Rethinking informed consent
Consent is the first step toward the ethical use of data, but it's not the last.
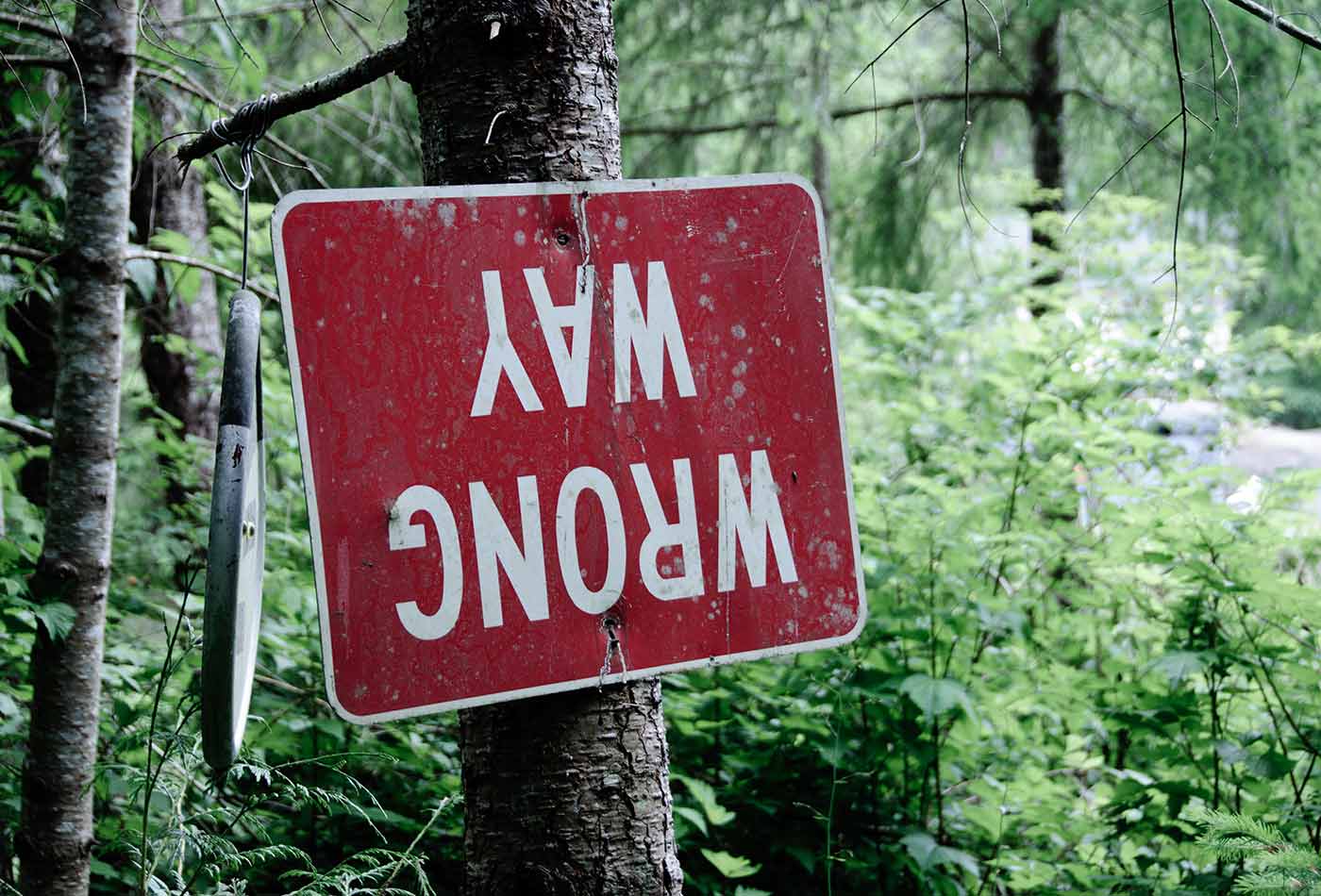
Informed consent is part of the bedrock of data ethics. DJ Patil, Hilary Mason, and I have written about it, as have many others. It’s rightfully part of every code of data ethics I’ve seen. But I have to admit misgivings—not so much about the need for consent, but about what it means. Obtaining consent to collect and use data isn’t the end of the process; at best, it’s the beginning, and perhaps not a very good one.
Helen Nissenbaum, in an interview with Scott Berinato, articulates some of the problems. It’s easy to talk about informed consent, but what do we mean by “informed”? Almost everyone who reads this article has consented to some kind of medical procedure; did any of us have a real understanding of what the procedure was and what the risks were? We rely on the prestige or status of the doctor, but unless we’re medical professionals, or have done significant online research, we have, at best, a vague notion of what’s going to happen and what the risks are. In medicine, for the most part, things come out all right. The problems with consent to data collection are much deeper.
The problem starts with the origin of the consent criterion. It comes from medicine and the social sciences, in which consenting to data collection and to being a research subject has a substantial history. It arose out of experiments with mind-boggling ethical problems (for example, the Tuskeegee syphilis experiment), and it still isn’t always observed (paternalism is still a thing). “Consent” in medicine is limited: whether or not you understand what you’re consenting to, you are consenting to a single procedure (plus emergency measures if things go badly wrong). The doctor can’t come back and do a second operation without further consent. And likewise, “consent” in the social sciences is limited to a single study: you become a single point in an array of data that ceases to exist when the study is complete.
That may have been true years ago, but those limitations on how consent is used seem very shaky, as Nissenbaum argues. Consent is fundamentally an assurance about context: consenting to a medical procedure means the doctors do their stuff, and that’s it. The outcome might not be what you want, but you’ve agreed to take the risk. But what about the insurance companies? They get the data, and they can repackage and exchange it. What happens when, a few years down the road, you’re denied coverage because of a “pre-existing condition”? That data has moved beyond the bounds of an operating room. What happens when data from an online survey or social media profile is shared with another organization and combined and re-combined with other data? When it is used in other contexts, can it be de-anonymized and used to harm the participants? That single point in an array of data has now become a constellation of points feeding many experiments, not all of which are benign.
I’m haunted by the question, “what are users consenting to?” Technologists rarely think through the consequences of their work carefully enough; but even if they did, there will always be consequences that can’t be foreseen or understood, particularly when data from different sources is combined. So, consenting to data collection, whether it’s clicking on the ever-present checkbox about cookies or agreeing to Facebook’s license agreement, is significantly different from agreeing to surgery. We really don’t know how that data is used, or might be used, or could be used in the future. To use Nissenbaum’s language, we don’t know where data will flow, nor can we predict the contexts in which it will be used.
Consent frequently isn’t optional, but compelled. Writing about the #DeleteFacebook movement, Jillian York argues that for many, deleting Facebook is not an option: “for people with marginalized identities, chronic illnesses, or families spread across the world, walking away [from Facebook] means leaving behind a potentially vital safety net of support.” She continues by writing that small businesses, media outlets, artists, and activists rely on it to reach audiences. While no one is compelled to sign up, or to remain a user, for many “deleting facebook” means becoming a non-entity. If Facebook is your only way to communicate with friends, relatives, and support communities, refusing “consent” may not be an option; consent is effectively compelled. The ability to withdraw consent from Facebook is a sign of privilege. If you lack privilege, an untrustworthy tool may be better than no tool at all.
One alternative to consent is the idea that you own the data and should be compensated for its use. Eric Posner, Glen Weyl, and others have made this argument, which essentially substitutes a market economy for consent: if you pay me enough, I’ll let you use my data. However, markets don’t solve many problems. In “It’s time for a bill of data rights,” Martin Tisne argues that data ownership is inadequate. When everything you do creates data, it’s no more meaningful to own your “digital shadow” than your physical one. How do you “own” your demographic profile? Do you even “own” your medical record? Tisne writes: “A person doesn’t ‘own’ the fact that she has diabetes—but she can have the right not to be discriminated against because of it… But absent government regulation to prevent health insurance companies from using data about preexisting conditions, individual consumers lack the ability to withhold consent. … Consent, to put it bluntly, does not work.” And it doesn’t work whether or not consent is mediated by a market. At best, the market may give some incremental income, but at worst, it gives users incentives to act against their best interest.
It’s also easy to forget that in many situations, users are compensated for their data: we’re compensated by the services that Facebook, Twitter, Google, and Amazon provide. And that compensation is significant; how many of us could do our jobs without Google? The economic value of those services to me is large, and the value of my data is actually quite limited. To Google, the dozens of Google searches I do in a day are worth a few cents at most. Google’s market valuation doesn’t derive from the value of my data or yours in isolation, but the added value that comes from aggregating data across billions of searches and other sources. Who owns that added value? Not me. An economic model for consent (I consent to let you use my data if you pay me) misses the point: data’s value doesn’t live with the individual.
It would be tragic to abandon consent, though I agree with Nissenbaum that we urgently need to get beyond “incremental improvement to consent mechanisms.” It is time to recognize that consent has serious limitations, due partly to its academic and historical origins. It’s important to gain consent for participation in an experiment; otherwise, the subject isn’t a participant but a victim. However, while understanding the consequences of any action has never been easy, the consent criterion arose when consequences were far more limited and data didn’t spread at the speed of light.
So, the question is: how do we get beyond consent? What kinds of controls can we place on the collection and use of data that align better with the problems we’re facing? Tisne suggests a “data bill of rights”: a set of general legal principles about how data can be used. The GDPR is a step in this direction; the Montreal Declaration for the Responsible Development of Artificial Intelligence could be reformulated as a “bill of data rights.” But a data bill of rights assumes a new legal infrastructure, and by nature such infrastructures place the burden of redress on the user. Would one bring a legal action against Facebook or Google for violation of one’s data rights? Europe’s enforcement of GDPR will provide an important test case, particularly since this case is essentially about data flows and contexts. It isn’t clear that our current legal institutions can keep pace with the many flows and contexts in which data travels.
Nissenbaum starts from the knowledge that data moves, and that the important questions aren’t around how our data is used, but where our data travels. This shift in perspective is important precisely because data sets become more powerful when they’re combined; because it isn’t possible to anticipate all the ways data might be used; and because once data has started flowing, it’s very hard to stop it. But we have to admit we don’t yet know how to ask for consent about data flows or how to prove they are under control. Which data flows should be allowed? Which shouldn’t? We want to enable medical research on large aggregated data sets without jeopardizing the insurance coverage of the people whose data are in those sets. Data would need to carry metadata with it that describes where it could be transferred and how it could be used once it’s transferred; it makes no sense to talk about controlling data flows if that control can’t be automated.
As Ben Lorica and I have argued, the only way forward is through more automation, not less; issues of scale won’t let us have it any other way. In a conversation, Andrew Zaldivar told me of his work with Margaret Mitchell, Timnit Gebru, and others, on model cards that describe the behavior of a machine learning model, and of Timnit Gebru’s work on Datasheets for Datasets, which specify how a data set was collected, how it is intended to be used, and other information. Model cards and data set datasheets are a step toward the kind of metadata we’d need to automate control over data flows, to build automated tools that manage where data can and can’t travel, to protect public goods as well as personal privacy. In the past year, we’ve seen how easy it is to be overly optimistic about tool building, but we are all already using data at the scale of Google and Facebook. There will need to be human systems that override automatic control over data flows, but automation is an essential ingredient.
Consent is the first step along the path toward ethical use of data, but not the last one. What is the next step?