Chapter 1. Introduction
In this first chapter, we will introduce machine learning pipelines and outline all the steps that go into building them. We’ll explain what needs to happen to move your machine learning model from an experiment to a robust production system. We’ll also introduce our example project that we will use throughout the rest of the book to demonstrate the principles we describe.
Why Machine Learning Pipelines?
The key benefit of machine learning pipelines lies in the automation of the model life cycle steps. When new training data becomes available, a workflow which includes data validation, preprocessing, model training, analysis, and deployment should be triggered. We have observed too many data science teams manually going through these steps, which is costly and also a source of errors. Let’s cover some details of the benefits of machine learning pipelines:
- Ability to focus on new models, not maintaining existing models
-
Automated machine learning pipelines will free up data scientists from maintaining existing models. We have observed too many data scientists spending their days on keeping previously developed models up to date. They run scripts manually to preprocess their training data, they write one-off deployment scripts, or they manually tune their models. Automated pipelines allow data scientists to develop new models, the fun part of their job. Ultimately, this will lead to higher job satisfaction and retention in a competitive job market.
- Prevention of bugs
-
Automated pipelines can prevent bugs. As we will see in later chapters, newly created models will be tied to a set of versioned data and preprocessing steps will be tied to the developed model. This means that if new data is collected, a new model will be generated. If the preprocessing steps are updated, the training data will become invalid and a new model will be generated. In manual machine learning workflows, a common source of bugs is a change in the preprocessing step after a model was trained. In this case, we would deploy a model with different processing instructions than what we trained the model with. These bugs might be really difficult to debug since an inference of the model is still possible, but simply incorrect. With automated workflows, these errors can be prevented.
- Useful paper trail
-
The experiment tracking and the model release management generate a paper trail of the model changes. The experiment will record changes to the model’s hyperparameters, the used datasets, and the resulting model metrics (e.g., loss or accuracy). The model release management will keep track of which model was ultimately selected and deployed. This paper trail is especially valuable if the data science team needs to re-create a model or track the model’s performance.
- Standardization
-
Standardized machine learning pipelines improve the experience of a data science team. Due to the standardized setups, data scientists can be onboarded quickly or move across teams and find the same development environments. This improves efficiency and reduces the time spent getting set up on a new project. The time investment of setting up machine learning pipelines can also lead to an improved retention rate.
- The business case for pipelines
-
The implementation of automated machine learning pipelines will lead to three key impacts for a data science team:
-
More development time for novel models
-
Simpler processes to update existing models
-
Less time spent to reproduce models
-
All these aspects will reduce the costs of data science projects. But furthermore, automated machine learning pipelines will:
-
Help detect potential biases in the datasets or in the trained models. Spotting biases can prevent harm to people who interact with the model. For example, Amazon’s machine learning–powered resume screener was found to be biased against women.
-
Create a paper trail (via experiment tracking and model release management) that will assist if questions arise around data protection laws, such as Europe’s General Data Protection Regulation (GDPR).
-
Free up development time for data scientists and increase their job satisfaction.
When to Think About Machine Learning Pipelines
Machine learning pipelines provide a variety of advantages, but not every data science project needs a pipeline. Sometimes data scientists simply want to experiment with a new model, investigate a new model architecture, or reproduce a recent publication. Pipelines wouldn’t be useful in these cases. However, as soon as a model has users (e.g., it is being used in an app), it will require continuous updates and fine-tuning. In these situations, we are back in the scenarios we discussed earlier about continuously updating models and reducing the burden of these tasks for data scientists.
Pipelines also become more important as a machine learning project grows. If the dataset or resource requirements are large, the approaches we discuss allow for easy infrastructure scaling. If repeatability is important, this is provided through the automation and the audit trail of machine learning pipelines.
Overview of the Steps in a Machine Learning Pipeline
A machine learning pipeline starts with the ingestion of new training data and ends with receiving some kind of feedback on how your newly trained model is performing. This feedback can be a production performance metric or feedback from users of your product. The pipeline includes a variety of steps, including data preprocessing, model training, and model analysis, as well as the deployment of the model. You can imagine that going through these steps manually is cumbersome and very error-prone. In the course of this book, we will introduce tools and solutions to automate your machine learning pipeline.
As you can see in Figure 1-1, the pipeline is actually a recurring cycle. Data can be continuously collected and, therefore, machine learning models can be updated. More data generally means improved models. And because of this constant influx of data, automation is key. In real-world applications, you want to retrain your models frequently. If you don’t, in many cases accuracy will decrease because the training data is different from the new data that the model is making predictions on. If retraining is a manual process, where it is necessary to manually validate the new training data or analyze the updated models, a data scientist or machine learning engineer would have no time to develop new models for entirely different business problems.
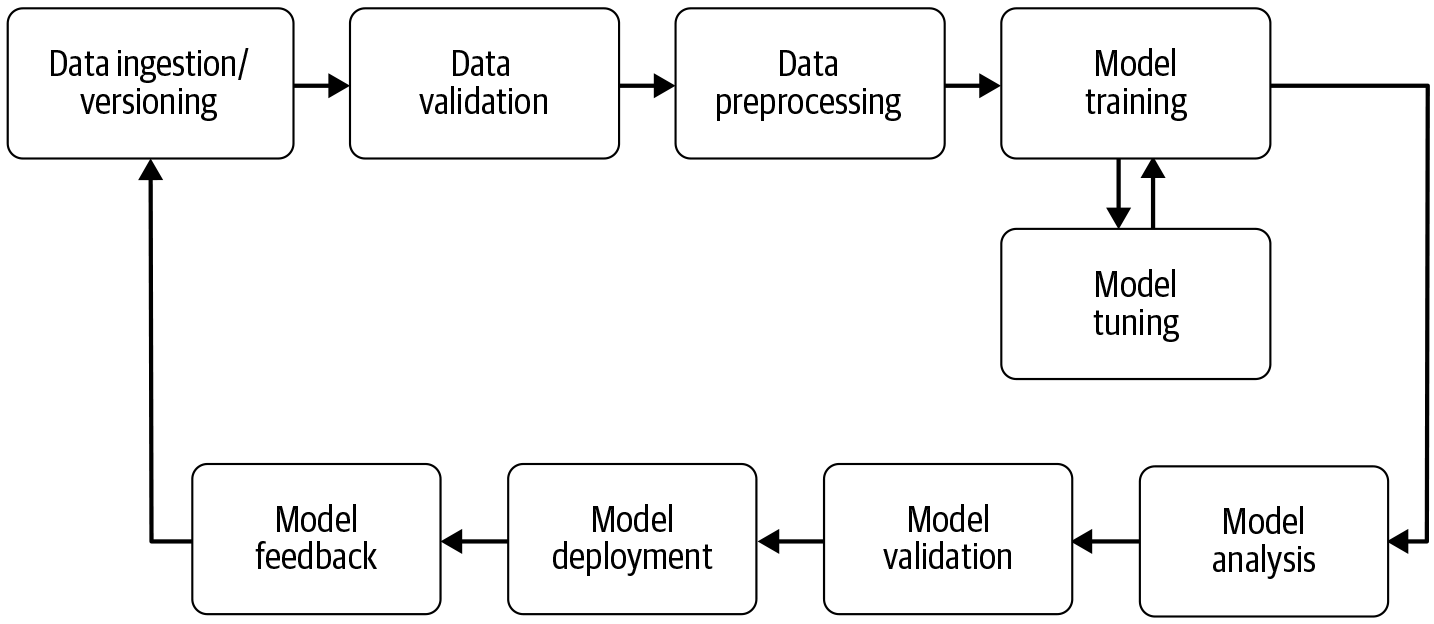
Figure 1-1. Model life cycle
A machine learning pipeline commonly includes the steps in the following sections.
Data Ingestion and Data Versioning
Data ingestion, as we describe in Chapter 3, is the beginning of every machine learning pipeline. In this pipeline step, we process the data into a format that the following components can digest. The data ingestion step does not perform any feature engineering (this happens after the data validation step). It is also a good moment to version the incoming data to connect a data snapshot with the trained model at the end of the pipeline.
Data Validation
Before training a new model version, we need to validate the new data. Data validation (Chapter 4) focuses on checking that the statistics of the new data are as expected (e.g., the range, number of categories, and distribution of categories). It also alerts the data scientist if any anomalies are detected. For example, if you are training a binary classification model, your training data could contain 50% of Class X samples and 50% of Class Y samples. Data validation tools provide alerts if the split between these classes changes, where perhaps the newly collected data is split 70/30 between the two classes. If a model is being trained with such an imbalanced training set and the data scientist hasn’t adjusted the model’s loss function, or over/under sampled category X or Y, the model predictions could be biased toward the dominant category.
Common data validation tools will also allow you to compare different datasets. If you have a dataset with a dominant label and you split the dataset into a training and validation set, you need to make sure that the label split is roughly the same between the two datasets. Data validation tools will allow you to compare datasets and highlight anomalies.
If the validation highlights anything out of the ordinary, the pipeline can be stopped here and the data scientist can be alerted. If a shift in the data is detected, the data scientist or the machine learning engineer can either change the sampling of the individual classes (e.g., only pick the same number of examples from each class), or change the model’s loss function, kick off a new model build pipeline, and restart the life cycle.
Data Preprocessing
It is highly likely that you cannot use your freshly collected data and train your machine learning model directly. In almost all cases, you will need to preprocess the data to use it for your training runs. Labels often need to be converted to one or multi-hot vectors.1 The same applies to the model inputs. If you train a model from text data, you want to convert the characters of the text to indices or the text tokens to word vectors. Since preprocessing is only required prior to model training and not with every training epoch, it makes the most sense to run the preprocessing in its own life cycle step before training the model.
Data preprocessing tools can range from a simple Python script to elaborate graph models. While most data scientists focus on the processing capabilities of their preferred tools, it is also important that modifications of preprocessing steps can be linked to the processed data and vice versa. This means if someone modifies a processing step (e.g., allowing an additional label in a one-hot vector conversion), the previous training data should become invalid and force an update of the entire pipeline. We describe this pipeline step in Chapter 5.
Model Training and Tuning
The model training step (Chapter 6) is the core of the machine learning pipeline. In this step, we train a model to take inputs and predict an output with the lowest error possible. With larger models, and especially with large training sets, this step can quickly become difficult to manage. Since memory is generally a finite resource for our computations, the efficient distribution of the model training is crucial.
Model tuning has seen a great deal of attention lately because it can yield significant performance improvements and provide a competitive edge. Depending on your machine learning project, you may choose to tune your model before starting to think about machine learning pipelines or you may want to tune it as part of your pipeline. Because our pipelines are scalable, thanks to their underlying architecture, we can spin up a large number of models in parallel or in sequence. This lets us pick out the optimal model hyperparameters for our final production model.
Model Analysis
Generally, we would use accuracy or loss to determine the optimal set of model parameters. But once we have settled on the final version of the model, it’s extremely useful to carry out a more in-depth analysis of the model’s performance (described in Chapter 7). This may include calculating other metrics such as precision, recall, and AUC (area under the curve), or calculating performance on a larger dataset than the validation set used in training.
Another reason for an in-depth model analysis is to check that the model’s predictions are fair. It’s impossible to tell how the model will perform for different groups of users unless the dataset is sliced and the performance is calculated for each slice. We can also investigate the model’s dependence on features used in training and explore how the model’s predictions would change if we altered the features of a single training example.
Similar to the model-tuning step and the final selection of the best performing model, this workflow step requires a review by a data scientist. However, we will demonstrate how the entire analysis can be automated with only the final review done by a human. The automation will keep the analysis of the models consistent and comparable against other analyses.
Model Versioning
The purpose of the model versioning and validation step is to keep track of which model, set of hyperparameters, and datasets have been selected as the next version to be deployed.
Semantic versioning in software engineering requires you to increase the major version number when you make an incompatible change in your API or when you add major features. Otherwise, you increase the minor version number. Model release management has another degree of freedom: the dataset. There are situations in which you can achieve a significant difference of model performance without changing a single model parameter or architecture description by providing significantly more and/or better data for the training process. Does that performance increase warrant a major version upgrade?
While the answer to this question might be different for every data science team, it is essential to document all inputs into a new model version (hyperparameters, datasets, architecture) and track them as part of this release step.
Model Deployment
Once you have trained, tuned, and analyzed your model, it is ready for prime time. Unfortunately, too many models are deployed with one-off implementations, which makes updating models a brittle process.
Modern model servers allow you to deploy your models without writing web app code. Often, they provide multiple API interfaces like representational state transfer (REST) or remote procedure call (RPC) protocols and allow you to host multiple versions of the same model simultaneously. Hosting multiple versions at the same time will allow you to run A/B tests on your models and provide valuable feedback about your model improvements.
Model servers also allow you to update a model version without redeploying your application, which will reduce your application’s downtime and reduce the communication between the application development and the machine learning teams. We describe model deployment in Chapters 8 and 9.
Feedback Loops
The last step of the machine learning pipeline is often forgotten, but it is crucial to the success of data science projects. We need to close the loop. We can then measure the effectiveness and performance of the newly deployed model. During this step, we can capture valuable information about the performance of the model. In some situations, we can also capture new training data to increase our datasets and update our model. This may involve a human in the loop, or it may be automatic. We discuss feedback loops in Chapter 13.
Except for the two manual review steps (the model analysis step and the feedback step), we can automate the entire pipeline. Data scientists should be able to focus on the development of new models, not on updating and maintaining existing models.
Data Privacy
At the time of writing, data privacy considerations sit outside the standard machine learning pipeline. We expect this to change in the future as consumer concerns grow over the use of their data and new laws are brought in to restrict the usage of personal data. This will lead to privacy-preserving methods being integrated into tools for building machine learning pipelines.
We discuss several current options for increasing privacy in machine learning models in Chapter 14:
-
Differential privacy, where math guarantees that model predictions do not expose a user’s data
-
Federated learning, where the raw data does not leave a user’s device
-
Encrypted machine learning, where either the entire training process can run in the encrypted space or a model trained on raw data can be encrypted
Pipeline Orchestration
All the components of a machine learning pipeline described in the previous section need to be executed or, as we say, orchestrated, so that the components are being executed in the correct order. Inputs to a component must be computed before a component is executed. The orchestration of these steps is performed by tools such as Apache Beam, Apache Airflow (discussed in Chapter 11), or Kubeflow Pipelines for Kubernetes infrastructure (discussed in Chapter 12).
While data pipeline tools coordinate the machine learning pipeline steps, pipeline artifact stores like the TensorFlow ML MetadataStore capture the outputs of the individual processes. In Chapter 2, we will provide an overview of TFX’s MetadataStore and look behind the scenes of TFX and its pipeline components.
Why Pipeline Orchestration?
In 2015, a group of machine learning engineers at Google concluded that one of the reasons machine learning projects often fail is that most projects come with custom code to bridge the gap between machine learning pipeline steps.2 However, this custom code doesn’t transfer easily from one project to the next. The researchers summarized their findings in the paper “Hidden Technical Debt in Machine Learning Systems.”3 The authors argue in this paper that the glue code between the pipeline steps is often brittle and that custom scripts don’t scale beyond specific cases. Over time, tools like Apache Beam, Apache Airflow, or Kubeflow Pipelines have been developed. These tools can be used to manage the machine learning pipeline tasks; they allow a standardized orchestration and an abstraction of the glue code between tasks.
While it might seem cumbersome at first to learn a new tool (e.g., Beam or Airflow) or a new framework (e.g., Kubeflow) and set up an additional machine learning infrastructure (e.g., Kubernetes), the time investment will pay off very soon. By not adopting standardized machine learning pipelines, data science teams will face unique project setups, arbitrary log file locations, unique debugging steps, etc. The list of complications can be endless.
Directed Acyclic Graphs
Pipeline tools like Apache Beam, Apache Airflow, and Kubeflow Pipelines manage the flow of tasks through a graph representation of the task dependencies.
As the example graph in Figure 1-2 shows, the pipeline steps are directed. This means that a pipeline starts with Task A and ends with Task E, which guarantees that the path of execution is clearly defined by the tasks’ dependencies. Directed graphs avoid situations where some tasks start without all dependencies fully computed. Since we know that we must preprocess our training data before training a model, the representation as a directed graph prevents the training task from being executed before the preprocessing step is completed.
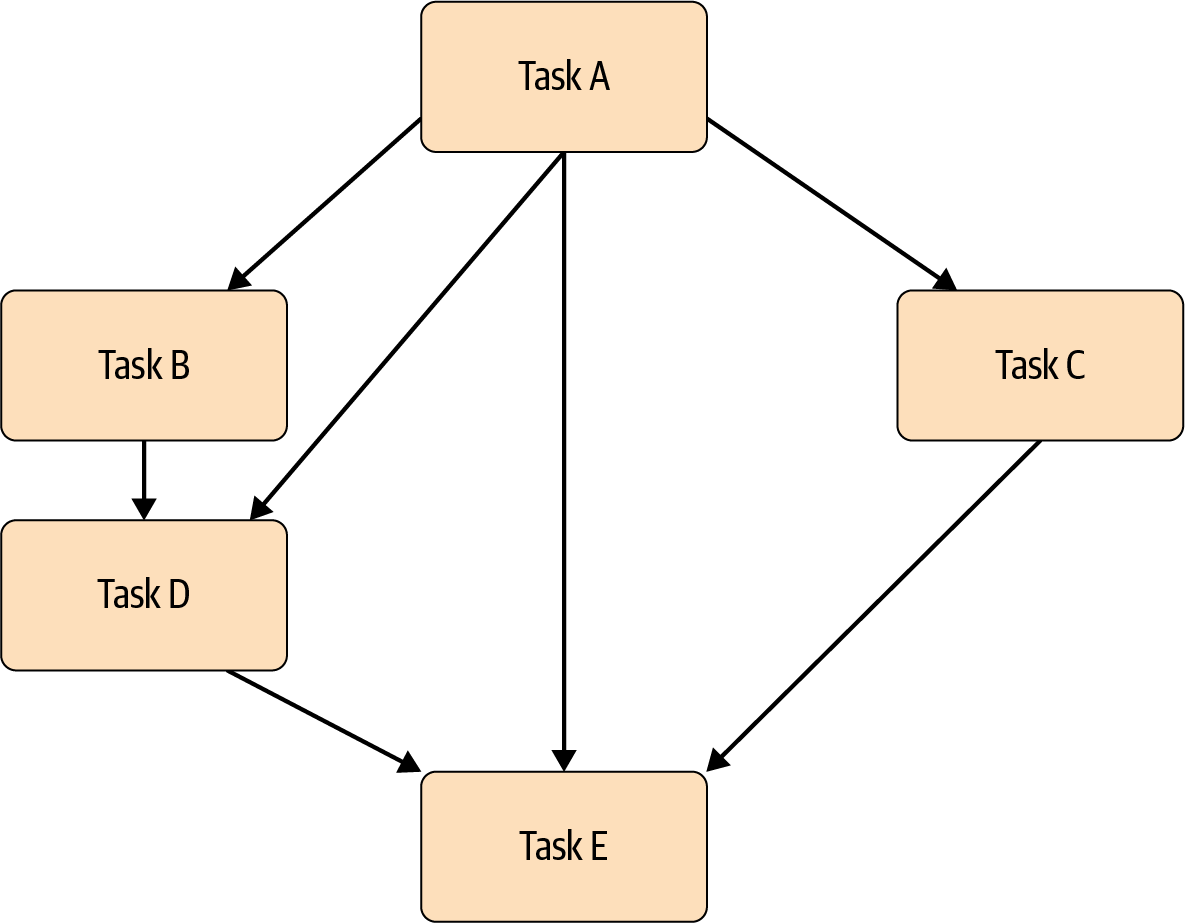
Figure 1-2. Example directed acyclic graph
Pipeline graphs must also be acyclic, meaning that a graph isn’t linking to a previously completed task. This would mean that the pipeline could run endlessly and therefore wouldn’t finish the workflow.
Because of the two conditions (being directed and acyclic), pipeline graphs are called directed acyclic graphs (DAGs). You will discover DAGs are a central concept behind most workflow tools. We will discuss more details about how these graphs are executed in Chapters 11 and 12.
Our Example Project
To follow along with this book, we have created an example project using open source data. The dataset is a collection of consumer complaints about financial products in the United States, and it contains a mixture of structured data (categorical/numeric data) and unstructured data (text). The data is taken from the Consumer Finance Protection Bureau.
Figure 1-3 shows a sample from this dataset.
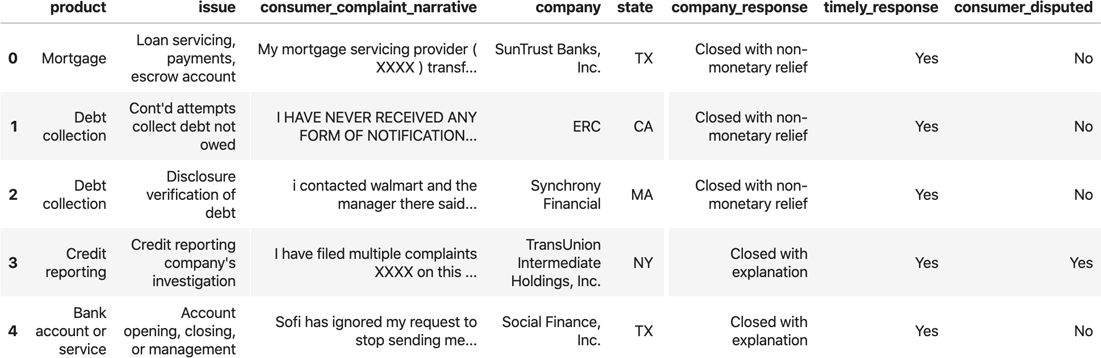
Figure 1-3. Data sample
The machine learning problem is, given data about the complaint, to predict whether the complaint was disputed by the consumer. In this dataset, 30% of complaints are disputed, so the dataset is not balanced.
Project Structure
We have provided our example project as a GitHub repo, and you can clone it as normal using the following command:
$
git clone https://github.com/Building-ML-Pipelines/\
building-machine-learning-pipelines.git
Python Package Versions
To build our example project, we used Python 3.6–3.8. We used TensorFlow version 2.2.0 and TFX version 0.22.0. We will do our best to update our GitHub repo with future versions, but we cannot guarantee that the project will work with other language or package versions.
Our example project contains the following:
-
A chapters folder containing notebooks for standalone examples from Chapters 3, 4, 7, and 14
-
A components folder with the code for common components such as the model definition
-
A complete interactive pipeline
-
An example of a machine learning experiment, which is the starting point for the pipeline
-
Complete example pipelines orchestrated by Apache Beam, Apache Airflow, and Kubeflow Pipelines
-
A utility folder with a script to download the data
In the following chapters. we will guide you through the necessary steps to turn the example machine learning experiment, in our case a Jupyter Notebook with a Keras model architecture, into a complete end-to-end machine learning pipeline.
Our Machine Learning Model
The core of our example deep learning project is the model generated by the function get_model
in the components/module.py
script of our example project. The model predicts whether a consumer disputed a complaint using the following features:
-
The financial product
-
The subproduct
-
The company’s response to the complaint
-
The issue that the consumer complained about
-
The US state
-
The zip code
-
The text of the complaint (the narrative)
For the purpose of building the machine learning pipeline, we assume that the model architecture design is done and we won’t modify the model. We discuss the model architecture in more detail in Chapter 6. But for this book, the model architecture is a very minor point. This book is all about what you can do with your model once you have it.
Goal of the Example Project
Over the course of this book, we will demonstrate the necessary frameworks, components, and infrastructure elements to continuously train our example machine learning model. We will use the stack in the architecture diagram shown in Figure 1-4.
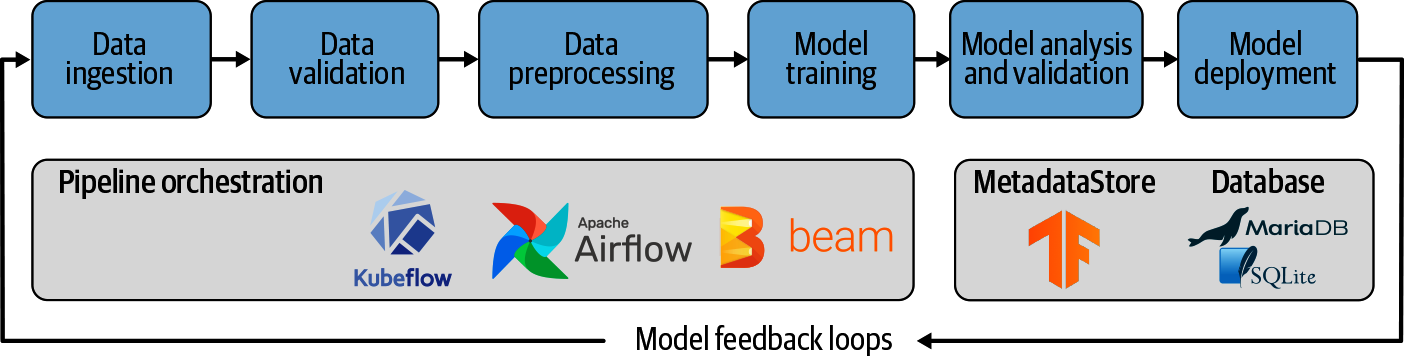
Figure 1-4. Machine learning pipeline architecture for our example project
We have tried to implement a generic machine learning problem that can easily be replaced with your specific machine learning problem. The structure and the basic setup of the machine learning pipeline remains the same and can be transferred to your use case. Each component will require some customization (e.g., where to ingest the data from), but as we will discuss, the customization needs will be limited.
Summary
In this chapter, we have introduced the concept of machine learning pipelines and explained the individual steps. We have also shown the benefits of automating this process. In addition, we have set the stage for the following chapters and included a brief outline of every chapter along with an introduction of our example project. In the next chapter, we will start building our pipeline!
1 In supervised classification problems with multiple classes as outputs, it’s often necessary to convert from a category to a vector such as (0,1,0), which is a one-hot vector, or from a list of categories to a vector such as (1,1,0), which is a multi-hot vector.
2 Google started an internal project called Sibyl in 2007 to manage an internal machine learning production pipeline. However, in 2015, the topic gained wider attention when D. Sculley et al. published their learnings of machine learning pipelines, “Hidden Technical Debt in Machine Learning Systems”.
3 D. Sculley et al., “Hidden Technical Debt in Machine Learning Systems,” Google, Inc. (2015).
Get Building Machine Learning Pipelines now with the O’Reilly learning platform.
O’Reilly members experience books, live events, courses curated by job role, and more from O’Reilly and nearly 200 top publishers.