata, websites).1 Human annotation is also the overriding truth on top of automation concepts like pre-labeling and other processes that generate new data like predictions and tags. These combinations of “high-level” human work, existing data, and machine work form a core of the much broader concepts of training data outlined later in this chapter.
What Is Training Data Most Concerned With?
This book covers a variety of people, organizational, and technical concerns. We’ll walk through each of these concepts in detail in a moment, but before we do, let’s think about areas training data is focused on.
For example, how does the schema, which is a map between your annotations and their meaning for your use case, accurately represent the problem? How do you ensure raw data is collected and used in a way relevant to the problem? How are human validation, monitoring, controls, and correction applied?
How do you repeatedly achieve and maintain acceptable degrees of quality when there is such a large human component? How does it integrate with other technologies, including data sources and your application?
To help organize this, you can broadly divide the overall concept of training data into the following topics: schema, raw data, quality, integrations, and the human role. Next, I’ll take a deeper look at each of those topics.
Schema
A schema is formed through labels, attributes, spatial representations, and relations to external data. Annotators use the schema while making annotations. Schemas are the backbone of your AI and central in every aspect of training data.
Conceptually, a schema is the map between human input and meaning for your use case. It defines what the ML program is capable of outputting. It’s the vital link, it’s what binds together everyone’s hard work. So to state the obvious, it’s important.
A good schema is useful and relevant to your specific need. It’s usually best to create a new, custom schema, and then keep iterating on it for your specific cases. It’s normal to draw on domain-specific databases for inspirations, or to fill in certain levels of detail, but be sure that’s done in the context of guidance for a new, novel, schema. Don’t expect an existing schema from another context to work for ML programs without further updates.
So, why is it important to design it according to your specific needs, and not some predefined set?
First, the schema is for both human annotation and ML use. An existing domain-specific schema may be designed for human use in a different context or for machine use in a classic, non-ML context. This is one of those cases where two things might seem to produce similar output, but the outcome is actually formed in totally different ways. For example, two different math functions might both output the same value, but run on completely different logic. The output of the schema may appear similar, but the differences are important to make it friendly to annotation and ML use.
Second, if the schema is not useful, then even great model predictions are not useful. Failure with schema design likely will cascade to failure of the overall system. The context here is that ML programs can usually only make predictions based on what is included in the schema.2 It’s rare that an ML program will produce relevant results that are better than the original schema. It’s also rare that it will predict something that a human, or group of humans, looking at the same raw data could not also predict.
It is common to see schemas that have questionable value. So, it’s really worth stopping and thinking “If we automatically got the data labeled with this schema, would it actually be useful to us?” and “Can a human looking at the raw data reasonably choose something from the schema that fits it?”
In the first few chapters, we will cover the technical aspects of schemas, and we will come back to schema concerns through practical examples later in the book.
Raw data
Raw data is any form of Binary Large Object (BLOB) data or pre-structured data that is treated as a single sample for purposes of annotation. Examples include videos, images, text, documents, and geospatial and multi-dimensional data. When we think about raw data as part of training data, the most important thing is that the raw data is collected and used in a way relevant to the schema.
To illustrate the idea of the relevance of raw data to a schema, let’s consider the difference between hearing a sports game on the radio, seeing it on TV, or being at the game in person. It’s the same event regardless of the medium, but you receive a very different amount of data in each context. The context of the raw data collection, via TV, radio, or in person, frames the potential of the raw data. So, for example, if you were trying to determine possession of the ball automatically, the visual raw data will likely be a better fit then the radio raw data.
Compared to software, we humans are good at automatically making contextual correlations and working with noisy data. We make many assumptions, often drawing on data sources not present in the moment to our senses. This ability to understand the context above the directly sensed sights, sounds, etc. makes it difficult to remember that software is more limited here.
Software only has the context that is programmed into it, be it through data or lines of code. This means the real challenge with raw data is overcoming our human assumptions around context to make the right data available.
So how do you do that? One of the more successful ways is to start with the schema, and then map ideas of raw data collection to that. It can be visualized as a chain of problem -> schema -> raw data. The schema’s requirements are always defined by the problem or product. That way there is always this easy check of “Given the schema, and the raw data, can a human make a reasonable judgment?”
Centering around the schema also encourages thinking about new methods of data collection, instead of limiting ourselves to existing or easiest-to-reach data collection methods. Over time, the schema and raw data can be jointly iterated on; this is just to get started. Another way to relate the schema to the product is to consider the schema as representing the product. So to use the cliché of “product market fit,” this is “product data fit.”
To put the above abstractions into more concrete terms, we’ll discuss some common issues that arise in industry. Differences between data used during development and production is one of the most common sources of errors. It is common because it is somewhat unavoidable. That’s why being able to get to some level of “real” data early in the iteration process is crucial. You have to expect that production data will be different, and plan for it as part of your overall data collection strategy.
The data program can only see the raw data and the annotations—only what is given to it. If a human annotator is relying on knowledge outside of what can be understood from the sample presented, it’s unlikely the data program will have that context, and it will fail. We must remember that all needed context must be present, either in the data or lines of code of the program.
To recap:
-
The raw data needs to be relevant to the schema.
-
The raw data should be as similar to production data as possible.
-
The raw data should have all the context needed in the sample itself.
Annotations
Each annotation is a single example of something specified in the schema. Imagine two cliffs with an open space in the middle, the left representing the schema, and the right a single raw data file. An annotation is the concrete bridge between the schema and raw data, as shown in Figure 1-2.
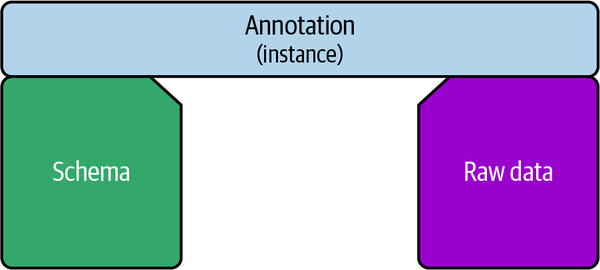
Figure 1-2. Relationships among schema, single annotation, and raw data
While the schema is “abstract,” meaning that it is referenced and reused between multiple annotations, each annotation has the actual specific values that fill in the answers to the questions in the schema.
Annotations are usually the most numerous form of data in a training data system because each file often has tens, or even hundreds, of annotations. An annotation is also known as an “instance” because it’s a single instance of something in the schema.
More technically, each annotation instance usually contains a key to relate it to a label or attribute within a schema and a file or child file representing the raw data. In practical terms, each file will usually contain a list of instances.
Quality
Training data quality naturally occurs on a spectrum. What is acceptable in one context may not be in another.
So what are the biggest factors that go into training data quality? Well, we already talked about two of them: schema and raw data. For example:
-
A bad schema may cause more quality issues than bad annotators.
-
If the concept is not clear in the raw data sample, it’s unlikely it will be clear to the ML program.
Often, annotation quality is the next biggest item. Annotation quality is important, but perhaps not in the ways you may expect. Specifically, people tend to think of annotation quality as “was it annotated right?” But “right” is often out of scope. To understand how the “right” answer is often out of scope, let’s imagine we are annotating traffic lights, and the light in the sample you are presented with is off (e.g., a power failure) and your only options from the schema are variations on an active traffic light. Clearly, either the schema needs to be updated to include an “off” traffic light, or our production system will never be usable in a context where a traffic light may have a power failure.
To move into a slightly harder-to-control case, consider that if the traffic light is really far away or at an odd angle, that will also limit the worker’s ability to annotate it properly. Often, these cases sound like they should be easily manageable, but in practice they often aren’t. So more generally, real issues with annotation quality tend to circle back to issues with the schema and raw data. Annotators surface problems with schemas and data in the course of their work. High-quality annotation is as much about the effective communication of these issues rather than exclusively annotating “correctly.”
I can’t emphasize enough that schema and raw data deserve a lot of attention. However, annotating correctly does still matter, and one of the approaches is to have multiple people look at the same sample. This is often costly, and someone must interpret the meaning of the multiple opinions on the same sample, adding further cost. For an industry-usable case, where the schema has a reasonable degree of complexity, the meta-analysis of the opinions is a further time sink.
Think of a crowd of people watching a sports game instant replay. Imagine trying to statistically sample their opinions to get a “proof” of what is “more right.” Instead of this, we have a referee who individually reviews the situation and makes a determination. The referee may not be “right” but, for better or worse, the social norm is to have the referee (or a similar process) make the call.
Similarly, often a more cost-effective approach is used. A percent of the data is sampled randomly for a review loop, and annotators raise issues with the schema and raw data fit, as they occur. This review loop and quality assurance processes will be discussed in more depth later.
If the review method fails, and it seems you would still need multiple people to annotate the same data in order to ensure high quality, you probably have a bad product data fit, and you need to change the schema or raw data collection to fix it.
Zooming out from schema, raw data, and annotation, the other big aspects of quality are the maintenance of the data and the integration points with ML programs. Quality includes cost considerations, expected use, and expected failure rates.
To recap, quality is first and foremost formed by the schema and raw data, then by the annotators and associated processes, and rounded out by maintenance and integration.
Integrations
Much time and energy are often focused on “training the model.” However, because training a model is a primarily technical data-science-focused concept, it can lead us to underemphasize other important aspects of using the technology effectively.
What about maintenance of the training data? What about ML programs that output useful training data results, such as sampling, finding errors, reducing workload, etc., that are not involved with training a model? How about the integration with the application that the results of the model or ML subprogram will be used in? What about tech that tests and monitors datasets? The hardware? Human notifications? How the technology is packaged into other tech?
Training the model is just one component. To successfully build an ML program, a data-driven program, we need to think about how all the technology components work together. And to hit the ground running, we need to be aware of the growing training data ecosystem. Integration with data science is multifaceted, it’s not just about some final “output” of annotations. It’s about the ongoing human control, maintenance, schema, validation, lifecycle, security, etc. A batch of outputted annotations is like the result of a single SQL query, it’s a single, limited view into a complex database.
A few key aspects to remember about working with integrations:
-
The training data is only useful if it can be consumed by something, usually within a larger program.
-
Integration with data science has many touch points and requires big-picture thinking.
-
Getting a model trained is only a small part of the overall ecosystem.
The human role
Humans affect data programs by controlling the training data. This includes determining the aspects we have discussed so far, the schema, raw data, quality, and integrations with other systems. And of course, people are involved with annotation itself, when humans look at each individual sample.
This control is exercised at many stages, and by many people, from establishing initial training data to performing human evaluations of data science outputs and validating data science results. This large volume of people being involved is very different from classic ML.
We have new metrics, like how many samples were accepted, how long is spent on each task, the lifecycles of datasets, the fidelity of raw data, what the distribution of the schema looks like, etc. These aspects may overlap with data science terms, like class distribution, but are worth thinking of as separate concepts. For example, model metrics are based on the ground truth of the training data, so if the data is wrong, the metrics are wrong. And as discussed in “Quality Assurance Automation”, metrics around something like annotator agreement can miss larger points of schema and raw data issues.
Human oversight is about so much more than just quantitative metrics. It’s about qualitative understanding. Human observation, human understanding of the schema, raw data, individual samples, etc., are of great importance. This qualitative view extends into business and use case concepts. Further, these validations and controls quickly extend from being easily defined, to more of an art form, acts of creation. This is not to mention the complicated political and social expectations that can arise around system performances and output.
Working with training data is an opportunity to create: to capture human intelligence and insights in novel ways; to frame problems in a new training data context; to create new schemas, to collect new raw data, and use other training data–specific methods.
This creation, this control, it’s all new. While we have established patterns for various types of human–computer interaction, there is much less established for human-ML program interactions—for human supervision, a data-driven system, where the humans can directly correct and program the data.
For example, we expect an average office worker to know how to use word processing, but we don’t expect them to use video editing tools. Training data requires subject matter experts. So in the same way a doctor must know how to use a computer for common tasks today, they must now learn how to use standard annotation patterns. As human-controlled data-driven programs emerge and become more common, these interactions will continue to increase in importance and variance.
Training Data Opportunities
Now that we understand many of the fundamentals, let’s frame some opportunities. If you’re considering adding training data to your ML/AI program, some questions you may want to ask are:
-
What are the best practices?
-
Are we doing this the “right” way?
-
How can my team work more efficiently with training data?
-
What business opportunities can training data–centric projects unlock?
-
Can I turn an existing work process, like an existing quality assurance pipeline, into training data? What if all of my training data could be in one place instead of shuffling data from A to B to C? How can I be more proficient with training data tools?
Broadly, a business can:
-
Increase revenue by shipping new AI/ML data products.
-
Maintain existing revenue by improving the performance of an existing product through AI/ML data.
-
Reduce security risks—reduce risks and costs from AI/ML data exposure and loss.
-
Improve productivity by moving employee work further up the automation food chain. For example, by continuously learning from data, you can create your AI/ML data engine.
All of these elements can lead to transformations through an organization, which I’ll cover next.
Business Transformation
Your team and company’s mindset around training data is important. I’ll provide more detail in Chapter 7, but for now, here are some important ways to start thinking about this:
-
Start viewing all existing routine work at the company as an opportunity to create training data.
-
Realize that work not captured in a training data system is lost.
-
Begin shifting annotation to be part of every frontline worker’s day.
-
Define your organizational leadership structures to better support training data efforts.
-
Manage your training data processes at scale. What works for an individual data scientist might be very different from what works for a team, and different still for a corporation with multiple teams.
In order to accomplish all of this, it’s important to implement strong training data practices within your team and organization. To do this, you need to create a training data–centric mindset at your company. This can be complex and may take time, but it’s worth the investment.
To do this, involve subject matter experts in your project planning discussions. They’ll bring valuable insights that will save your team time downstream. It’s also important to use tools to maintain abstractions and integrations for raw data collection, ingress, and egress. You’ll need new libraries for specific training data purposes so you can build on existing research. Having the proper tools and systems in place will help your team perform with a data-centric mindset. And finally, make sure you and your teams are reporting and describing training data. Understanding what was done, why it was done, and what the outcomes were will inform future projects.
All of this may sound daunting now, so let’s break things down a step further. When you first get started with training data, you’ll be learning new training data–specific concepts that will lead to mindset shifts. For example, adding new data and annotations will become part of your routine workflows. You’ll be more informed as you get initial datasets, schemas, and other configurations set up. This book will help you become more familiar with new tools, new APIs, new SDKs, and more, enabling you to integrate training data tools into your workflow.
Training Data Efficiency
Efficiency in training data is a function of many parts. We’ll explore this in greater detail in the chapters to come, but for now, consider these questions:
-
How can we create and maintain better schemas?
-
How can we better capture and maintain raw data?
-
How can we annotate more efficiently?
-
How can we reduce the relevant sample counts so there is less to annotate in the first place?
-
How can we get people up to speed on new tools?
-
How can we make this work with our application? What are the integration points?
As with most processes, there are a lot of areas to improve efficiency, and this book will show you how sound training data practices can help.
Tooling Proficiency
New tools, like Diffgram, HumanSignal, and more now offer many ways to help realize your training data goals. As these tools grow in complexity, being able to master them becomes more important. You may have picked up this book looking for a broad overview, or to optimize specific pain points. Chapter 2 will discuss tools and trade-offs.
Process Improvement Opportunities
Consider a few common areas people want to improve, such as:
-
Annotation quality being poor, too costly, too manual, too error prone
-
Duplicate work
-
Subject matter expert labor cost too high
-
Too much routine or tedious work
-
Nearly impossible to get enough of the original raw data
-
Raw data volume clearly exceeding any reasonable ability to manually look at it
You may want a broader business transformation, to learn new tools, or to optimize a specific project or process. The question is, naturally, what’s the next best step for you to take, and why should you take it? To help you answer that, let’s now talk about why training data matters.
Why Training Data Matters
In this section, I’ll cover why training data is important for your organization, and why a strong training data practice is essential. These are central themes throughout the book, and you’ll see them come up again in the future.
First, training data determines what your AI program, your system, can do. Without training data, there is no system. With training data, the opportunities are only bounded by your imagination! Sorta. Well, okay, in practice, there’s still budget, resources such as hardware, and team expertise. But theoretically, anything that you can form into a schema and record raw data for, the system can repeat. Conceptually, the model can learn anything. Meaning, the intelligence and ability of the system depends on the quality of the schema, and the volume and variety of data you can teach it. In practice, effective training data gives you a key edge when all else—budget, resources, etc.—are equal.
Second, training data work is upstream, before data science work. This means data science is dependent on training data. Errors in training data flow down to data science. Or to use the cliché—garbage in, garbage out. Figure 1-3 walks through what this data flow looks like in practice.
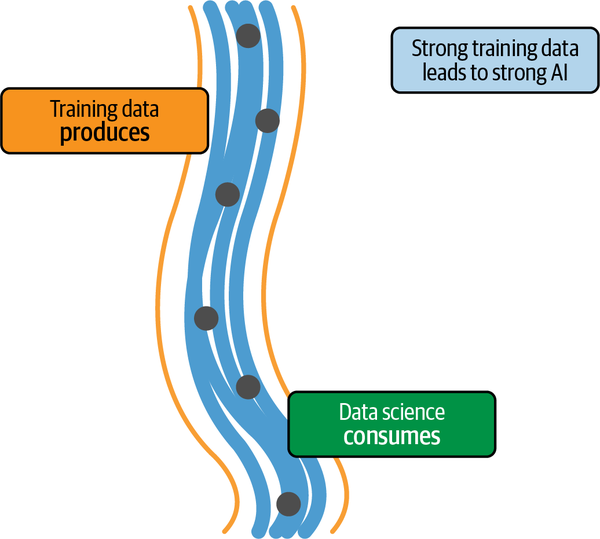
Figure 1-3. Conceptual positions of training data and data science
Third, the art of training data represents a shift in thinking about how to build AI systems. Instead of over-focusing on improving mathematical algorithms, in parallel with them, we continue to optimize the training data to better match our needs. This is the heart of the AI transformation taking place, and the core of modern automation. For the first time, knowledge work is now being automated.
ML Applications Are Becoming Mainstream
In 2005, a university team used a training data–based3 approach to engineer a vehicle, Stanley, that could drive autonomously on an off-road 175-mile-long desert course, winning the Defense Advanced Research Projects Agency (DARPA) grand challenge. About 15 years later, in October 2020, an automotive company released a controversial Full Self-Driving (FSD) technology in public, ushering in a new era of consumer awareness. In 2021, data labeling concerns started getting mentioned on earnings calls. In other words, the mainstream is starting to get exposed to training data.
This commercialization goes beyond headlines of AI research results. In the last few years, we have seen the demands placed on technology increase dramatically. We expect to be able to speak to software and be understood, to automatically get good recommendations and personalized content. Big tech companies, startups, and businesses alike are increasingly turning to AI to address this explosion in use case combinations.
AI knowledge, tooling, and best practices rapidly expand. What used to be the exclusive domain of a few is now becoming common knowledge, and pre-built API calls. We are at the transition phase, going from R&D demos to the early stages of real-world industry use cases.
Expectations around automation are being redefined. Cruise control, to a new car buyer, has gone from just “maintain constant speed” to include “lane keeping, distance pacing, and more.” These are not future considerations. These are current consumer and business expectations. They indicate clear and present needs to have an AI strategy and to have ML and training data competency in your company.
The Foundation of Successful AI
Machine learning is about learning from data. Historically, this meant creating datasets in the form of logs, or similar tabular data such as “Anthony viewed a video.”
These systems continue to have significant value. However, they have some limits. They won’t help us do things modern training data–powered AI can do, like build systems to understand a CT scan or other medical imaging, understand football tactics, or in the future, operate a vehicle.
The idea behind this new type of AI is a human expressly saying, “Here’s an example of what a player passing a ball looks like,” “Here’s what a tumor looks like,” or “This section of the apple is rotten.”
This form of expression is similar to how in a classroom a teacher explains concepts to students: by words and examples. Teachers help fill the gap between the textbooks, and students build a multidimensional understanding over time. In training data, the annotator acts as the teacher, filling the gap between the schema and the raw data.
Training Data Is Here to Stay
As mentioned earlier, use cases for modern AI/ML data are transitioning from R&D to industry. We are at the very start of a long curve in that business cycle. Naturally, the specifics shift quickly. However, the conceptual ideas around thinking of day-to-day work as annotation, encouraging people to strive more and more for unique work, and oversight of increasingly capable ML programs, are all here to stay.
On the research side, algorithms and ideas on how to use training data both keep improving. For example, the trend is for certain types of models to require less and less data to be effective. The fewer samples a model needs to learn, the more weight is put on creating training data with greater breadth and depth. And on the other side of the coin, many industry use cases often require even greater amounts of data to reach business goals. In that business context, the need for more and more people to be involved in training data puts further pressure on tooling.
In other words, the expansion directions of research and industry put more and more importance on training data over time.
Training Data Controls the ML Program
The question in any system is control. Where is the control? In normal computer code, this is human-written logic in the form of loops, if statements, etc. This logic defines the system.
In classic machine learning, the first steps include defining features of interest and a dataset. Then an algorithm generates a model. While it may appear that the algorithm is in control, the real control is exercised by choosing the features and data, which determine the algorithm’s degrees of freedom.
In a deep learning system, the algorithm does its own feature selection. The algorithm attempts to determine (learn) what features are relevant to a given goal. That goal is defined by training data. In fact, training data is the primary definition of the goal.
Here’s how it works. An internal part of the algorithm, called a loss function, describes a key part of how the algorithm can learn a good representation of this goal. The algorithm uses the loss function to determine how close it is to the goal defined in the training data.
More technically, the loss is the error we want to minimize during model training. For a loss function to have human meaning, there must be some externally defined goal, such as a business objective that makes sense relative to the loss function. That business objective may be defined in part through the training data.
In a sense, this is a “goal within a goal”; the training data’s goal is to best relate to the business objective, and the loss function’s goal is to relate the model to the training data. So to recap, the loss function’s goal is to optimize the loss, but it can only do that by having some precursor reference point, which is defined by the training data. Therefore, to conceptually skip the middleman of the loss function, the training data is the “ground truth” for the correctness of the model’s relationship to the human-defined goal. Or to put it simply: the human objective defines the training data, which then defines the model.
New Types of Users
In traditional software development there is a degree of dependency between the end user and the engineer. The end user cannot truly say whether the program is “correct,” and neither can the engineer.
It’s hard for an end user to say what they want until a prototype of it has been built. Therefore, both the end user and engineer are dependent on each other. This is called a circular dependency. The ability to improve software comes from the interplay between both, to be able to iterate together.
With training data, the humans control the meaning of the system when doing the literal supervision. Data scientists control it when working on schemas, for example when choosing abstractions such as label templates.
For example, if I, as an annotator, were to label a tumor as cancerous, when in fact it’s benign, I would be controlling the output of the system in a detrimental way. In this context, it’s worth understanding that there is no validation possible to ever 100% eliminate this control. Engineering cannot, both because of the volume of data and because of lack of subject matter expertise, control the data system.
There used to be this assumption that data scientists knew what “correct” was. The theory was that they could define some examples of “correct,” and then as long as the human supervisors generally stuck to that guide, they knew what correct was. Examples of all sorts of complications arise immediately: How can an English-speaking data scientist know if a translation to French is correct? How can a data scientist know if a doctor’s medical opinion on an X-ray image is correct? The short answer is—they can’t. As the role of AI systems grows, subject matter experts increasingly need to exercise control on the system in ways that supersede data science.4
Let’s consider why this is different from the traditional “garbage in, garbage out” concept. In a traditional program, an engineer can guarantee that the code is “correct” with, e.g., a unit test. This doesn’t mean that it gives the output desired by the end user, just that the code does what the engineer feels it’s supposed to do. So to reframe this, the promise is “gold in, gold out” as long as the user puts gold in, they will get gold out.
Writing an AI unit test is difficult in the context of training data. In part, this is because the controls available to data science, such as a validation set, are still based on the control (doing annotations) executed by individual AI supervisors.
Further, AI supervisors may be bound by the abstractions engineering defines for them to use. However, if they are able to define the schema themselves, they are more deeply woven into the fabric of the system itself, thus further blurring of the lines between “content” and “system.”
This is distinctly different from classic systems. For example, on a social media platform, your content may be the value, but it’s still clear what is the literal system (the box you type in, the results you see, etc.) and the content you post (text, pictures, etc.).
Now that we’re thinking in terms of form and content, how does control fit back in? Examples of control include:
-
Abstractions, like the schema, define one level of control.
-
Annotation, literally looking at samples, defines another level of control.
While data science may control the algorithms, the controls of training data often act in an “oversight” capacity, above the algorithm.
Training Data in the Wild
So far, we’ve covered a lot of concepts and theory, but training data in practice can be a complex and challenging thing to do well.
What Makes Training Data Difficult?
The apparent simplicity of data annotation hides the vast complexity, novel considerations, new concepts and new forms of art involved. It may appear that a human selects an appropriate label, the data goes through a machine process, and voilà, we have a solution, right? Well, not quite. Here are a few common elements that can prove difficult.
Subject matter experts (SMEs) are working with technical folks in new ways and vice versa. These new social interactions introduce new “people” challenges. Experts have individual experiences, beliefs, inherent bias, and prior experiences. Also, experts from multiple fields may have to work more closely than usual together. Users are operating novel annotation interfaces with few common expectations on what standard design looks like.
Additional challenges include:
-
The problem itself may be difficult to articulate, with unclear answers or poorly defined solutions.
-
Even if the knowledge is well formed in a person’s head, and the person is familiar with the annotation interface, inputting that knowledge accurately can be tedious and time consuming.
-
Often there is a voluminous amount of data labeling work with multiple datasets to manage and technical challenges around storing, accessing, and querying the new forms of data.
-
Given that this is a new discipline, there is a lack of organizational experience and operational excellence that can only come with time.
-
Organizations with a strong classical ML culture may have trouble adapting to this fundamentally different, yet operationally critical, area. This blindspot of thinking they have already understood and implemented ML, when in fact it’s a totally different form.
-
As it is a new art form, general ideas and concepts are not well known. There is a lack of awareness, access, or familiarity to the right training data tools.
-
Schemas may be complex, with thousands of elements, including nested conditional structures. And media formats impose challenges like series, relationships, and 3D navigation.
-
Most automation tools introduce new challenges and difficulties.
While the challenges are myriad and at times difficult, we’ll tackle each of them in this book to provide a roadmap you and your organization can implement to improve training data.
The Art of Supervising Machines
Up to this point, we’ve covered some of the basics and a few of the challenges around training data. Let’s shift gears away from the science for a moment and focus on the art. The apparent simplicity of annotation hides the vast volume of work involved. Annotation is to training data what typing is to writing. Simply pressing keys on a keyboard doesn’t provide value if you don’t have the human element informing the action and accurately carrying out the task.
Training data is a new paradigm upon which a growing list of mindsets, theories, research, and standards are emerging. It involves technical representations, people decisions, processes, tooling, system design, and a variety of new concepts specific to it.
One thing that makes training data so special is that it is capturing the user’s knowledge, intent, ideas, and concepts without specifying “how” they arrived at them. For example, if I label a “bird,” I am not telling the computer what a bird is, the history of birds, etc.—only that it is a bird. This idea of conveying a high level of intent is different from most classical programming perspectives. Throughout this book, I will come back to this idea of thinking of training data as a new form of coding.
A New Thing for Data Science
While an ML model may consume a specific training dataset, this book will unpack the myriad of concepts around the abstract concepts of training data. More generally, training data is not data science. They have different goals. Training data produces structured data; data science consumes it. Training data is mapping human knowledge from the real world into the computer. Data science is mapping that data back to the real world. They are the two different sides of the coin.
Similar to how a model is consumed by an application, training data must be consumed by data science to be useful. The fact that it’s used in this way should not detract from its differences. Training data still requires mappings of concepts to a form usable by data science. The point is having clearly defined abstractions between them, instead of ad hoc guessing on terms.
It seems more reasonable to think of training data as an art practiced by all the other professions, that is practiced by subject matter experts from all walks of life, than to think of data science as the all-encompassing starting point. Given how many subject matter experts and non-technical people are involved, the rather preposterous alternative would seem to assume that data science towers over all! It’s perfectly natural that, to data science, training data will be synonymous with labeled data and a subset of overall concerns; but to many others, training data is its own domain.
While attempting to call anything a new domain or art form is automatically presumptuous, I take solace in that I am simply labeling something people are already doing. In fact, things make much more sense when we treat it as its own art and stop shoehorning it into other existing given categories. I cover this in more detail in Chapter 7.
Because training data as a named domain is new, the language and definitions remain fluid. The following terms are all closely related:
-
Training data
-
Data labeling
-
Human computer supervision
-
Annotation
-
Data program
Depending on the context, those terms can map to various definitions:
-
The overall art of training data
-
The act of annotating, such as drawing geometries and answering schema questions
-
The definition of what we want to achieve in a machine learning system, the ideal state desired
-
The control of the ML system, including correction of existing systems
-
A system that relies on human-controlled data
For example, I can refer to annotation as a specific subcomponent of the overall concept of training data. I can also say “to work with training data,” to mean the act of annotating. As a novel developing area, people may say data labeling and mean just the literal basics of annotation, while others mean the overall concept of training data.
The short story here is it’s not worth getting too hung up on any of those terms, and the context it’s used in is usually needed to understand the meaning.
ML Program Ecosystem
Training data interacts with a growing ecosystem of adjacent programs and concepts. It is common to send data from a training data program to an ML modeling program, or to install an ML program on a training data platform. Production data, such as predictions, is often sent to a training data program for validation, review, and further control. The linkage between these various programs continues to expand. Later in this book we cover some of the technical specifics of ingesting and streaming data.
Raw data media types
Data comes in many media types. Popular media types include images, videos, text, PDF/document, HTML, audio, time series, 3D/DICOM, geospatial, sensor fusion, and multimodal. While popular media types are often the best supported in practice, in theory any media type can be used. Forms of annotation include attributes (detailed options), geometries, relationships, and more. We’ll cover all of this in great detail as the book progresses, but it’s important to note that if a media type exists, someone is likely attempting to extract data from it.
Data-Centric Machine Learning
Subject matter experts and data entry folks may end up spending four to eight hours a day, every day, on training data tasks like annotation. It’s a time-intensive task, and it may become their primary work. In some cases, 99% of the overall team’s time is spent on training data and 1% on the modeling process, for example by using an AutoML-type solution or having a large team of SMEs.5
Data-centric AI means focusing on training data as its own important thing—creating new data, new schemas, new raw data capturing techniques, and new annotations by subject matter experts. It means developing programs with training data at the heart and deeply integrating training data into aspects of your program. There was mobile-first, and now there’s data-first.
In the data-centric mindset you can:
-
Use or add data collection points, such as new sensors, new cameras, new ways to capture documents, etc.
-
Add new human knowledge in the form of, for example, new annotations, e.g., from subject matter experts.
The rationales behind a data-centric approach are:
-
The majority of the work is in the training data, and the data science aspect is out of our control.
-
There are more degrees of freedom with training data and modeling than with algorithm improvements alone.
When I combine this idea of data-centric AI with the idea of seeing the breadth and depth of training data as its own art, I start to see the vast fields of opportunities. What will you build with training data?
Failures
It’s common for any system to have a variety of bugs and still generally “work.” Data programs are similar. For example, some classes of failures are expected, and others are not. Let’s dive in.
Data programs work when their associated sets of assumptions remain true, such as assumptions around the schema and raw data. These assumptions are often most obvious at creation, but can be changed or modified as part of a data maintenance cycle.
To dive into a visual example, imagine a parking lot detection system. The system may have very different views, as shown in Figure 1-4. If we create a training data set based on a top-down view (left) and then attempt to use a car-level view (right) we will likely get an “unexpected” class of failure.
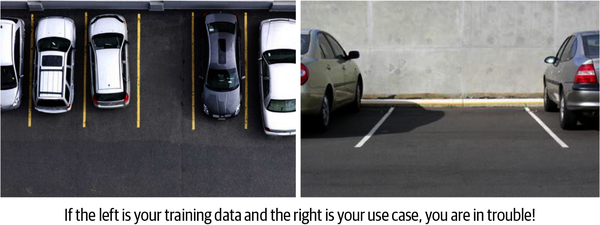
Figure 1-4. Comparison of major differences in raw data that would likely lead to an unexpected failure
Why was there a failure? A machine learning system trained only on images from a top-down view, as in the left image, has a hard time running in an environment where the images are from a front view, as shown in the right image. In other words, the system would not understand the concept of a car and parking lot from a front view if it has never seen such an image during training.
While this may seem obvious, a very similar issue caused a real-world failure in a US Air Force system, leading them to think their system was materially better than it actually was.
How can we prevent failures like this? Well, for this specific one, it’s a clear example of why it’s important that the data we use to train a system closely matches production data. What about failures that aren’t listed specifically in a book?
The first step is being aware of training data best practices. Earlier, talking about human roles, I mentioned how communication with annotators and subject matter experts is important. Annotators need to be able to flag issues, especially those regarding alignment of schemas and raw data. Annotators are uniquely positioned to surface issues outside the scope of specified instructions and schemas, e.g., when that “common sense” that something isn’t right kicks in.
Admins need to be aware of the concept of creating a novel, well-named schema. The raw data should always be relevant to the schema, and maintenance of the data is a requirement.
Failure modes are surfaced during development through discussions around schema, expected data usage, and discussions with annotators.
History of Development Affects Training Data Too
When we think of classic software programs, their historical development biases them toward certain states of operation. An application designed for a smartphone has a certain context, and may be better or worse than a desktop application at certain things. A spreadsheet app may be better suited for desktop use; a money-sending system disallows random edits. Once a program like that has been written, it becomes hard to change core aspects, or “unbias it.” The money-sending app has many assumptions built around an end user not being able to “undo” a transaction.
The history of a given model’s development, accidental or intentional, also affects training data. Imagine a crop inspection application mostly designed around diseases that affect potato crops. There were assumptions made regarding everything from the raw data format (e.g., that the media is captured at certain heights), to the types of diseases, to the volume of samples. It’s unlikely it will work well for other types of crops. The original schema may make assumptions that become obsolete over time. The system’s history will affect the ability to change the system going forward.
What Training Data Is Not
Training data is not an ML algorithm. It is not tied to a specific machine learning approach.
Rather, it’s the definition of what we want to achieve. The fundamental challenge is effectively identifying and mapping the desired human meaning into a machine-readable form.
The effectiveness of training data depends primarily on how well it relates to the human-defined meaning assigned to it and how reasonably it represents real model usage. Practically, choices around training data have a huge impact on the ability to train a model effectively.
Generative AI
Generative AI (GenAI) concepts, like generative pre-trained transformers (GPTs) and large language models (LLMs), became very popular in early 2023. Here, I will briefly touch on how these concepts relate to training data.
At the time of writing, this area is moving very rapidly. Major commercial players are being extremely restrictive in what they share publicly, so there’s a lot of speculation and hype but little consensus. Therefore, most likely, some of this Generative AI section will be out of date by the time you are reading it.
We can begin with the concept of unsupervised learning. The broadly stated goal of unsupervised learning in the GenAI context is to work without newly defined human-made labels. However, LLMs’ “pre-training” is based off of human source material. So you still need data, and usually human-generated data, to get something that’s meaningful to humans. The difference is when “pre-training” a generative AI, the data doesn’t initially need labels to create an output, leading to GenAI being affectionately referred to as the unsupervised “monster.” This “monster,” as shown in Figure 1-5 must still be tamed with human supervision.
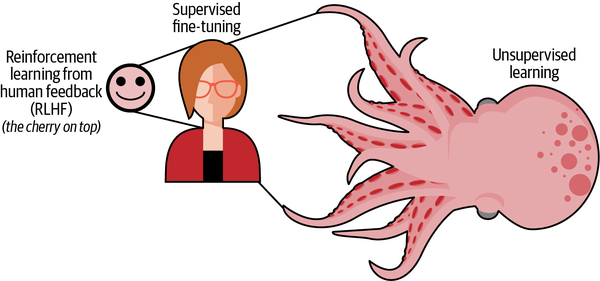
Figure 1-5. Relationship of unsupervised learning to supervised fine-tuning and human alignment
Broadly speaking, these are the major ways that GenAI interacts with human supervision:
- Human alignment
- Human supervision is crucial to build and improve GenAI models.
- Efficiency improvements
- GenAI models can be used to improve tedious supervision tasks (like image segmentation).
- Working in tandem with supervised AI
- GenAI models can be used to interpret, combine, interface with, and use supervised outputs.
- General awareness of AI
- AI is being mentioned daily in major news outlets and on earning calls by companies. General excitement around AI has increased dramatically.
I’ll expand on the human alignment concept in the next subsection.
You can also use GenAI to help improve supervised training data efficiency. Some “low-hanging” fruit, in terms of generic object segmentation, generic classification of broadly accepted categories, etc., is all possible (with some caveats) through current GenAI systems. I cover this more in Chapter 8 when I discuss automation.
Working in tandem with supervised AI is mostly out of scope of this book, beyond briefly stating that there is surprisingly little overlap. GenAI and supervised systems are both important building blocks.
Advances in GenAI have made AI front-page news again. As a result, organizations are rethinking their AI objectives and putting more energy into AI initiatives in general, not just GenAI. To ship a GenAI system, human alignment (in other words, training data) is needed. To ship a complete AI system, often GenAI + supervised AI is needed. Learning the skills in this book for working with training data will help you with both goals.
Human Alignment Is Human Supervision
Human supervision, the focus of this book, is often referred to as human alignment in the generative AI context. The vast majority of concepts discussed in this book also apply to human alignment, with some case-specific modifications.
The goal is less for the model to directly learn to repeat an exact representation, but rather to “direct” the unsupervised results. While exactly which human alignment “direction” methods are best is a subject of hot debate, specific examples of current popular approaches to human alignment include:
-
Direct supervision, such as question and answer pairs, ranking outputs (e.g., personal preference, best to worst), and flagging specifically iterated concerns such as “not safe for work.” This approach was key to GPT-4’s fame.
-
Indirect supervision, such as end users voting up/down, providing freeform feedback, etc. Usually, this input must go through some additional process before being presented to the model.
-
Defining a “constitutional” set of instructions that lay out specific human supervision (human alignment) principles for the GenAI system to follow.
-
Prompt engineering, meaning defining “code-like” prompts, or coding in natural language.
-
Integration with other systems to check the validity of results.
There is little consensus on the best approaches, or how to measure results. I would like to point out that many of these approaches have been focused on text, limited multimodal (but still text) output, and media generation. While this may seem extensive, it’s a relatively limited subsection of the more general concept of humans attaching repeatable meaning to arbitrary real-world concepts.
In addition to the lack of consensus, there is also conflicting research in this space. For example, two common ends of the spectrum are that some claim to observe emergent behavior, and others assert that the benchmarks were cherry-picked and that it’s a false result (e.g., that the test set is conflated with the training data). While it seems clear that human supervision has something to do with it, exactly what level, and how much, and what technique is an open question in the GenAI case. In fact, some results show that small human-aligned models can work as well or better than large models.
While you may notice some differences in terminology, many of the principles in this book apply as well to GenAI alignment as to training data. Specifically, all forms of direct supervision are training data supervision. A few notes before wrapping up the GenAI topic: I don’t specifically cover prompt engineering in this book, nor other GenAI-specific concepts. However, if you are looking to build a GenAI system, you will still need data, and high-quality supervision will remain a critical part of GenAI systems for the foreseeable future.
Summary
This chapter has introduced high-level ideas around training data for machine learning. Let’s recap why training data is important:
-
Consumers and businesses show increasing expectations around having ML built in, both for existing and new systems, increasing the importance of training data.
-
It serves as the foundation of developing and maintaining modern ML programs.
-
Training data is an art and a new paradigm. It’s a set of ideas around new, data-driven programs, and is controlled by humans. It’s separate from classic ML, and comprises new philosophies, concepts, and implementations.
-
It forms the foundation of new AI/ML products, maintaining revenue from existing lines of business, by replacing or improving costs through AI/ML upgrades, and is a fertile ground for R&D.
-
As a technologist or as a subject matter expert, it’s now an important skill set to have.
The art of training data is distinct from data science. Its focus is on the control of the system, with the goal that the system itself will learn. Training data is not an algorithm or a single dataset. It’s a paradigm that spans professional roles, from subject matter experts, to data scientists, to engineers and more. It’s a way to think about systems that opens up new use cases and opportunities.
Before reading on, I encourage you to review these key high-level concepts from this chapter:
-
Key areas of concern include schemas, raw data, quality, integrations, and the human role.
-
Classic training data is about discovery, while modern training data is a creative art; the means to “copy” knowledge.
-
Deep learning algorithms generate models based on training data. Training data defines the goal, and the algorithm defines how to work toward this goal.
-
Training data that is validated only “in a lab” will likely fail in the field. This can be avoided by primarily using field data as the starting point, by aligning the system design, and by expecting to rapidly update models.
-
Training data is like code.
In the next chapter we will cover getting set up with your training data system and we’ll learn about tools.
1 In most cases, that existing data is thought of as a “sample,” even if it was created by a human at some point prior.
2 Without further deductions outside our scope of concern.
3 From “Stanley_(vehicle)”, Wikipedia, accessed on September 8, 2023: “Stanley was characterized by a machine learning based approach to obstacle detection. To correct a common error made by Stanley early in development, the Stanford Racing Team created a log of ‘human reactions and decisions’ and fed the data into a learning algorithm tied to the vehicle’s controls; this action served to greatly reduce Stanley’s errors. The computer log of humans driving also made Stanley more accurate in detecting shadows, a problem that had caused many of the vehicle failures in the 2004 DARPA Grand Challenge.”
4 There are statistical methods to coordinate experts’ opinions, but these are always “additional”; there still has to be an existing opinion.
5 I’m oversimplifying here. In more detail, the key difference is that while a data science AutoML training product and hosting may be complex itself, there are simply fewer people working on it.
6 Read Will Douglas Heaven’s article, “Google’s Medical AI Was Super Accurate in a Lab. Real Life Was a Different Story”, MIT Technology Review, April 27, 2020.
Get Training Data for Machine Learning now with the O’Reilly learning platform.
O’Reilly members experience books, live events, courses curated by job role, and more from O’Reilly and nearly 200 top publishers.